PointCRT: Detecting Backdoor in 3D Point Cloud via Corruption Robustness
MM '23: Proceedings of the 31st ACM International Conference on Multimedia(2023)
摘要
Backdoor attacks for point clouds have elicited mounting interest with the proliferation of deep learning. The point cloud classifiers can be vulnerable to malicious actors who seek to manipulate or fool the model with specific backdoor triggers. Detecting and rejecting backdoor samples during the inference stage can effectively alleviate backdoor attacks. Recently, some black-box test-time backdoor sample detection methods have been proposed in the 2D image domain, without any underlying assumptions about the backdoor triggers. However, upon examination, we have found that these detection techniques are not effective for 3D point clouds. As a result, there is a pressing need to bridge the gap for the development of a universal approach that is specifically designed for 3D point clouds. In this paper, we propose the first test-time backdoor sample detection method in 3D point cloud without assumption to the backdoor triggers, called Point Clouds Corruption Robustness Test (PointCRT). Based on the fact that the corruption robustness of clean samples remains relatively stable across various backdoor models, we propose the corruption robustness score to map the features into high-dimensional space. The corruption robustness score is a vector evaluated by label consistency, whose element is the minimum severity level of corruption that changes the label prediction of the victim model. Then, the trigger is identified by detecting the abnormal corruption robustness score through a nonlinear classification. The comprehensive experiments demonstrate PointCRT deals with all cases with the average AUC over 0.934 and F1 score over 0.864, with the enhancement of 18%-28% on ModelNet40. Our codes are available at: https://github.com/CGCL-codes/PointCRT.
更多查看译文
AI 理解论文
溯源树
样例
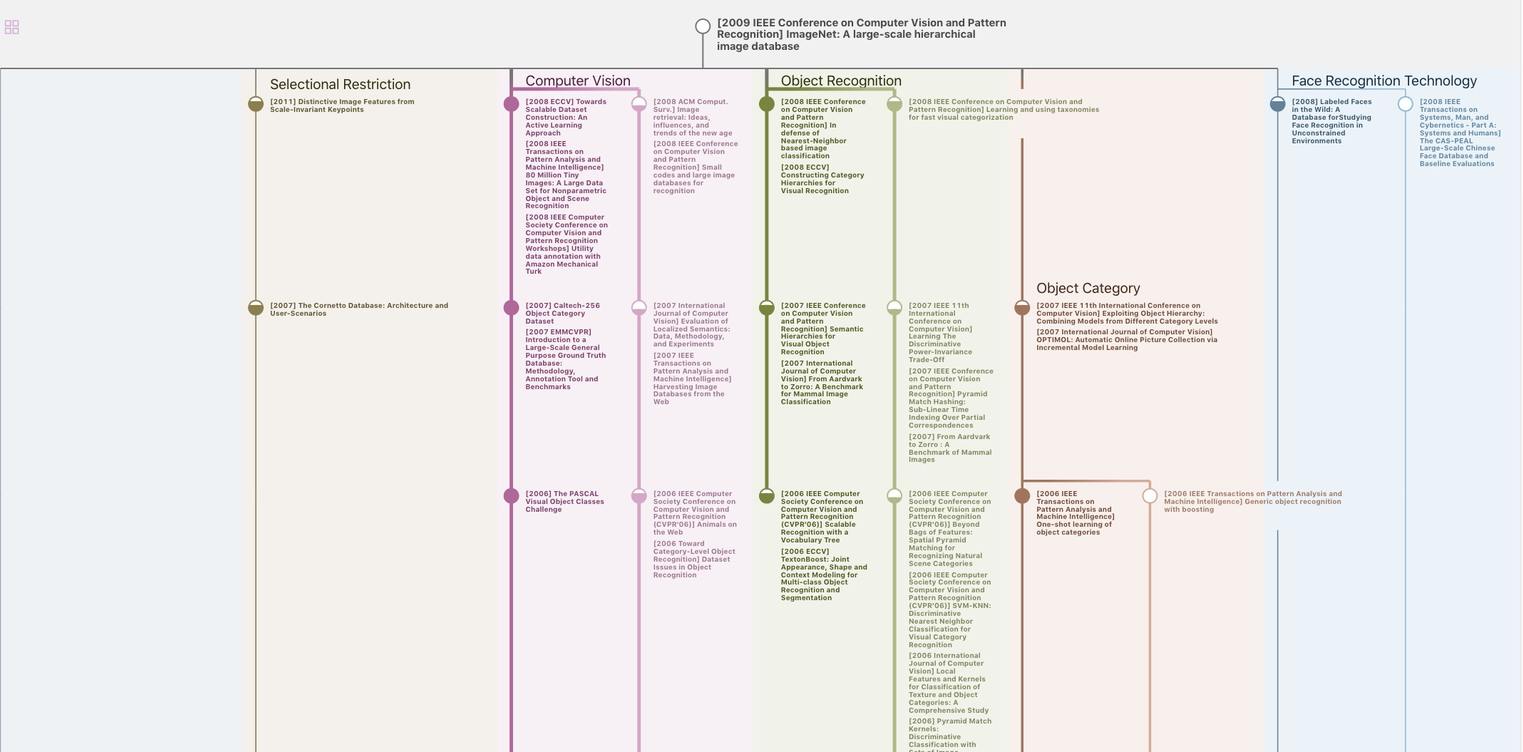
生成溯源树,研究论文发展脉络
Chat Paper
正在生成论文摘要