Lite-MKD: A Multi-modal Knowledge Distillation Framework for Lightweight Few-shot Action Recognition
MM '23: Proceedings of the 31st ACM International Conference on Multimedia(2023)
摘要
Existing few-shot action recognition methods have placed primary focus on improving the recognition accuracy while neglecting another important indicator in practical scenarios, i.e., model efficiency. In this paper, we make the first attempt and propose a Lightweight Multi-modal Knowledge Distillation framework (Lite-MKD) for few-shot action recognition. In this framework, the teacher model conducts multi-modal learning to achieve a comprehensive fusion of the optical flow, depth, and appearance features of human movements, thus achieving a more robust representation of actions. The student model is utilized to learn to recognize actions from the single RGB modality at a lower computational cost under the guidance of the teacher. To fully explore and integrate multi-modal information, a hierarchical Multi-modal Fusion Module (MFM) is introduced in the teacher model. Besides, a multi-level Distinguish-to-Mimic (D2M) knowledge distillation component is proposed for the student model. D2M improves the ability of the student model to mimic the action classification probabilities of the teacher model by enhancing the distinguishability of the student model for different video categories in the support set. Extensive experiments on three action recognition datasets Kinetics, HMDB51, and UCF101 demonstrate our framework's effectiveness and stable generalization ability. With a much more lightweight network for inference, we achieve comparable performance to previous state-of-the-art methods. Our source code is available at https://github.com/HuiGuanLab/Lite-MKD
更多查看译文
AI 理解论文
溯源树
样例
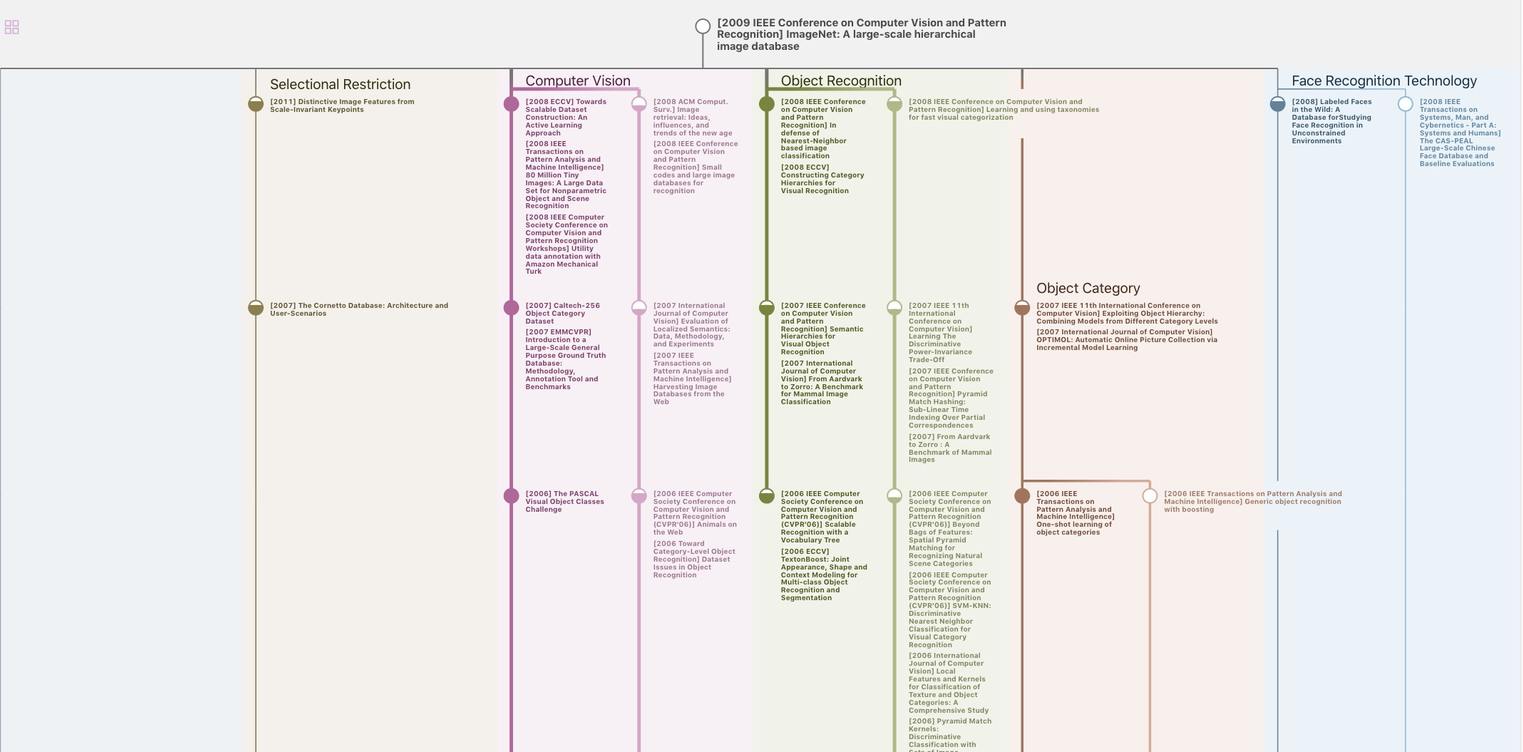
生成溯源树,研究论文发展脉络
Chat Paper
正在生成论文摘要