Bidomain Modeling Paradigm for Pansharpening
MM '23: Proceedings of the 31st ACM International Conference on Multimedia(2023)
摘要
Pansharpening is a challenging low-level vision task whose aim is to learn the complementary representation between spectral information and spatial detail. Despite the remarkable progress, existing deep neural network (DNN) based pansharpening algorithms are still confronted with common limitations. 1) These methods rarely consider the local specificity of different spectral bands; 2) They often extract the global detail in the spatial domain, which ignore the task-related degradation, e.g., the down-sampling process of MS image, and also suffer from limited receptive field. In this work, we propose a novel bidomain modeling paradigm for pansharpening problem (dubbed as BiMPan), which takes into both local spectral specificity and global spatial detail. More specifically, we first customize the specialized source-discriminative adaptive convolution (SDAConv) for every spectral band instead of sharing the identical kernels across all bands like prior works. Then, we devise a novel Fourier global modeling module (FGMM), which is capable of embracing global information while benefiting the disentanglement of image degradation. By integrating the band-aware local feature and Fourier global detail from these two functional designs, we can fuse a texture-rich while visually pleasing high-resolution MS image. Extensive experiments demonstrate that the proposed framework achieves favorable performance against current state-of-the-art pansharpening methods. The code is available at https://github.com/coder-qicao/BiMPan.
更多查看译文
AI 理解论文
溯源树
样例
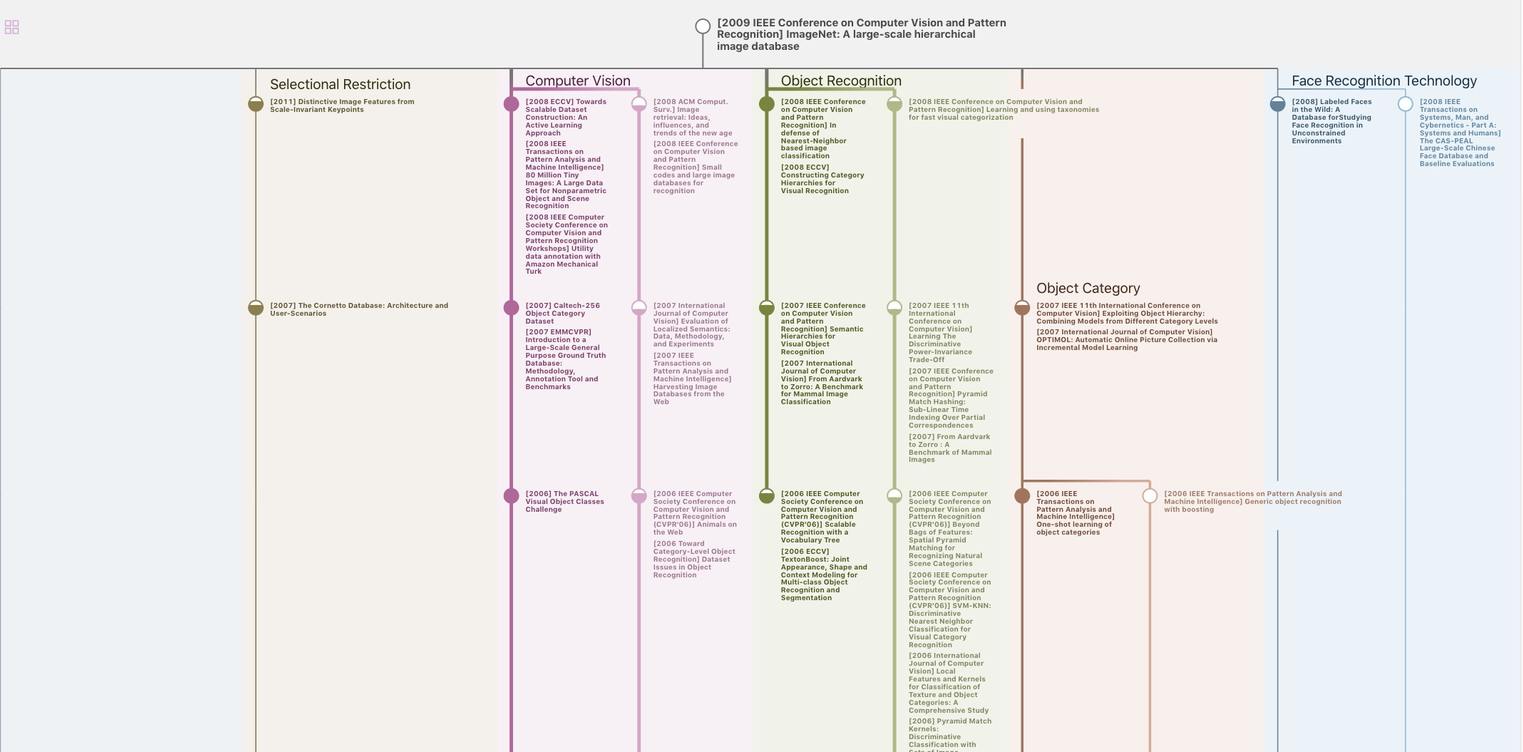
生成溯源树,研究论文发展脉络
Chat Paper
正在生成论文摘要