Constrained-Context Conditional Diffusion Models for Imitation Learning.
CoRR(2023)
摘要
Offline Imitation Learning (IL) is a powerful paradigm to learn visuomotor
skills, especially for high-precision manipulation tasks. However, IL methods
are prone to spurious correlation - expressive models may focus on distractors
that are irrelevant to action prediction - and are thus fragile in real-world
deployment. Prior methods have addressed this challenge by exploring different
model architectures and action representations. However, none were able to
balance between sample efficiency, robustness against distractors, and solving
high-precision manipulation tasks with complex action space. To this end, we
present $\textbf{C}$onstrained-$\textbf{C}$ontext $\textbf{C}$onditional
$\textbf{D}$iffusion $\textbf{M}$odel (C3DM), a diffusion model policy for
solving 6-DoF robotic manipulation tasks with high precision and ability to
ignore distractions. A key component of C3DM is a fixation step that helps the
action denoiser to focus on task-relevant regions around the predicted action
while ignoring distractors in the context. We empirically show that C3DM is
able to consistently achieve high success rate on a wide array of tasks,
ranging from table top manipulation to industrial kitting, that require varying
levels of precision and robustness to distractors. For details, please visit
this https://sites.google.com/view/c3dm-imitation-learning
更多查看译文
关键词
imitation learning,diffusion,models,constrained-context
AI 理解论文
溯源树
样例
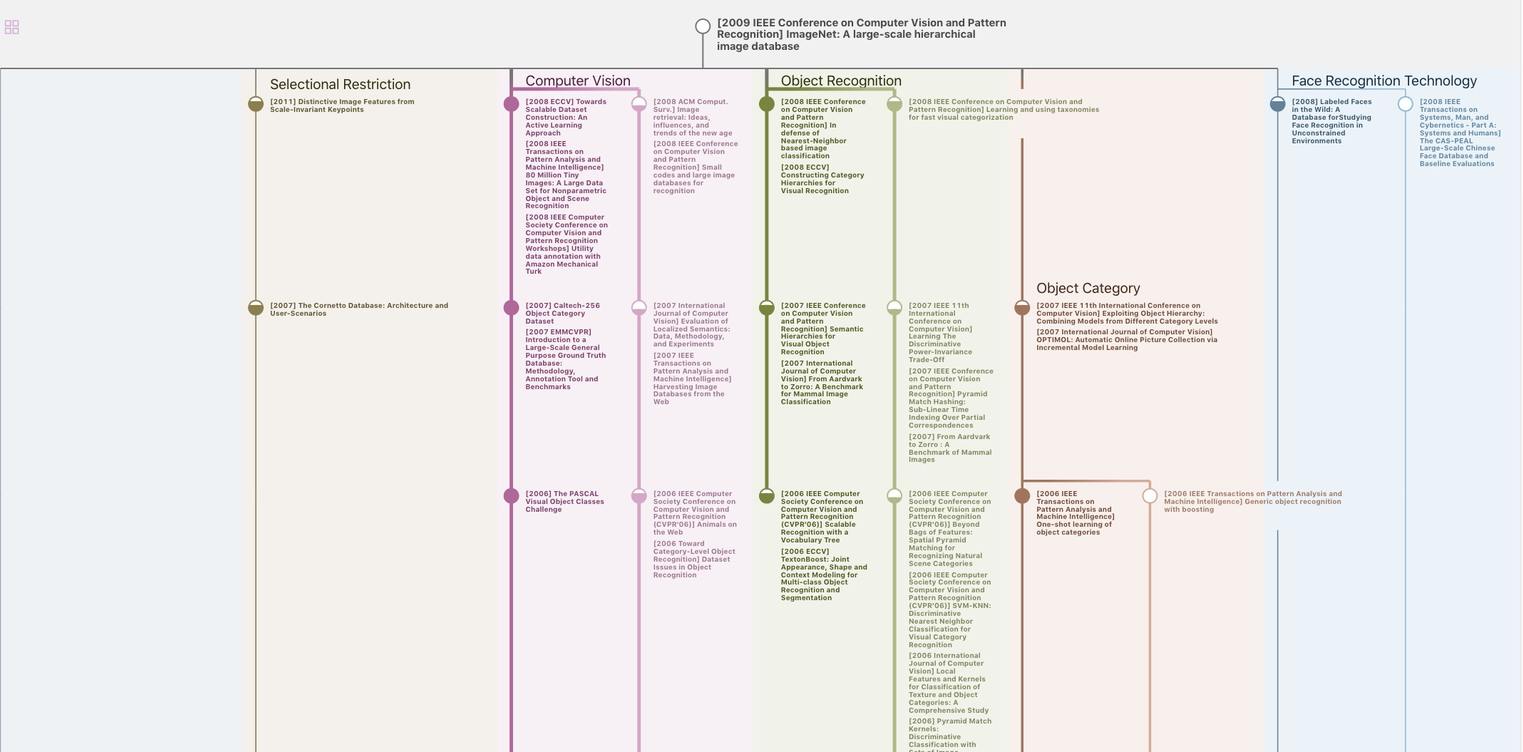
生成溯源树,研究论文发展脉络
Chat Paper
正在生成论文摘要