Expected Goals Prediction in Professional Handball using Synchronized Event and Positional Data
PROCEEDINGS OF THE 6TH INTERNATIONAL WORKSHOP ON MULTIMEDIA CONTENT ANALYSIS IN SPORTS, MMSPORTS 2023(2023)
摘要
In this study, we employ an extensive single-season dataset of event and positional data, as well as machine learning techniques, to build an Expected Goals (xG) model for handball. The selected features of the data include distances, angles, and game context. Several algorithms were considered, and after a five-fold cross-validation and hyperparameter optimization, CatBoost emerged as the most suitable, achieving a predictive accuracy of about 70%. Our methodology integrates a data synchronization and throw detection approach, along with a comprehensive exploration of feature importance using the SHapley Additive exPlanations (SHAP) method. The findings provide insights into handball strategy and player performance, unlocking new potential for game analysis and tactical planning. Looking forward, the practical applications of this research extend to enhancing player training, refining team strategies, and offering a deeper understanding of handball dynamics.
更多查看译文
关键词
Handball,Expected Goals,Machine Learning,Predictive Modeling
AI 理解论文
溯源树
样例
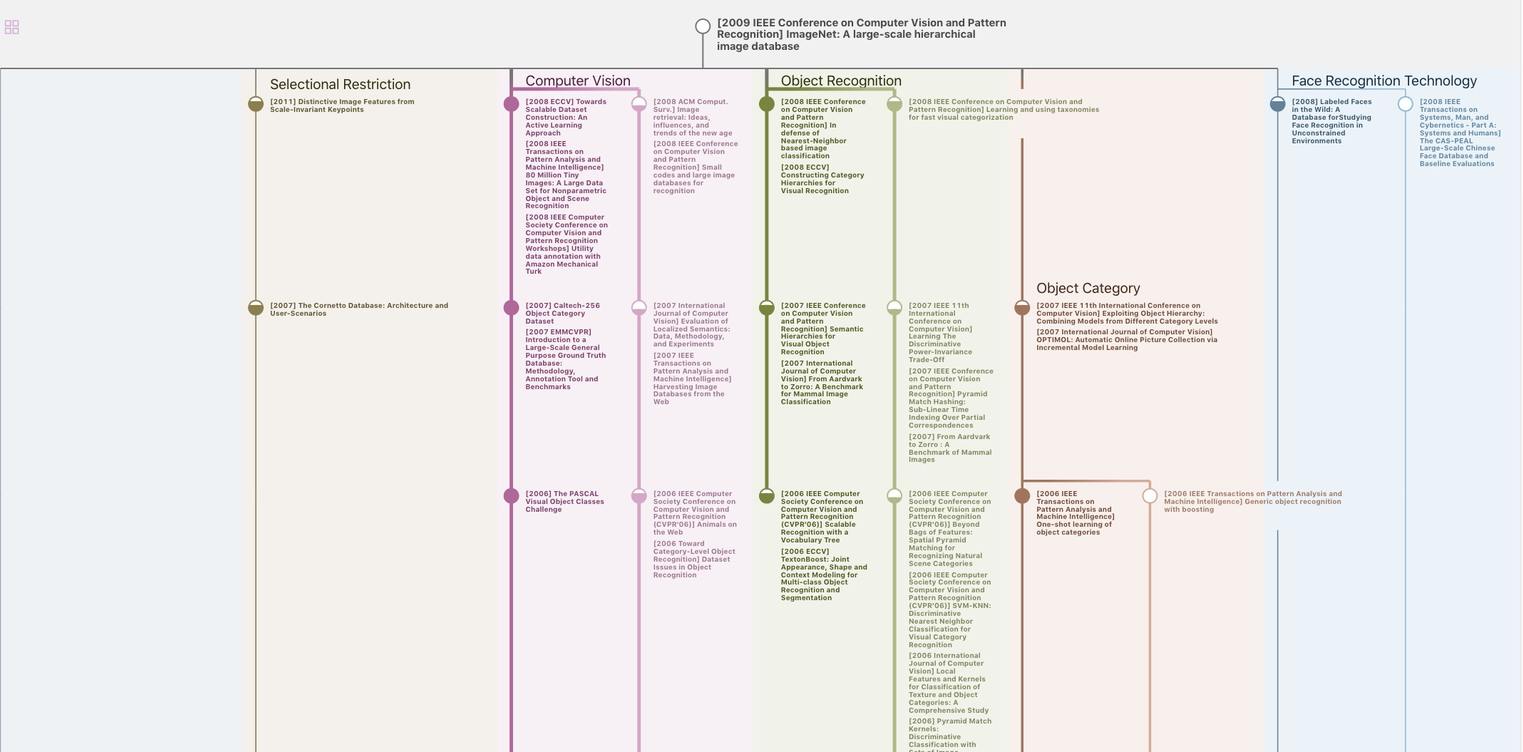
生成溯源树,研究论文发展脉络
Chat Paper
正在生成论文摘要