Improved pregnancy prediction performance in an updated deep-learning embryo selection model: a retrospective independent validation study
REPRODUCTIVE BIOMEDICINE ONLINE(2024)
摘要
Research question: What is the effect of increasing training data on the performance of ongoing pregnancy prediction after single vitrified -warmed blastocyst transfer (SVBT) in a deep -learning model? Design: A total of 3960 SVBT cycles were retrospectively analysed. Embryos were stratified according to the Society for Assisted Reproductive Technology age groups. Embryos were scored by deep -learning models iDAScore v1.0 (IDA-V1) and iDAScore v2.0 (IDA-V2) (15% more training data than v1.0) and by Gardner grading. The discriminative performance of the pregnancy prediction for each embryo scoring model was compared using the area under the curve (AUC) of the receiver operating characteristic curve for each maternal age group. Results: The AUC of iDA-V2, iDA-V1 and Gardener grading in all cohort were 0.736, 0.720 and 0.702, respectively. iDA-V2 was significantly higher than iDA-V1 and Gardener grading (P < 0.0001). Group > 35 years (n = 757): the AUC of iDA-V2 was significantly higher than Gardener grading (0.718 versus 0.694, P = 0.015); group aged 35-37 years (n = 821), the AUC of iDA-V2 was significantly higher than iDA-V1 (0.712 versus 0.696, P = 0.035); group aged 41-42 years (n = 715, the AUC of iDA-V2 was significantly higher than Gardener grading (0.745 versus 0.696, P = 0.007); group > 42 years (n = 660) and group aged 38-40 years (n =1007), no significant differences were found between the groups. Conclusion: The performance of deep learning models for pregnancy prediction will be improved by increasing the size of the training data.
更多查看译文
关键词
pregnancy prediction,single cryopreserved blastocyst transfer,artificial intelligence,deep learning model,blastocyst assessment
AI 理解论文
溯源树
样例
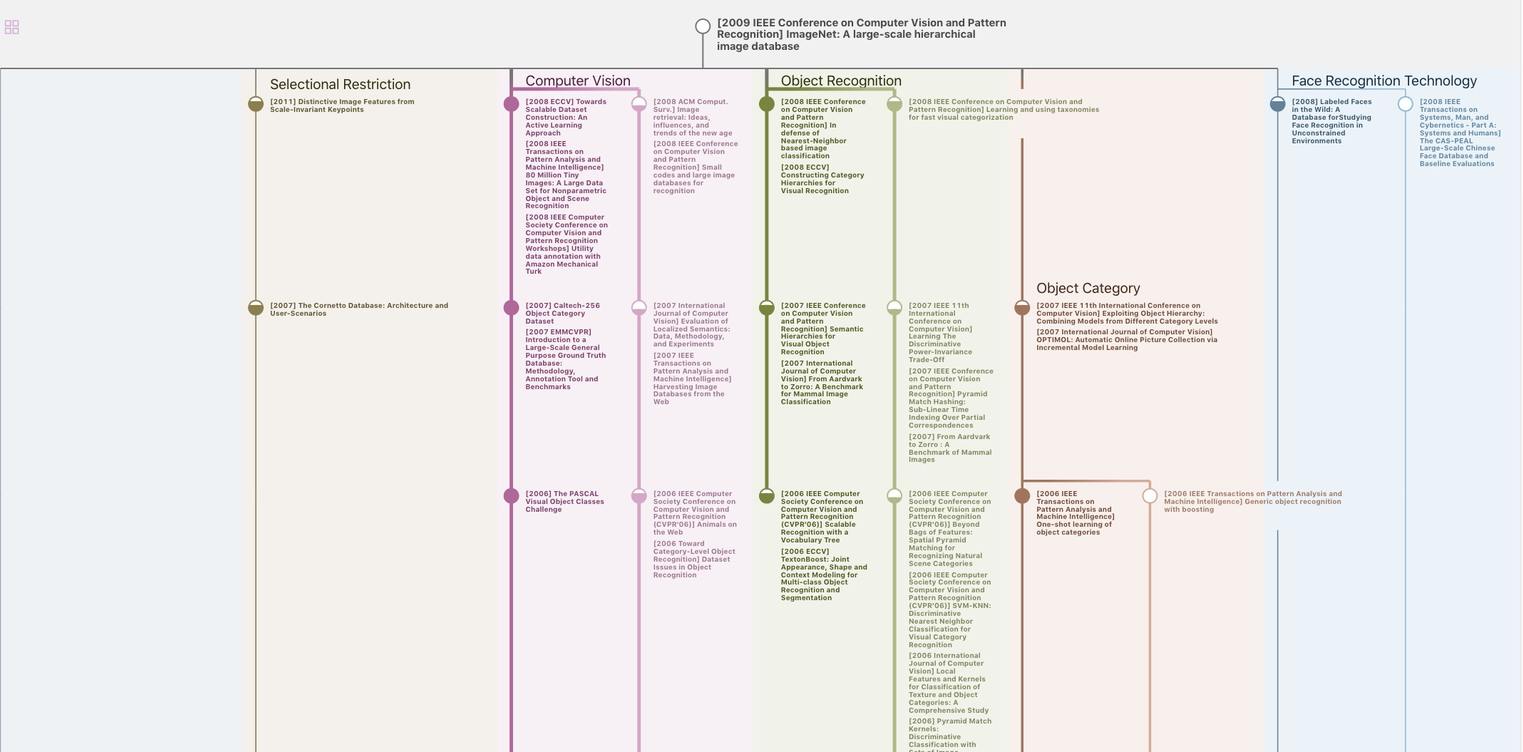
生成溯源树,研究论文发展脉络
Chat Paper
正在生成论文摘要