Layout-aware Single-image Document Flattening
ACM TRANSACTIONS ON GRAPHICS(2024)
摘要
Single image rectification of document deformation is a challenging task. Although some recent deep learning-based methods have attempted to solve this problem, they cannot achieve satisfactory results when dealing with document images with complex deformations. In this article, we propose a new efficient framework for document flattening. Our main insight is that most layout primitives in a document have rectangular outline shapes, making unwarping local layout primitives essentially homogeneous with unwarping the entire document. The former task is clearly more straightforward to solve than the latter due to the more consistent texture and relatively smooth deformation. On this basis, we propose a layout-aware deep model working in a divide-and-conquer manner. First, we employ a transformer-based segmentation module to obtain the layout information of the input document. Then a new regression module is applied to predict the global and local UV maps. Finally, we design an effective merging algorithm to correct the global prediction with local details. Both quantitative and qualitative experimental results demonstrate that our framework achieves favorable performance against state-of-the-art-methods. In addition, the current publicly available document flattening datasets have limited 3D paper shapes without layout annotation and also lack a general geometric correction metric. Therefore, we build a new-large-scale synthetic dataset by utilizing a fully automatic rendering method to generate deformed documents with diverse shapes and exact layout segmentation labels. We also propose a newgeometric correction metric based on our paired document UV maps. Code and dataset will be released at https://github.com/BunnySoCrazy/LA-DocFlatten
更多查看译文
关键词
Document image rectification,document layout analysis,deep neural networks,geometric models
AI 理解论文
溯源树
样例
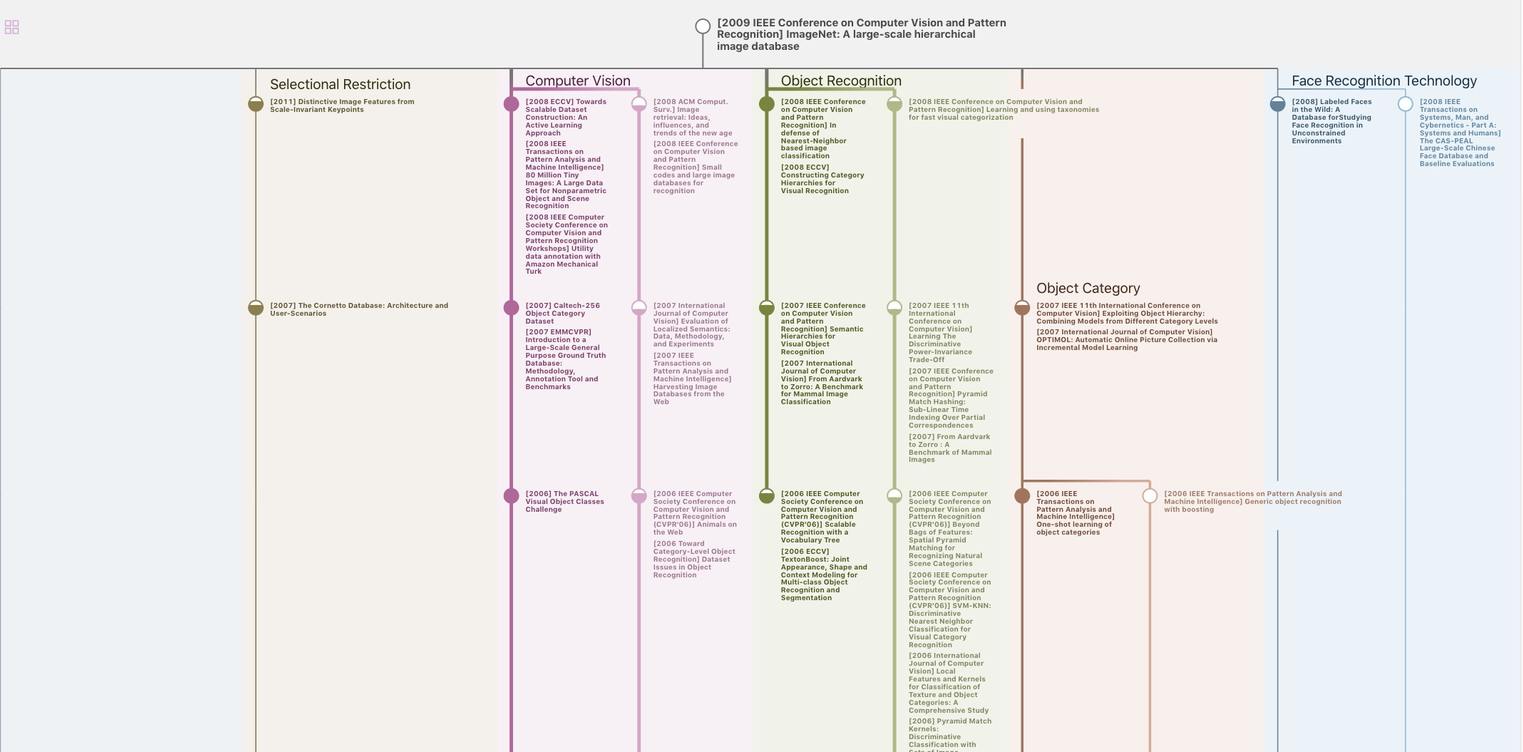
生成溯源树,研究论文发展脉络
Chat Paper
正在生成论文摘要