Entropy-based Optimization on Individual and Global Predictions for Semi-Supervised Learning
MM '23: Proceedings of the 31st ACM International Conference on Multimedia(2023)
摘要
Pseudo-labelling-based semi-supervised learning (SSL) has demonstrated remarkable success in enhancing model performance by effectively leveraging a large amount of unlabeled data. However, existing studies focus mainly on rectifying individual predictions (i.e., pseudo-labels) on each unlabeled instance but ignore the overall prediction statistics from a global perspective. Such neglect may lead to model collapse and performance degradation in SSL, especially in label-scarce scenarios. In this paper, we emphasize the cruciality of global prediction constraints and propose a new SSL method that employs Entropy-based optimization on both Individual and Global predictions of unlabeled instances, dubbed EntInG. Specifically, we propose two criteria for leveraging unlabeled data in SSL: individual prediction entropy minimization (IPEM) and global distribution entropy maximization (GDEM). On the one hand, we show that current dominant SSL methods can be viewed as an implicit form of IPEM improved by recent augmentation techniques. On the other hand, we construct a new distribution loss to encourage GDEM, which greatly benefits producing better pseudo-labels for unlabeled data. Theoretical analysis also demonstrates that our proposed criteria can be derived by enforcing mutual information maximization on unlabeled instances. Despite its simplicity, our proposed method can achieve significant accuracy gains on popular SSL classification benchmarks.
更多查看译文
AI 理解论文
溯源树
样例
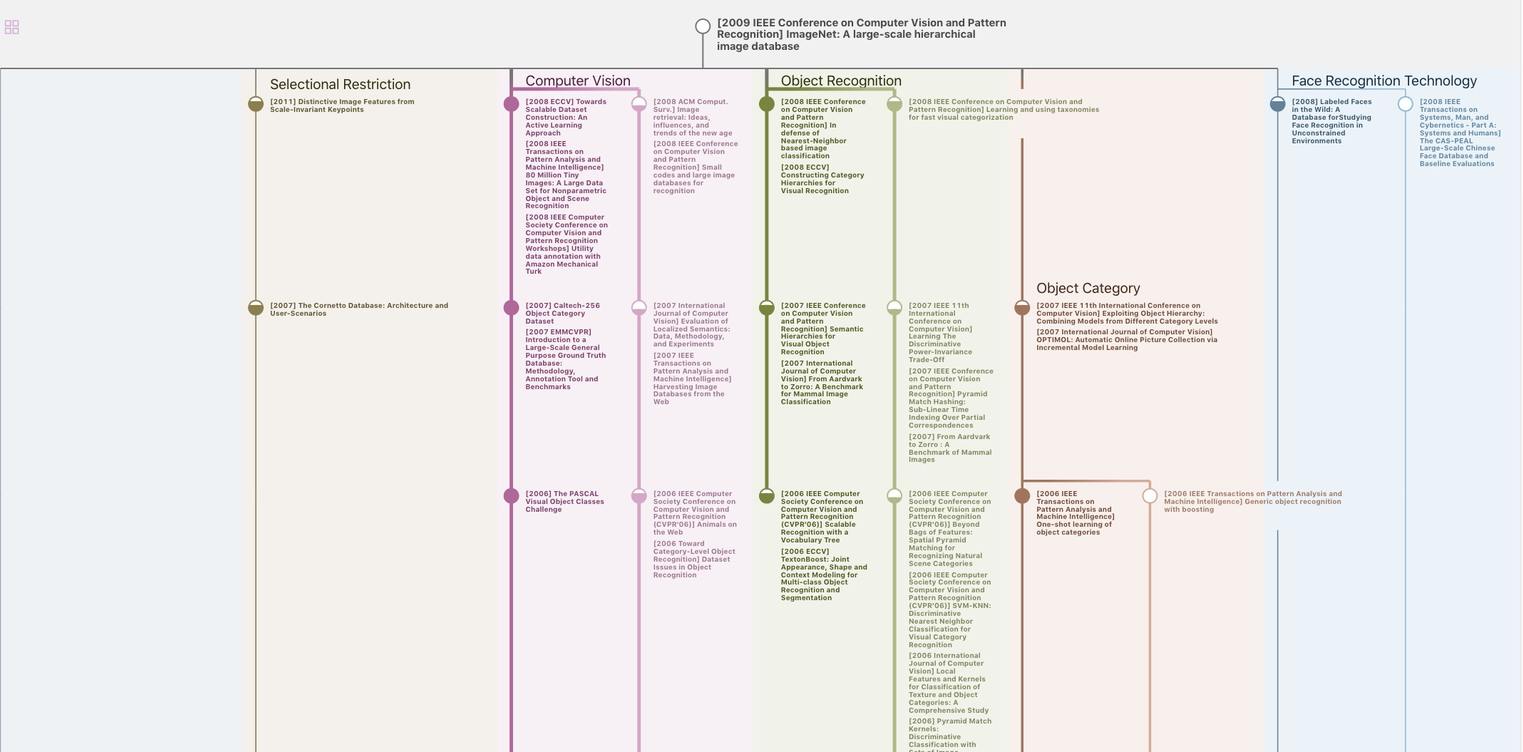
生成溯源树,研究论文发展脉络
Chat Paper
正在生成论文摘要