Can Large Language Models Design Accurate Label Functions?
CoRR(2023)
摘要
Programmatic weak supervision methodologies facilitate the expedited labeling
of extensive datasets through the use of label functions (LFs) that encapsulate
heuristic data sources. Nonetheless, the creation of precise LFs necessitates
domain expertise and substantial endeavors. Recent advances in pre-trained
language models (PLMs) have exhibited substantial potential across diverse
tasks. However, the capacity of PLMs to autonomously formulate accurate LFs
remains an underexplored domain. In this research, we address this gap by
introducing DataSculpt, an interactive framework that harnesses PLMs for the
automated generation of LFs. Within DataSculpt, we incorporate an array of
prompting techniques, instance selection strategies, and LF filtration methods
to explore the expansive design landscape. Ultimately, we conduct a thorough
assessment of DataSculpt's performance on 12 real-world datasets, encompassing
a range of tasks. This evaluation unveils both the strengths and limitations of
contemporary PLMs in LF design.
更多查看译文
关键词
large language models,language models,label
AI 理解论文
溯源树
样例
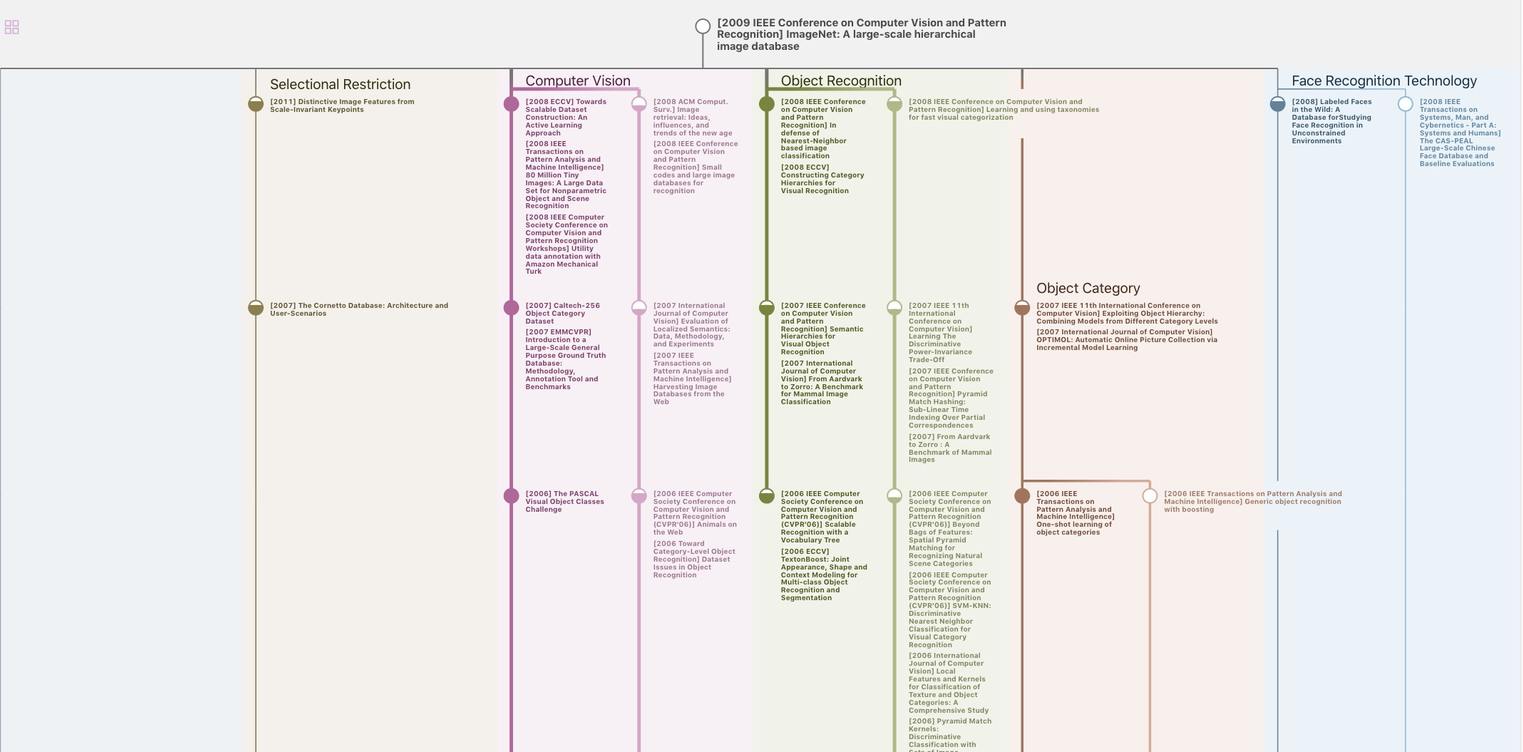
生成溯源树,研究论文发展脉络
Chat Paper
正在生成论文摘要