ACQ: Few-shot Backdoor Defense via Activation Clipping and Quantizing
MM '23: Proceedings of the 31st ACM International Conference on Multimedia(2023)
摘要
In recent years, deep neural networks(DNNs) have relied on an increasing amount of training samples as the premise of the deployment for real-world scenarios. This gives rise to backdoor attacks, where a small fraction of poisoned data is inserted into the training dataset to manipulate the predictions of DNNs when presented with backdoor inputs. Backdoor attacks pose serious security threats during the prediction stage of DNNs. As a result, there is growing research attention to defend against backdoor attacks. This paper proposes Activation Clipping and Quantizing (ACQ), a novel backdoor elimination module via transforming the intermediate-layer output of DNNs during forward propagation by embedding Clipper and Quantizer into the backdoored DNNs. ACQ is motivated by the observation that the backdoored DNNs always output abnormally large or small intermediate-layer activations when presented with backdoored samples, eventually leading to the malicious prediction of backdoored DNNs. ACQ modifies backdoored DNNs to keep the intermediate-layer activations in a proper domain and align the forward propagation of backdoored samples with that of clean samples. Besides, we highlight that ACQ has the ability to eliminate the backdoor of DNNs in few-shot even zero-shot scenarios, which requires much fewer or even no clean samples for the backdoor elimination stage than existing approaches. Experiments demonstrate the effectiveness and robustness of ACQ against various attacks and tasks compared to existing methods. Our code and Appendix can be found in https://github.com/Backdoor-defense/ACQ
更多查看译文
AI 理解论文
溯源树
样例
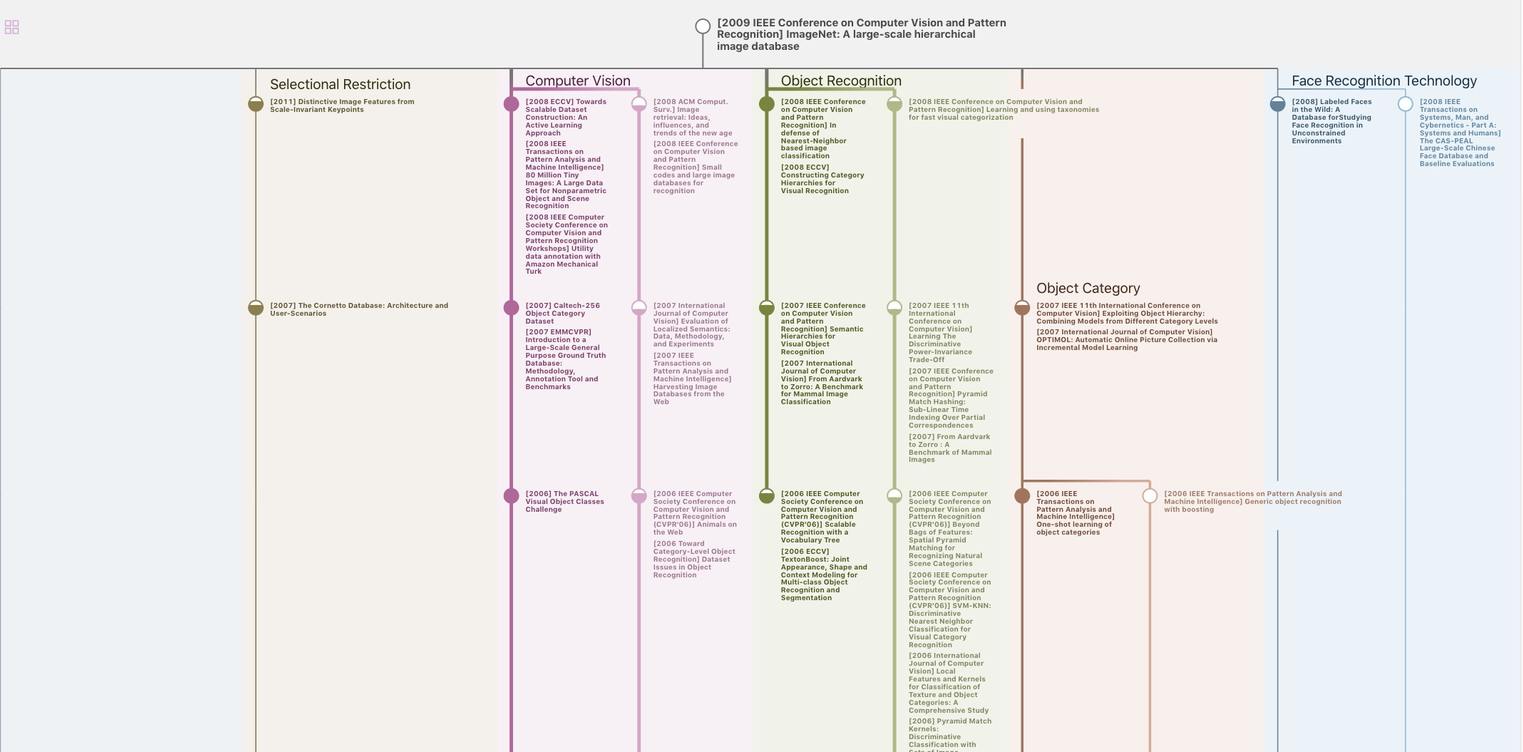
生成溯源树,研究论文发展脉络
Chat Paper
正在生成论文摘要