LiFT: Transfer Learning in Vision-Language Models for Downstream Adaptation and Generalization
MM '23: Proceedings of the 31st ACM International Conference on Multimedia(2023)
摘要
Pre-trained Vision-Language Models (VLMs) on large-scale image-text pairs, e.g., CLIP, have shown promising performance on zero-shot knowledge transfer. Recently, fine-tuning pre-trained VLMs to downstream few-shot classification with limited image annotation data yields significant gains. However, there are two limitations. First, most of the methods for fine-tuning VLMs only update newly added parameters while keeping the whole VLM frozen. Thus, it remains unclear how to directly update the VLM itself. Second, fine-tuning VLMs to a specific set of base classes would deteriorate the well-learned representation space such that the VLMs generalize poorly on novel classes. To address these issues, we first propose Layer-wise Fine-Tuning (LiFT) which achieves average gains of 3.9%, 4.3%, 4.2% and 4.5% on base classes under 2-, 4-, 8- and 16-shot respectively compared to the baseline CoOp over 11 datasets. Alternatively, we provide a parameter-efficient LiFT-Adapter exhibiting favorable performance while updating only 1.66% of total parameters. Further, we design scalable LiFT-NCD to identify both base classes and novel classes, which boosts the accuracy by an average of 5.01% over zero-shot generalization of CLIP, exploring the potential of VLMs in discovering novel classes.
更多查看译文
AI 理解论文
溯源树
样例
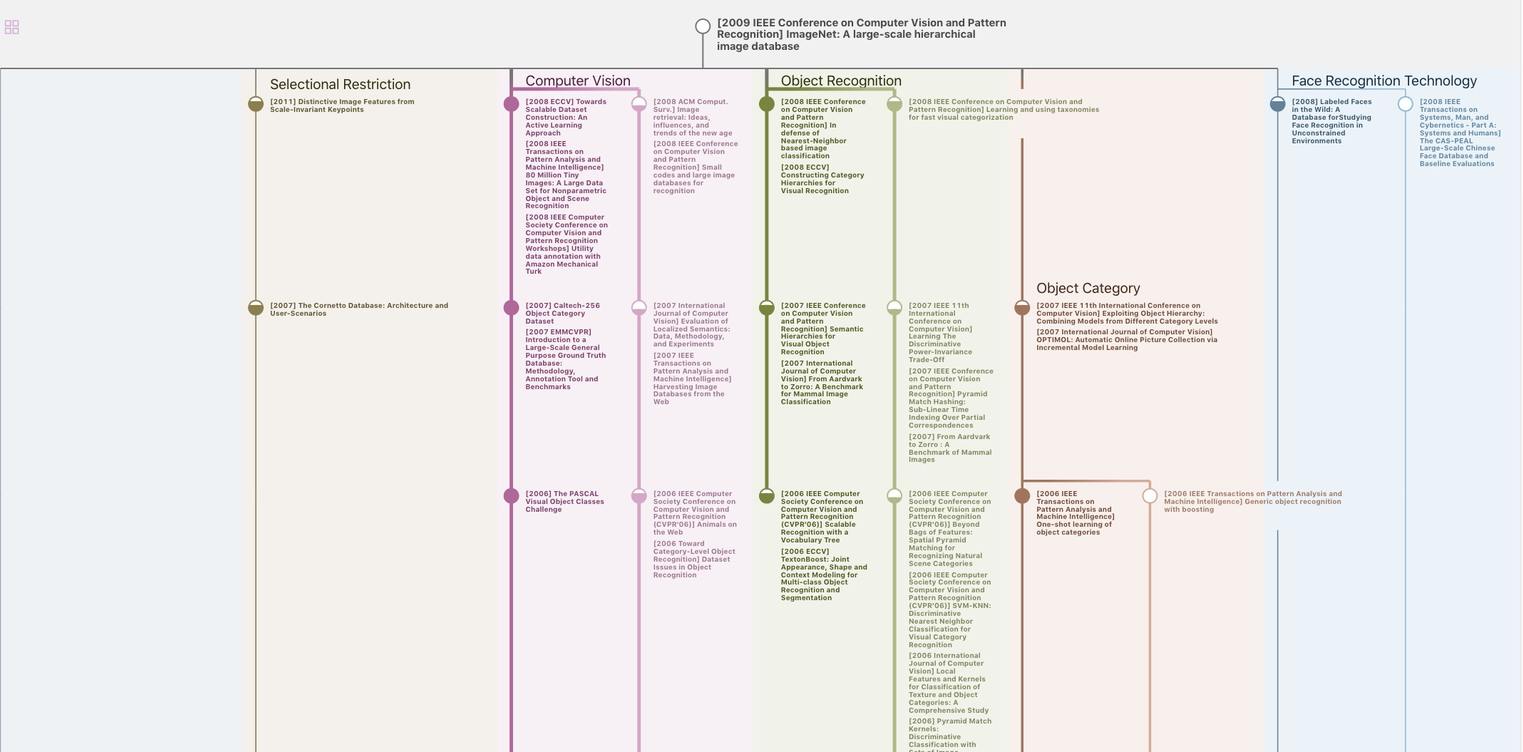
生成溯源树,研究论文发展脉络
Chat Paper
正在生成论文摘要