Potential for Machine Learning to Address Data Gaps in Human Toxicity and Ecotoxicity Characterization
ENVIRONMENTAL SCIENCE & TECHNOLOGY(2023)
摘要
Machine Learning (ML) is increasingly applied to fill data gaps in assessments to quantify impacts associated with chemical emissions and chemicals in products. However, the systematic application of ML-based approaches to fill chemical data gaps is still limited, and their potential for addressing a wide range of chemicals is unknown. We prioritized chemical-related parameters for chemical toxicity characterization to inform ML model development based on two criteria: (1) each parameter's relevance to robustly characterize chemical toxicity described by the uncertainty in characterization results attributable to each parameter and (2) the potential for ML-based approaches to predict parameter values for a wide range of chemicals described by the availability of chemicals with measured parameter data. We prioritized 13 out of 38 parameters for developing ML-based approaches, while flagging another nine with critical data gaps. For all prioritized parameters, we performed a chemical space analysis to assess further the potential for ML-based approaches to predict data for diverse chemicals considering the structural diversity of available measured data, showing that ML-based approaches can potentially predict 8-46% of marketed chemicals based on 1-10% with available measured data. Our results can systematically inform future ML model development efforts to address data gaps in chemical toxicity characterization.
更多查看译文
关键词
prioritization,uncertainty,chemical space,chemical properties,life cycleimpact assessment,chemical substitution,risk screening,safeand sustainable by design
AI 理解论文
溯源树
样例
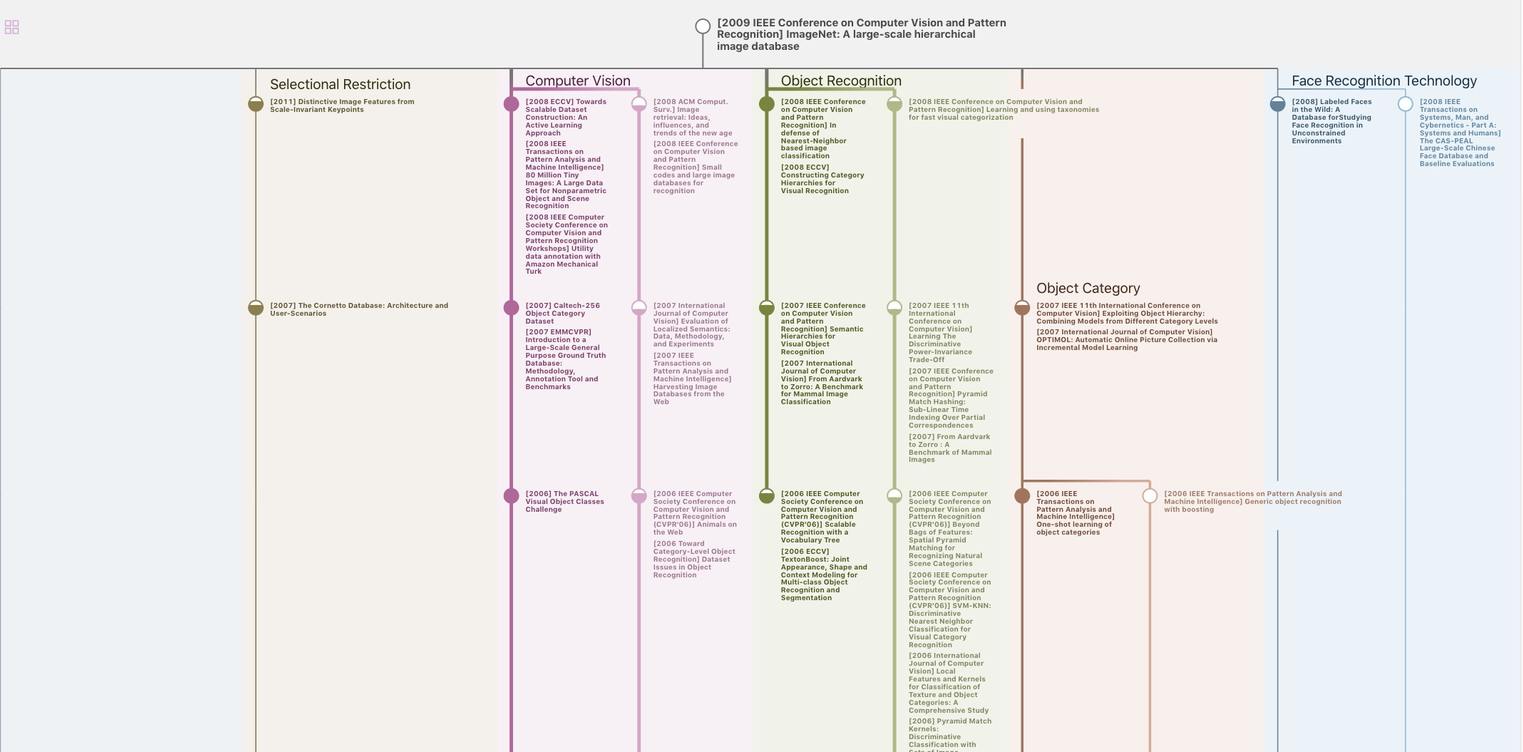
生成溯源树,研究论文发展脉络
Chat Paper
正在生成论文摘要