Knowledge Decomposition and Replay: A Novel Cross-modal Image-Text Retrieval Continual Learning Method
MM '23: Proceedings of the 31st ACM International Conference on Multimedia(2023)
摘要
To enable machines to mimic human cognitive abilities and alleviate the catastrophic forgetting problem in cross-modal image-text retrieval (CMITR), this paper proposes a novel continual learning method, Knowledge Decomposition and Replay (KDR), which emulates the process of knowledge decomposition and replay exhibited by humans in complex and changing environments. KDR has two components: a feature Decomposition-based CMITR Model (DCM) and a cross-task Generic Knowledge Replay strategy (GKR). DCM decomposes text and image features into task-specific and generic knowledge features, mimicking the human cognitive process of knowledge decomposition. Specifically, it employs a generic knowledge features extraction module for all tasks and a task-specific module for each task with a few trainable fully connected layers. Similarly, GKR emulates the human behavior of knowledge replay by utilizing the image-text similarity matrix output from the old task model with inputting the previous samples to induce the learning of the image-text similarity matrix output from the current task model with inputting the previous samples, using knowledge distillation technology. To demonstrate the effect of KDR, we adapted a continual learning dataset Seq-COCO from MSCOCO. Extensive experiments on Seq-COCO showed that KDR reduces catastrophic forgetting and consolidates general knowledge, improving the model's learning ability in CMITR.
更多查看译文
AI 理解论文
溯源树
样例
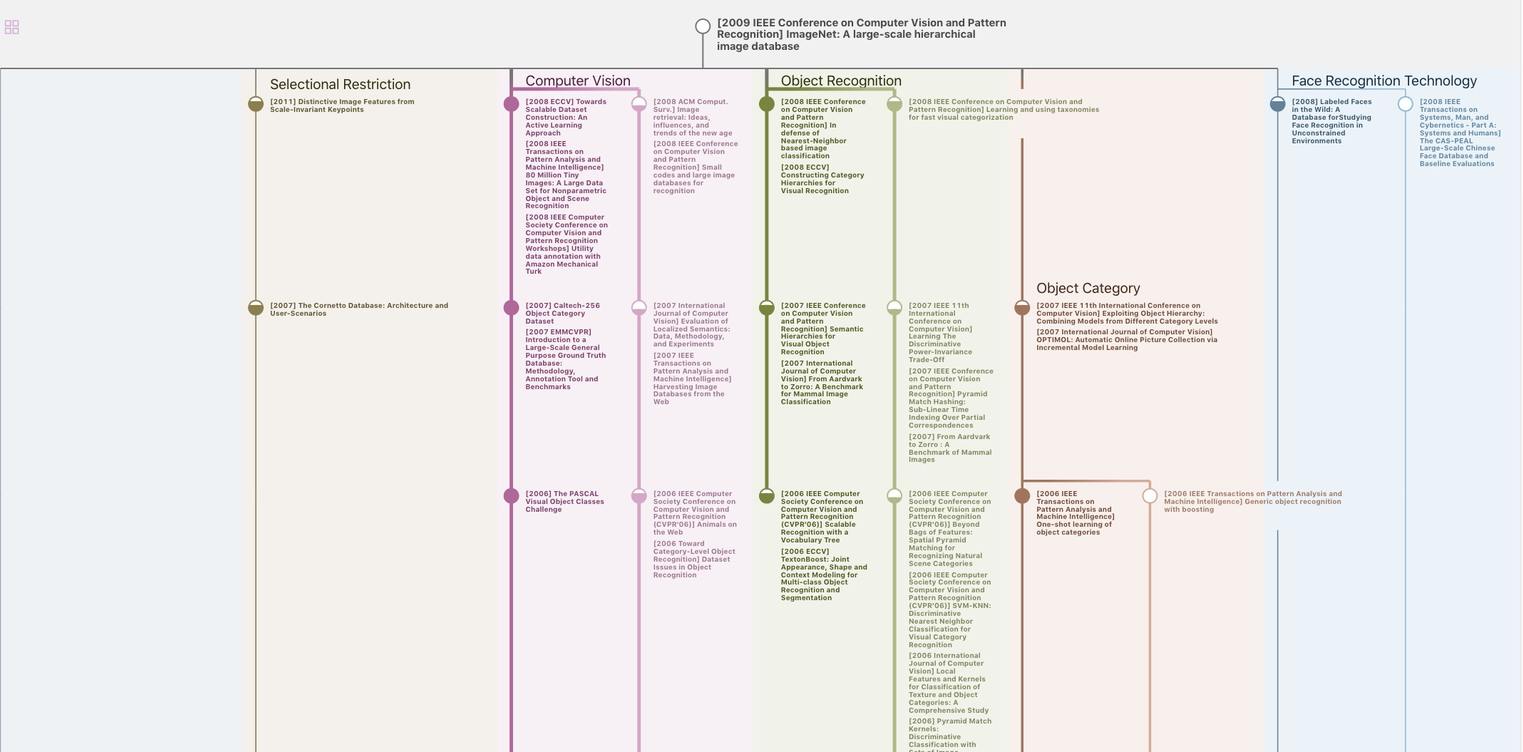
生成溯源树,研究论文发展脉络
Chat Paper
正在生成论文摘要