Add and Thin: Diffusion for Temporal Point Processes
NeurIPS(2023)
摘要
Autoregressive neural networks within the temporal point process (TPP)
framework have become the standard for modeling continuous-time event data.
Even though these models can expressively capture event sequences in a
one-step-ahead fashion, they are inherently limited for long-term forecasting
applications due to the accumulation of errors caused by their sequential
nature. To overcome these limitations, we derive ADD-THIN, a principled
probabilistic denoising diffusion model for TPPs that operates on entire event
sequences. Unlike existing diffusion approaches, ADD-THIN naturally handles
data with discrete and continuous components. In experiments on synthetic and
real-world datasets, our model matches the state-of-the-art TPP models in
density estimation and strongly outperforms them in forecasting.
更多查看译文
关键词
temporal point processes,diffusion
AI 理解论文
溯源树
样例
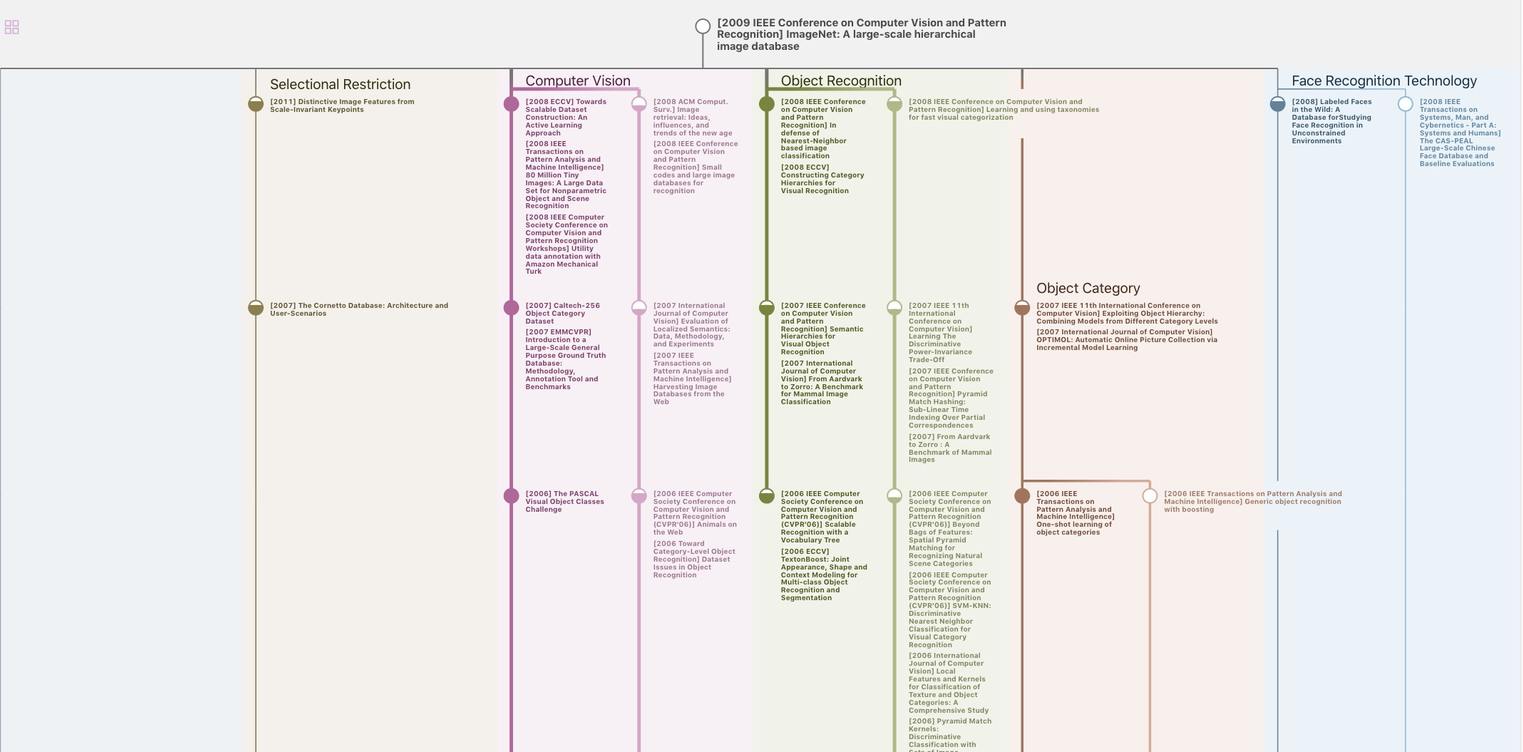
生成溯源树,研究论文发展脉络
Chat Paper
正在生成论文摘要