On The Open Prompt Challenge In Conditional Audio Generation
ICASSP 2024 - 2024 IEEE International Conference on Acoustics, Speech and Signal Processing (ICASSP)(2023)
摘要
Text-to-audio generation (TTA) produces audio from a text description,\nlearning from pairs of audio samples and hand-annotated text. However,\ncommercializing audio generation is challenging as user-input prompts are often\nunder-specified when compared to text descriptions used to train TTA models. In\nthis work, we treat TTA models as a ``blackbox'' and address the user prompt\nchallenge with two key insights: (1) User prompts are generally\nunder-specified, leading to a large alignment gap between user prompts and\ntraining prompts. (2) There is a distribution of audio descriptions for which\nTTA models are better at generating higher quality audio, which we refer to as\n``audionese''. To this end, we rewrite prompts with instruction-tuned models\nand propose utilizing text-audio alignment as feedback signals via margin\nranking learning for audio improvements. On both objective and subjective human\nevaluations, we observed marked improvements in both text-audio alignment and\nmusic audio quality.
更多查看译文
关键词
text-to-audio generation,prompt engineering,distributional drift
AI 理解论文
溯源树
样例
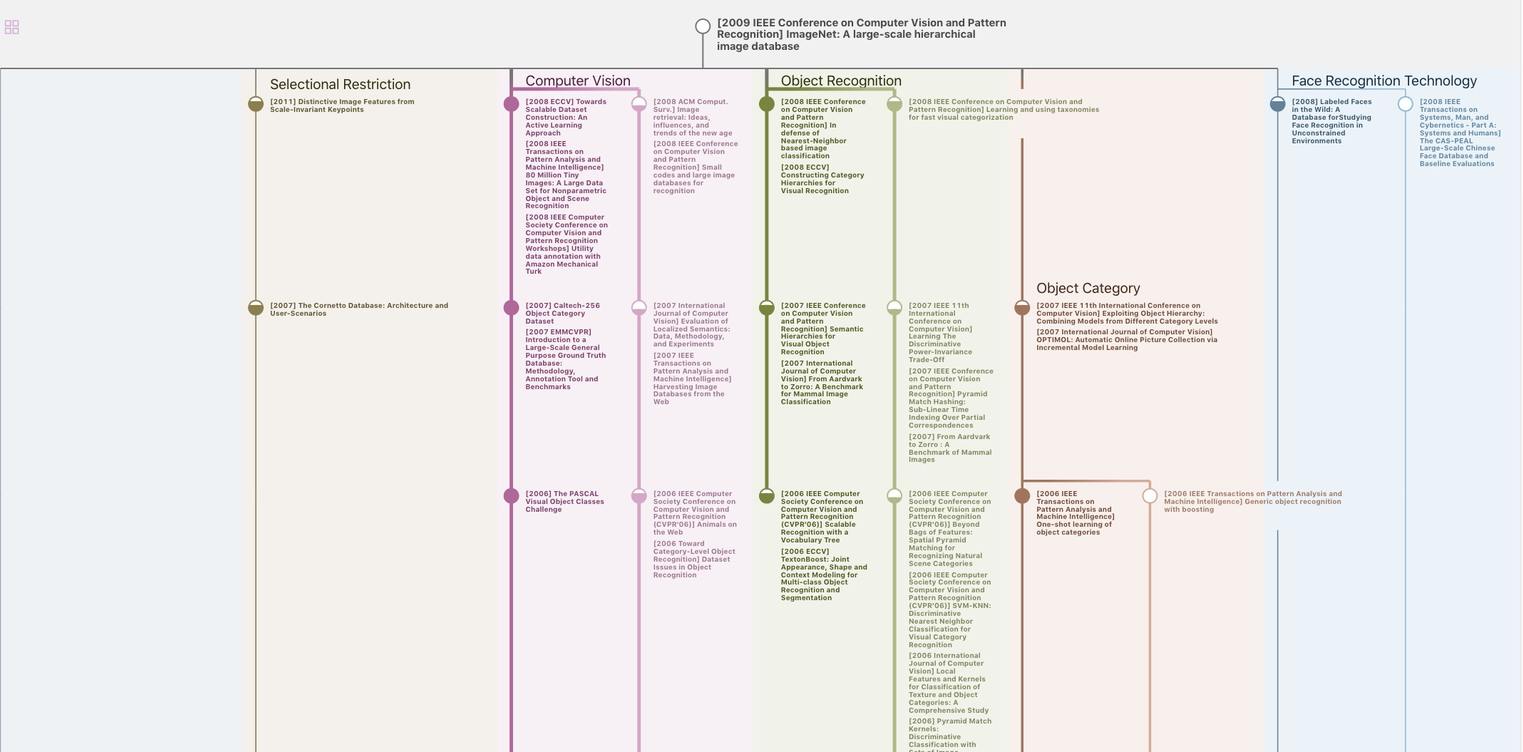
生成溯源树,研究论文发展脉络
Chat Paper
正在生成论文摘要