IS2Net: Intra-domain Semantic and Inter-domain Style Enhancement for Semi-supervised Medical Domain Generalization
MM '23: Proceedings of the 31st ACM International Conference on Multimedia(2023)
摘要
Domain generalization (DG) demonstrates superior generalization ability in cross-center medical image segmentation. Despite its great success, existing fully supervised DG methods require collecting a large quantity of pixel-level annotations which is quite expensive and time-consuming. To address this challenge, several semi-supervised domain generalized (SSDG) methods have been proposed by simply coupling semi-supervised learning (SSL) with DG tasks, which give rise to two main concerns: (1) Intra-domain dubious semantic information: the quality of pseudo labels in each source domain suffers from the limited amount of labeled data and cross-domain discrepancy. (2) Inter-domain intangible style relationship: current models fail in integrating domain-level information and overlook the relationships among different domains, which degrades the generalization ability of model. In light of these two issues, we propose a novel SSDG framework, namely IS2Net, by arranging an inter-domain generalization branch and several intra-domain SSL branches in a parallel manner, powered by two appealing designs that build a positive interaction between them: (1) A style and semantic memory mechanism is designed to provide both high-quality class-wise representations for intra-domain semantic enhancement and stable domain-specific knowledge for inter-domain style relationship construction. (2) Confident pseudo labeling strategy aims at generating more reliable supervision for intra and inter domain branches, and thus facilitating the learning process of the whole framework. Extensive experiments show that IS2Net yields consistent improvements over the state-of- the-art methods in three public benchmarks.
更多查看译文
AI 理解论文
溯源树
样例
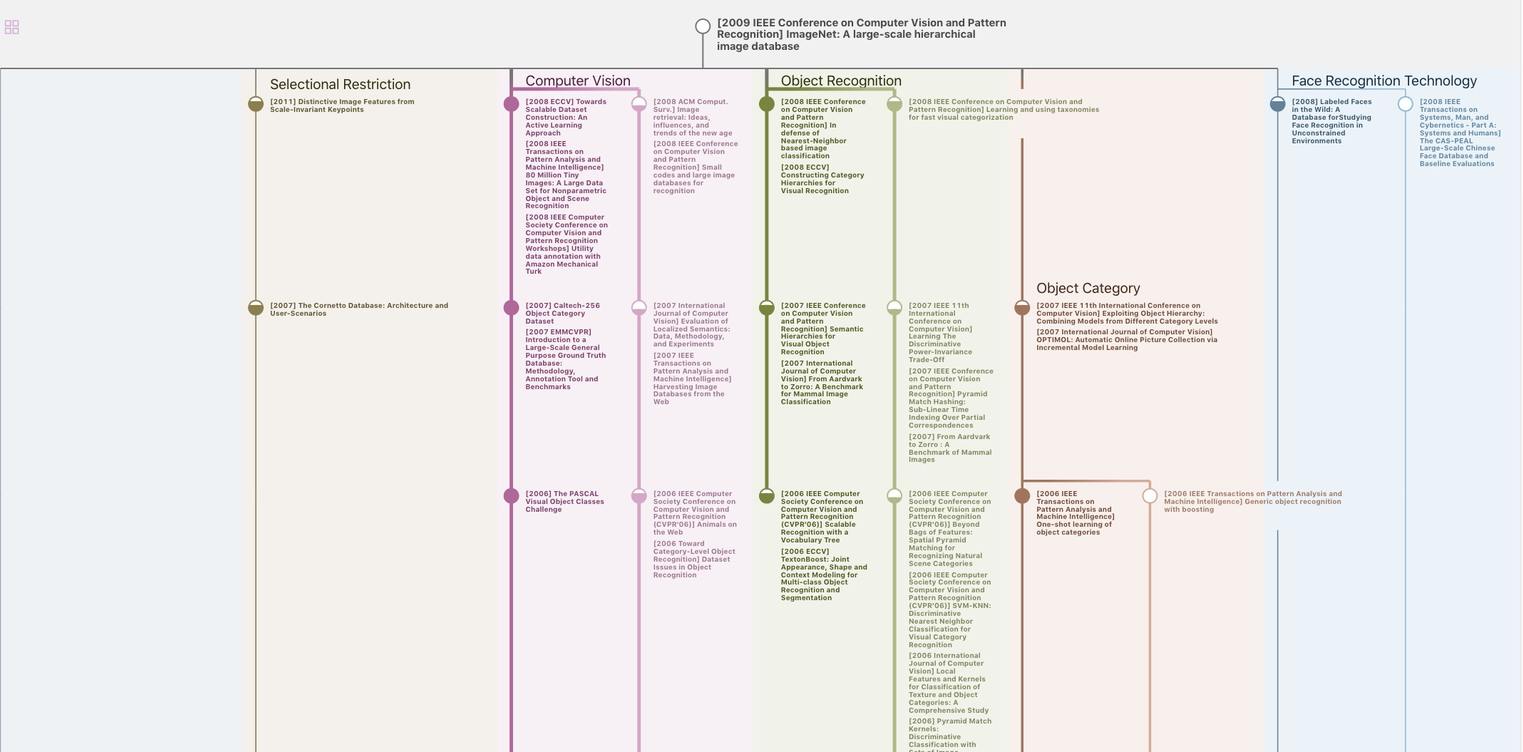
生成溯源树,研究论文发展脉络
Chat Paper
正在生成论文摘要