A machine learning-based Anomaly Detection Framework for building electricity consumption data
SUSTAINABLE ENERGY GRIDS & NETWORKS(2023)
摘要
A suboptimal management or system malfunction can often lead to abnormal energy consumptions in buildings, which result in a significant waste of energy. For this reason, the adoption of advanced monitoring systems, based on Machine Learning (ML) and visualization techniques, is crucial to avoid possible deviations from the baseline energy consumption. However, the historical data on which analyses are based generally do not report the occurrence of anomalies. Therefore, the application of supervised ML techniques is limited and unsupervised approaches are favored. Moreover, domain experts find most Machine Learning (ML) techniques hard to interpret, and thus find it difficult to contextualize anomalies. To overcome these issues, this work proposes a machine learning-based Anomaly Detection Framework (ADF) that involves the use of two complementary semi-supervised ML applications to obtain a highly interpretable and accurate detection of anomalies. Both techniques use Symbolic Aggregate approXimation (SAX) encoding to extract the most relevant information from load profiles. The aim of the first approach is to maximize the interpretability of the definition and distinction between anomalous and normal behavior. This is achieved using a Classification And Regression Tree (CART), albeit at the expense of a coarser output granularity. The second approach exploits an Multi-Layer Perceptron (MLP) algorithm to obtain a higher and more accurate output resolution, although it leads to a less interpretable definition of any anomalous behavior. The ADF has been applied to a real case study using electricity consumption data provided by a large telecommunications service provider. The results show that combining both ML models enhances the accuracy and interpretability of the detected anomalies.
更多查看译文
关键词
Anomaly detection,Electricity consumption,Machine learning,Semi-supervised,Synthetic ground truth,Symbolic aggregate approXimation,Smart meter
AI 理解论文
溯源树
样例
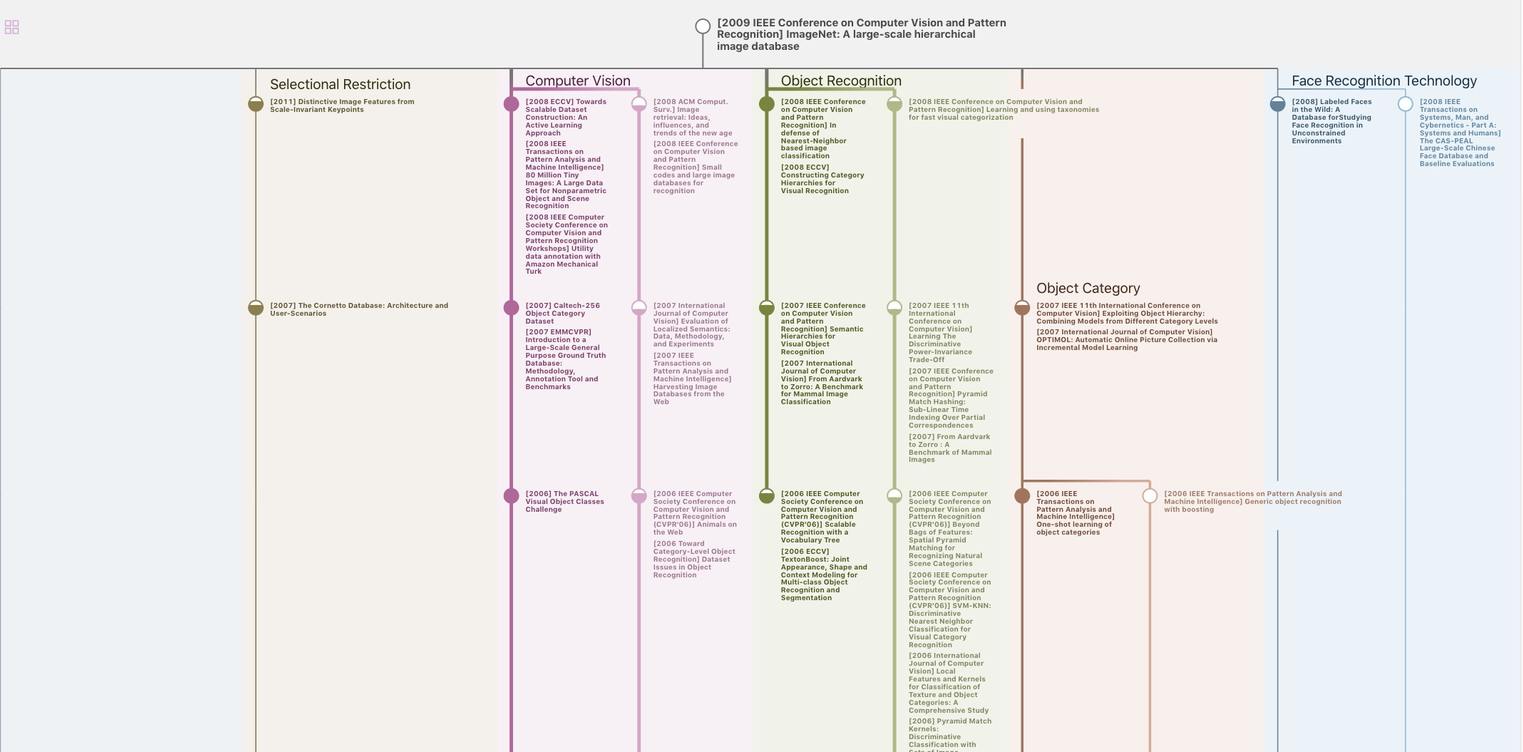
生成溯源树,研究论文发展脉络
Chat Paper
正在生成论文摘要