Slowfast Diversity-aware Prototype Learning for Egocentric Action Recognition
MM '23: Proceedings of the 31st ACM International Conference on Multimedia(2023)
摘要
Egocentric Action Recognition (EAR) is required to recognize both the interacting objects (noun) and the motion (verb) against cluttered backgrounds with distracting objects. For capturing interacting objects, traditional approaches heavily rely on luxury object annotations or detectors, though a few works heuristically enumerate the fixed sets of verb-constrained prototypes to roughly exclude the background. For capturing motion, the inherent variations of motion duration among egocentric videos with different lengths are almost ignored. To this end, we propose a novel Slowfast Diversity-aware Prototype learning (SDP) to effectively capture interacting objects by learning compact yet diverse prototypes, and adaptively capture motion in either long-time video or short-time video. Specifically, we present a new Part-to-Prototype (P2P) scheme to learn prototypes from raw videos covering the interacting objects by refining the semantic information from part level to prototype level. Moreover, for adaptively capturing motion, we design a new Slow-Fast Context (SFC) mechanism that explores the Up/Down augmentations for the prototype representation at the semantic level to strengthen the transient dynamic information in short-time videos and eliminate the redundant dynamic information in long-time videos, which are further fine-complemented via the slow- and fast-aware attentions. Extensive experiments demonstrate SDP outperforms state-of-the-art methods on two large-scale egocentric video benchmarks, i.e., EPIC-KITCHENS-100 and EGTEA.
更多查看译文
AI 理解论文
溯源树
样例
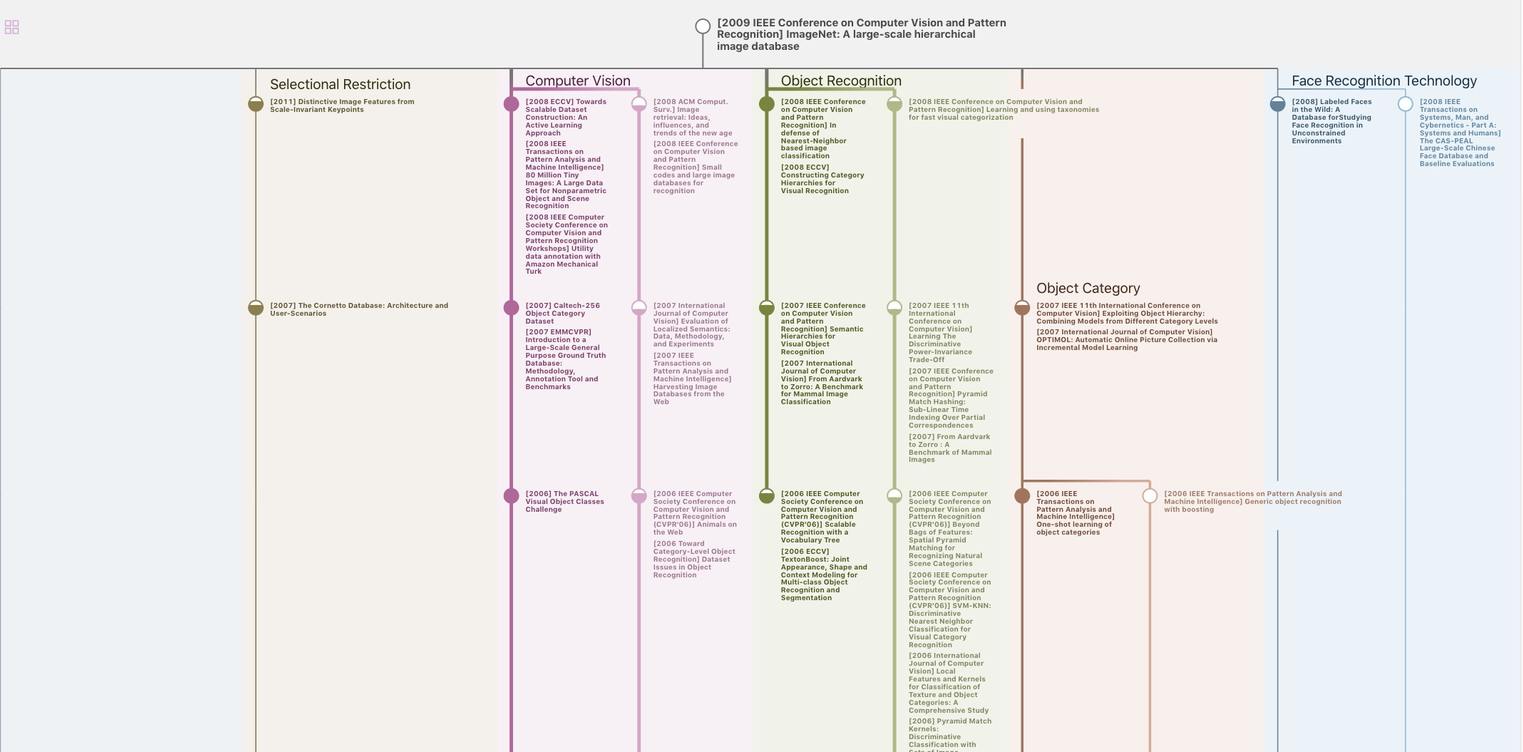
生成溯源树,研究论文发展脉络
Chat Paper
正在生成论文摘要