Diff4Rec: Sequential Recommendation with Curriculum-scheduled Diffusion Augmentation
MM '23: Proceedings of the 31st ACM International Conference on Multimedia(2023)
摘要
Sequential recommender systems often suffer from performance drops due to the data-sparsity issue in real-world scenarios. To address this issue, we bravely take advantage of the strength in diffusion model to conduct data augmentation for sequential recommendation in this paper. However, there remain two critical challenges for this scarcely-explored topic: (i) previous diffusion models are mostly designed for image generation aiming to capture pixel patterns, which can hardly be applied in data augmentation for sequential recommendation aiming to capture the user-item relations; (ii) given a specific diffusion model capable of user-item interaction augmentation, it is non-trivial to guarantee that the diffusion-generated data can always bring benefits towards the sequential recommendation model. To tackle these challenges, we propose Diff4Rec, a curriculum-scheduled diffusion augmentation framework for sequential recommendation. Specifically, a diffusion model is pre-trained on recommendation data via corrupting and reconstructing the user-item interactions in the latent space, and the generated predictions are leveraged to produce diversified augmentations for the sparse user-item interactions. Subsequently, a curriculum scheduling strategy is designed to progressively feed the diffusion-generated samples into the sequential recommenders, with respect to two levels, i.e., interaction augmentation and objective augmentation, to jointly optimize the data and model. Extensive experiments demonstrate that our proposed Diff4Rec framework is able to effectively achieve superior performance over several strong baselines, capable of making high-quality and robust sequential recommendations. We believe the proposed Diff4Rec has the promising potential to bring paradigm shift in multimedia recommendation.
更多查看译文
AI 理解论文
溯源树
样例
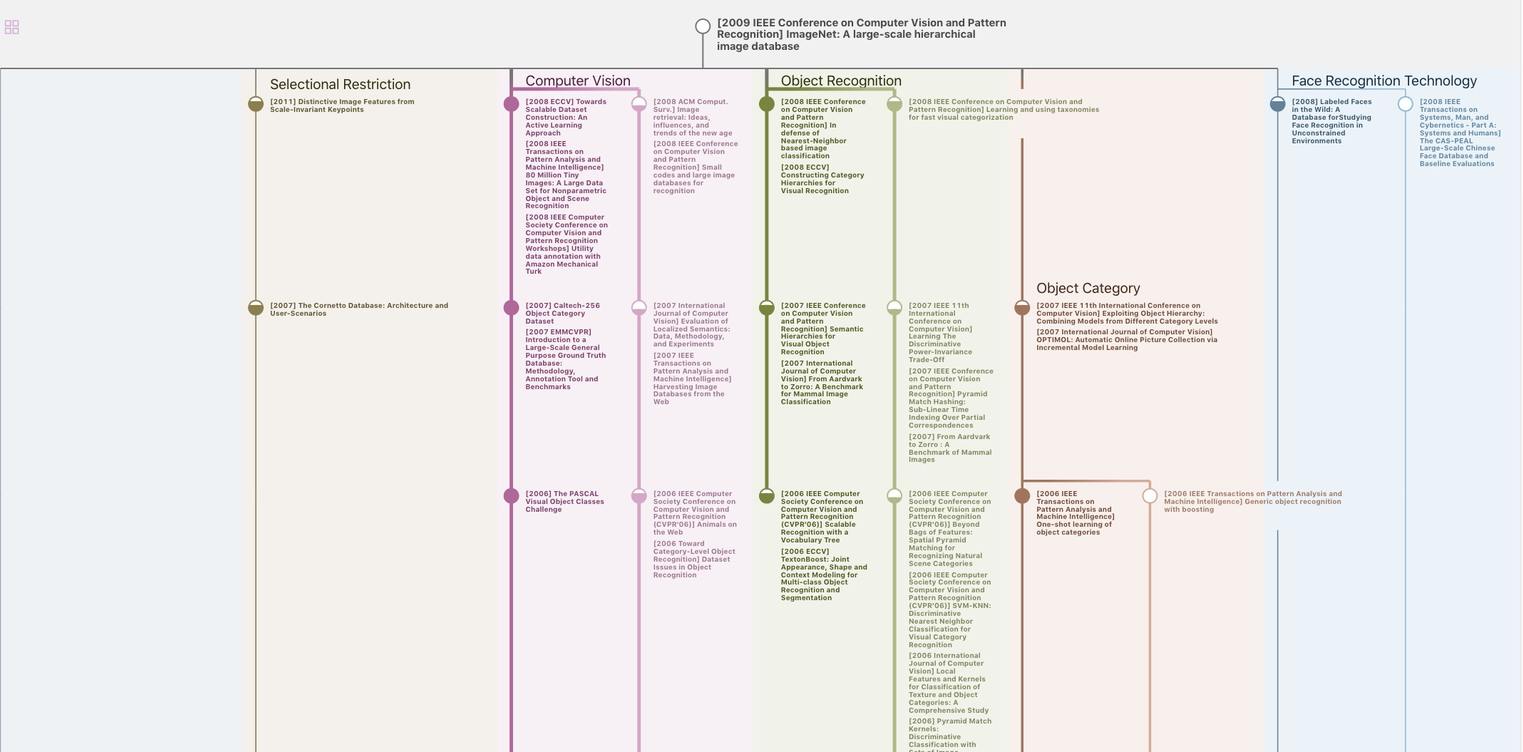
生成溯源树,研究论文发展脉络
Chat Paper
正在生成论文摘要