Learning Intra and Inter-Camera Invariance for Isolated Camera Supervised Person Re-identification.
MM '23: Proceedings of the 31st ACM International Conference on Multimedia(2023)
摘要
Supervised person re-identification assumes that a person has images captured
under multiple cameras. However when cameras are placed in distance, a person
rarely appears in more than one camera. This paper thus studies person re-ID
under such isolated camera supervised (ISCS) setting. Instead of trying to
generate fake cross-camera features like previous methods, we explore a novel
perspective by making efficient use of the variation in training data. Under
ISCS setting, a person only has limited images from a single camera, so the
camera bias becomes a critical issue confounding ID discrimination.
Cross-camera images are prone to being recognized as different IDs simply by
camera style. To eliminate the confounding effect of camera bias, we propose to
learn both intra- and inter-camera invariance under a unified framework. First,
we construct style-consistent environments via clustering, and perform
prototypical contrastive learning within each environment. Meanwhile, strongly
augmented images are contrasted with original prototypes to enforce
intra-camera augmentation invariance. For inter-camera invariance, we further
design a much improved variant of multi-camera negative loss that optimizes the
distance of multi-level negatives. The resulting model learns to be invariant
to both subtle and severe style variation within and cross-camera. On multiple
benchmarks, we conduct extensive experiments and validate the effectiveness and
superiority of the proposed method. Code will be available at
https://github.com/Terminator8758/IICI.
更多查看译文
关键词
isolated inter-camera,person,re-identification
AI 理解论文
溯源树
样例
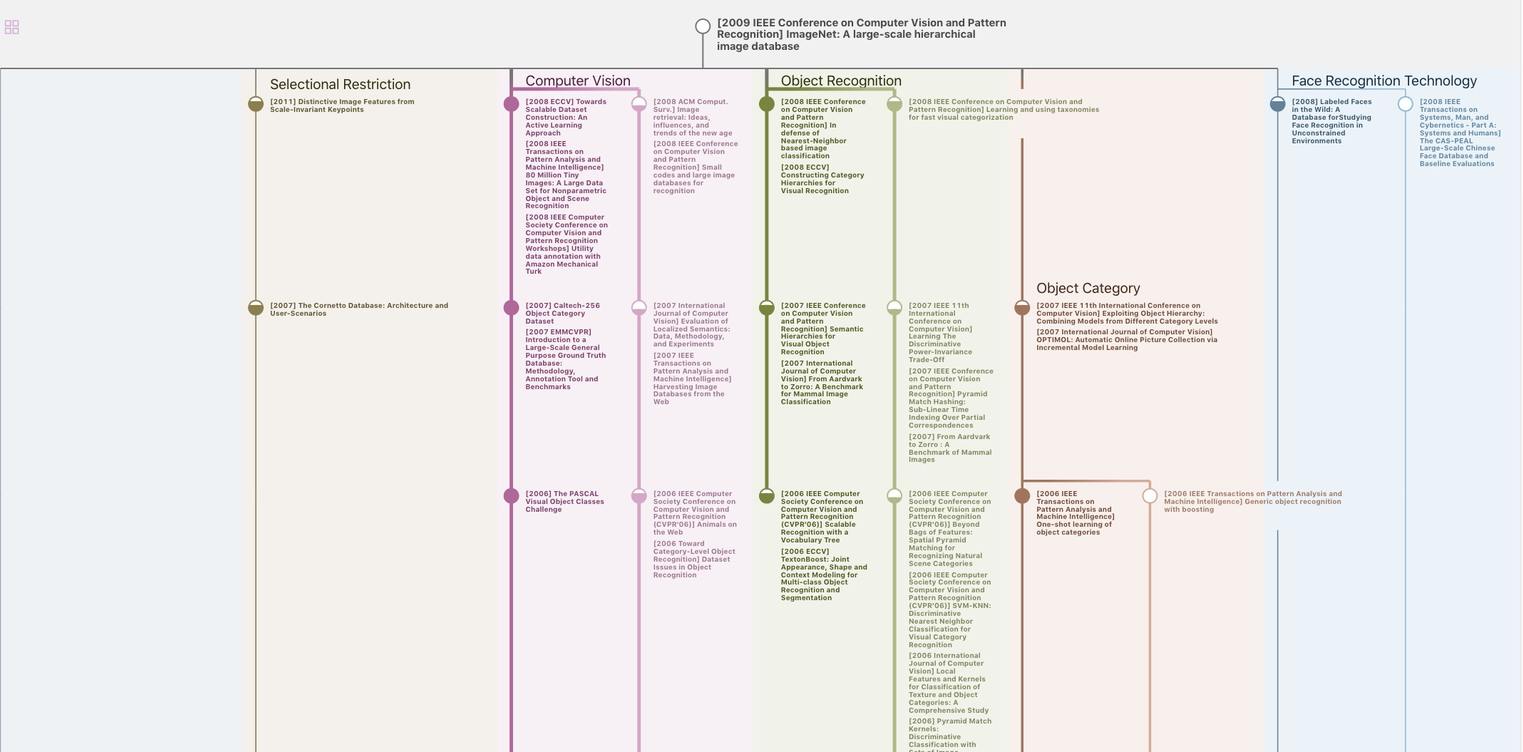
生成溯源树,研究论文发展脉络
Chat Paper
正在生成论文摘要