Towards Adaptable Graph Representation Learning: An Adaptive Multi-Graph Contrastive Transformer
MM '23: Proceedings of the 31st ACM International Conference on Multimedia(2023)
摘要
Significant progress has been made in graph representation learning in recent years. However, most of these methods model spatial relationships via predefined graphs or decouple spatial-temporal representations, which limits the generalization and effectiveness of the model. To address these issues, we introduce an adaptive multi-graph contrastive transformer (AMGCT) for general spatial-temporal graph representation learning. Specifically, we first propose adaptive multi-graph contrastive learning (AMGCL). Without any expert knowledge, AMGCL can gradually generate adaptive spatial graphs with different topologies to learn spatial representations from different views. Cross-graph contrastive learning further explores potential correlations between different views, making each view's features more discriminative. In addition, to avoid insufficient interaction caused by decoupling spatial-temporal information in existing methods, we design a coupled graph transformer (CGT) to consider spatial relationships at each stage of temporal modeling, explore complementary information between spatial and temporal domains, and obtain more compact spatial-temporal representations. Experimental results on two different spatial-temporal graph datasets and tasks demonstrate that the proposed method achieves excellent performance.
更多查看译文
AI 理解论文
溯源树
样例
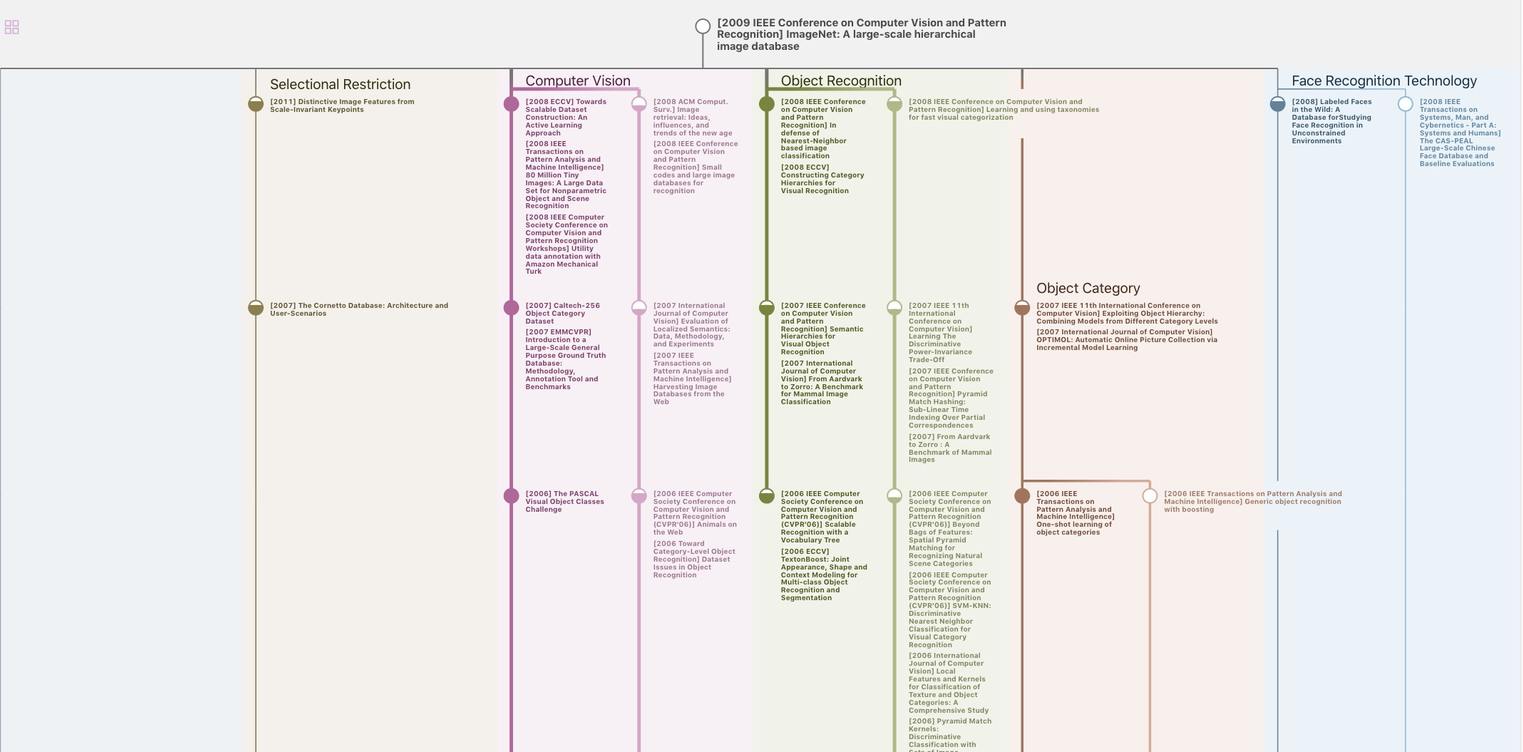
生成溯源树,研究论文发展脉络
Chat Paper
正在生成论文摘要