Deep Video Understanding with Video-Language Model
MM '23: Proceedings of the 31st ACM International Conference on Multimedia(2023)
摘要
Pre-trained video-language models (VLMs) have shown superior performance in high-level video understanding tasks, analyzing multi-modal information, aligning with Deep Video Understanding Challenge (DVUC) requirements.In this paper, we explore pre-trained VLMs' potential in multimodal question answering for long-form videos. We propose a solution called Dual Branches Video Modeling (DBVM), which combines knowledge graph (KG) and VLMs, leveraging their strengths and addressing shortcomings.The KG branch recognizes and localizes entities, fuses multimodal features at different levels, and constructs KGs with entities as nodes and relationships as edges.The VLM branch applies a selection strategy to adapt input movies into acceptable length and a cross-matching strategy to post-process results providing accurate scene descriptions.Experiments conducted on the DVUC dataset validate the effectiveness of our DBVM.
更多查看译文
AI 理解论文
溯源树
样例
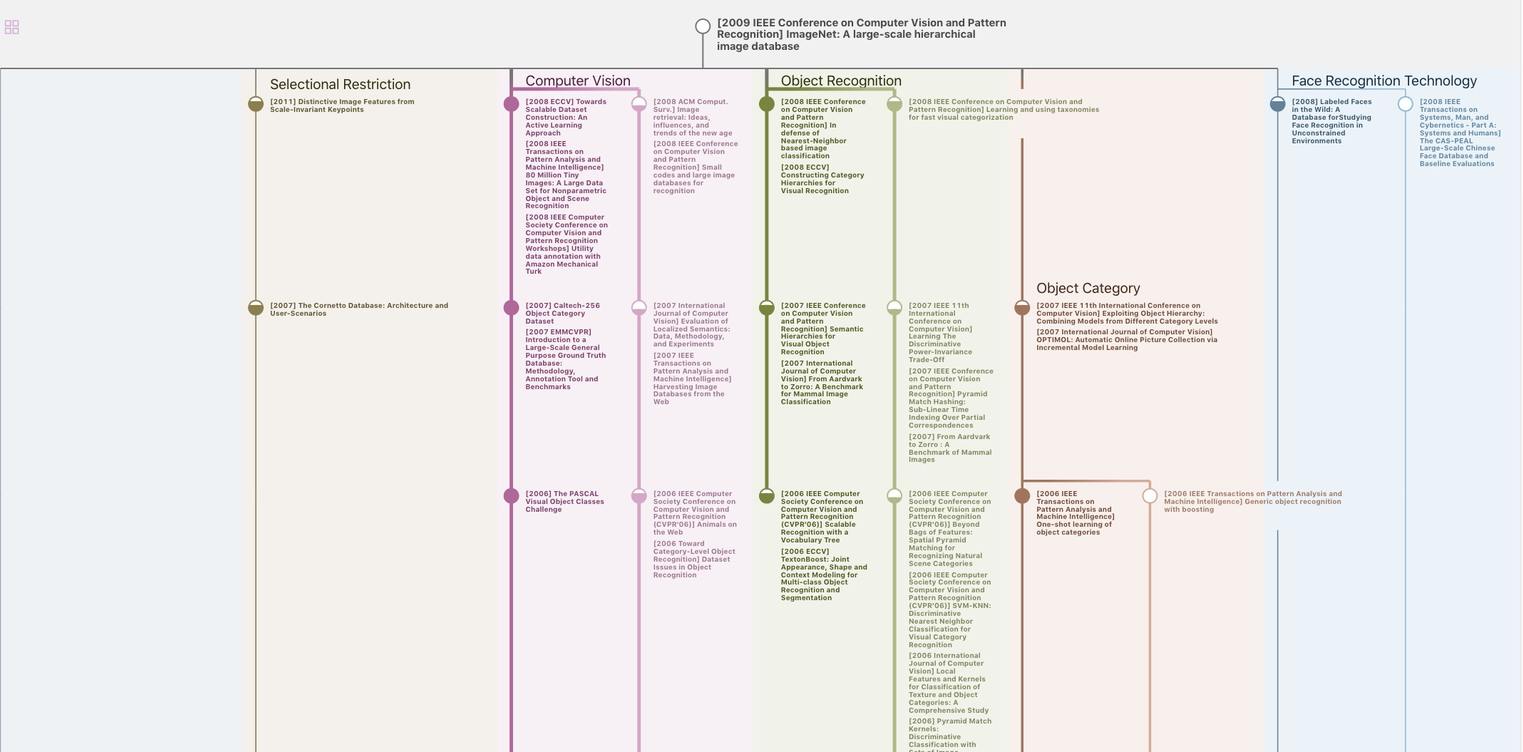
生成溯源树,研究论文发展脉络
Chat Paper
正在生成论文摘要