Exploring Coarse-to-Fine Action Token Localization and Interaction for Fine-grained Video Action Recognition
MM '23: Proceedings of the 31st ACM International Conference on Multimedia(2023)
摘要
Vision transformers have achieved impressive performance for video action recognition due to their strong capability of modeling long-range dependencies among spatio-temporal tokens. However, as for fine-grained actions, subtle and discriminative differences mainly exist in the regions of actors, directly utilizing vision transformers without removing irrelevant tokens will compromise recognition performance and lead to high computational costs. In this paper, we propose a coarse-to-fine action token localization and interaction network, namely C2F-ALIN, that dynamically localizes the most informative tokens at a coarse granularity and then partitions these located tokens to a fine granularity for sufficient fine-grained spatio-temporal interaction. Specifically, in the coarse stage, we devise a discriminative token localization module to accurately identify informative tokens and to discard irrelevant tokens, where each localized token corresponds to a large spatial region, thus effectively preserving the continuity of action regions.In the fine stage, we only further partition the localized tokens obtained in the coarse stage into a finer granularity and then characterize fine-grained token interactions in two aspects: (1) first using vanilla transformers to learn compact dependencies among all discriminative tokens; and (2) proposing a global contextual interaction module which enables each fine-grained tokens to communicate with all the spatio-temporal tokens and to embed the global context. As a result, our coarse-to-fine strategy is able to identify more relevant tokens and integrate global context for high recognition accuracy while maintaining high efficiency.Comprehensive experimental results on four widely used action recognition benchmarks, including FineGym, Diving48, Kinetics and Something-Something, clearly demonstrate the advantages of our proposed method in comparison with other state-of-the-art ones.
更多查看译文
AI 理解论文
溯源树
样例
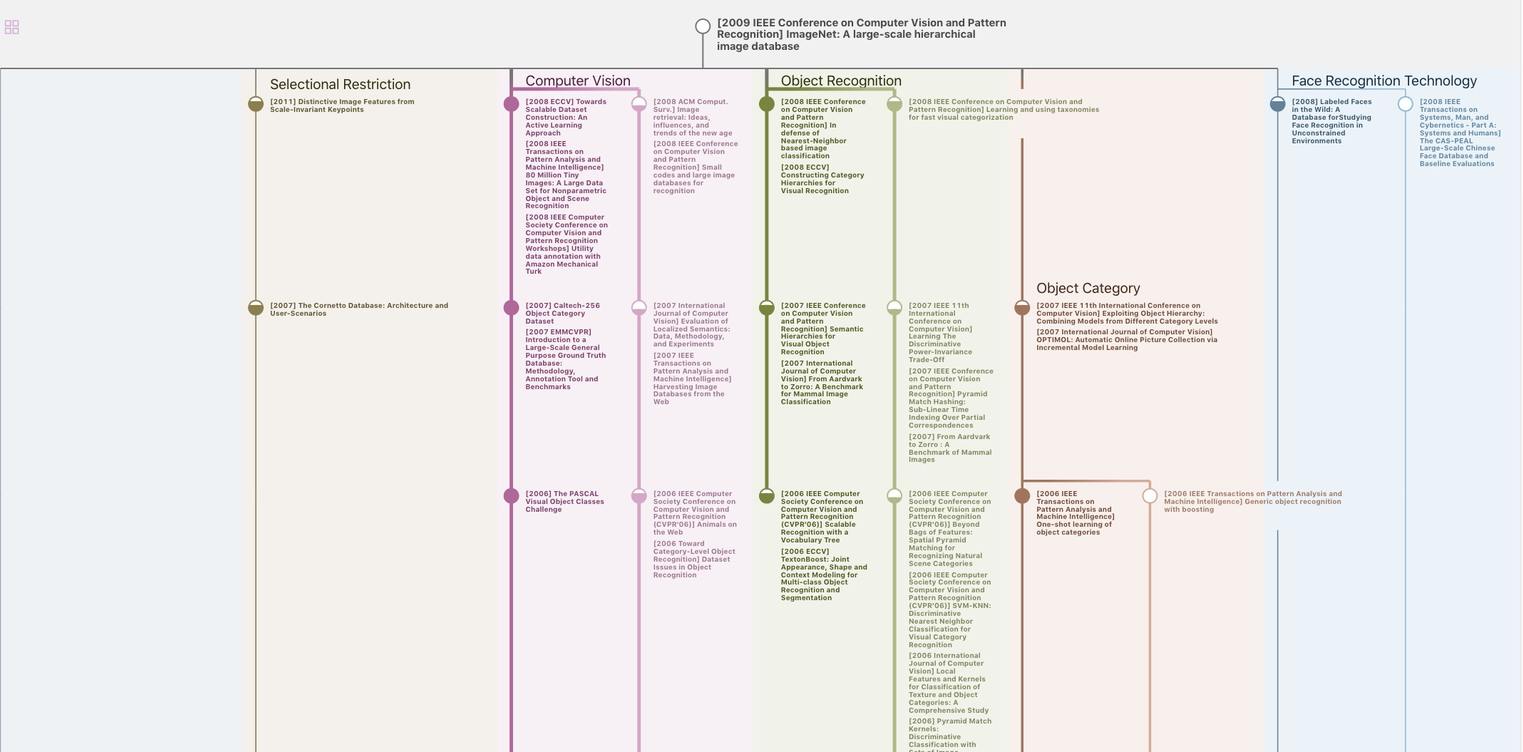
生成溯源树,研究论文发展脉络
Chat Paper
正在生成论文摘要