CoCa: A Connectivity-Aware Cascade Framework for Histology Gland Segmentation
MM '23: Proceedings of the 31st ACM International Conference on Multimedia(2023)
摘要
Gland segmentation is crucial for computer-aided diagnosis of adenocarcinoma. However, Topologically Critical Areas (TCAs), such as background tissues between two adjacent glands, can easily cause under- or over-connection of gland topological structures that may lead to the opposite diagnostic of the malignancy degree. Therefore, we provide a novel perspective for gland segmentation by incorporating gland connectivity information to locate critical errors within TCAs. We propose a Connectivity-Aware Cascade framework (CoCa) that explicitly encodes gland connectivity information into the network to locate all connectivity errors during training and then leverage attention operations to focus on these errors. Since under- or over-connected glands can change the Betti number (e.g., number of connected components) of glands, we design a Connectivity Refinement Module (CRM) to compare the Betti number of each gland to locate connectivity errors. We propose CoCa-Net to mine the topological relations among different biomedical entities to guide gland prediction. We also use contrastive learning to separate pixel embeddings of different classes within TCAs through our connectivity-aware hard example sampling strategy. Extensive experiments on the GlaS and CRAG datasets demonstrate the effectiveness of CoCa over state-of-the-art methods.
更多查看译文
AI 理解论文
溯源树
样例
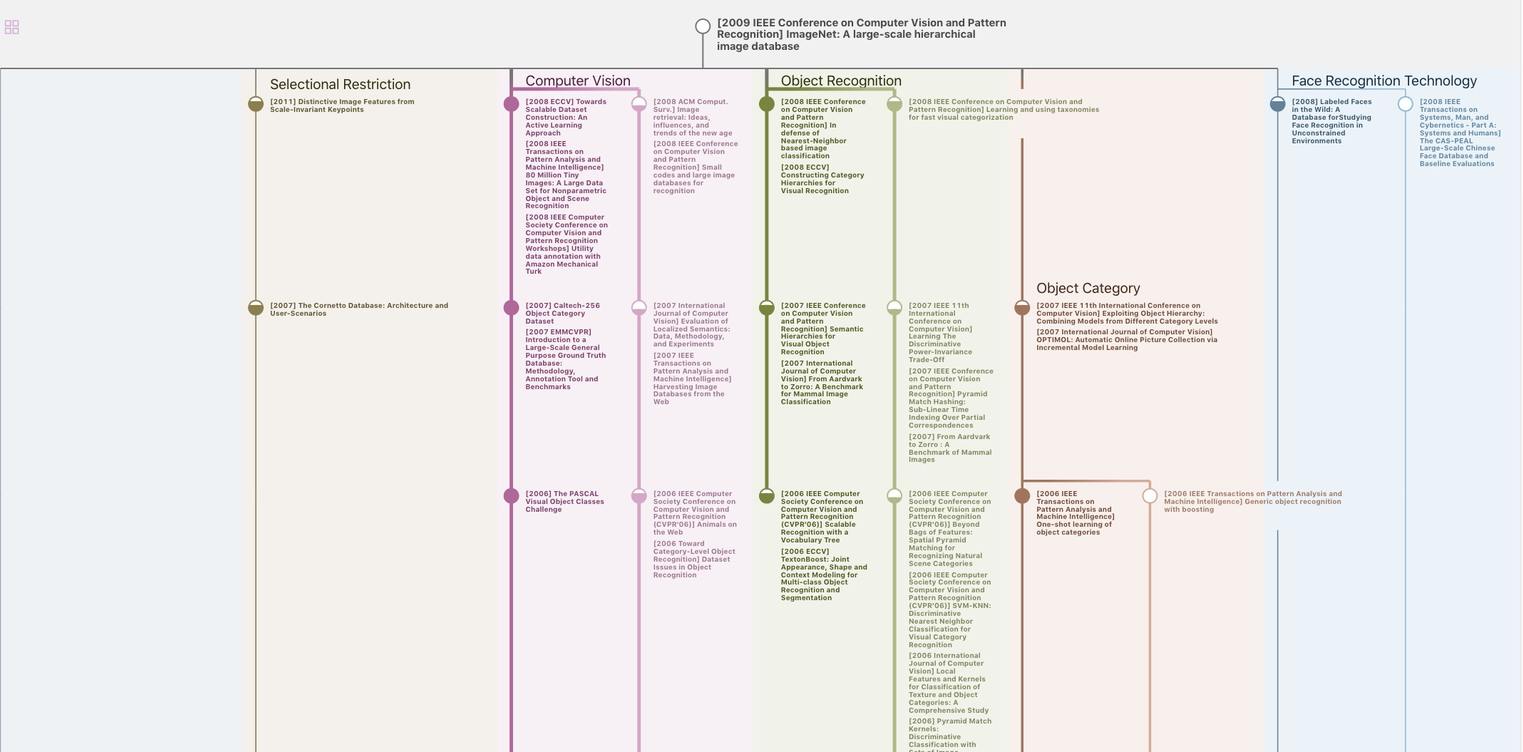
生成溯源树,研究论文发展脉络
Chat Paper
正在生成论文摘要