Soil temperature estimation at different depths over the central Tibetan Plateau integrating multiple Digital Earth observations and geo-computing
International Journal of Digital Earth(2023)
摘要
Soil temperature (ST) plays a critical role in ecosystems. Monitoring high-resolution ST profiles remains challenging due to the inherent heterogeneity of ST in space, time, and depth. To address this challenge, in this study, we integrate remote sensing techniques and deep learning methods to retrieve spatiotemporal continuous ST from 2011 to 2020 at four depths (5, 10, 20, and 40 cm) over the central Tibetan Plateau (TP). Landsat and MODIS observations were fused to obtain land surface properties at different resolutions. The fused variables were integrated with in-situ ST and soil moisture (SM) measurements to estimate spatiotemporally continuous (0.0005 degrees, 0.0025 degrees, and 0.0125 degrees) ST profile through deep learning-based training models. The deep belief network (DBN) was applied to estimate ST: (a) from layer to layer (LW-DBN), and (b) from land surface properties directly (Direct-DBN). The ten-fold cross-validation indicates that both approaches achieve promising results (R-2 > 0.836, MAE < 2.152 degrees C), and the Direct-DBN outperformed the LW-DBN at all spatial scales and depths. ST retrieval at deeper depths and coarser resolutions tend to have better monitoring accuracy. Further analysis indicates an increment of ST at all four depths over the study period, which provides valuable insights into global warming.
更多查看译文
关键词
Soil temperature profile,deep learning,Tibetan Plateau
AI 理解论文
溯源树
样例
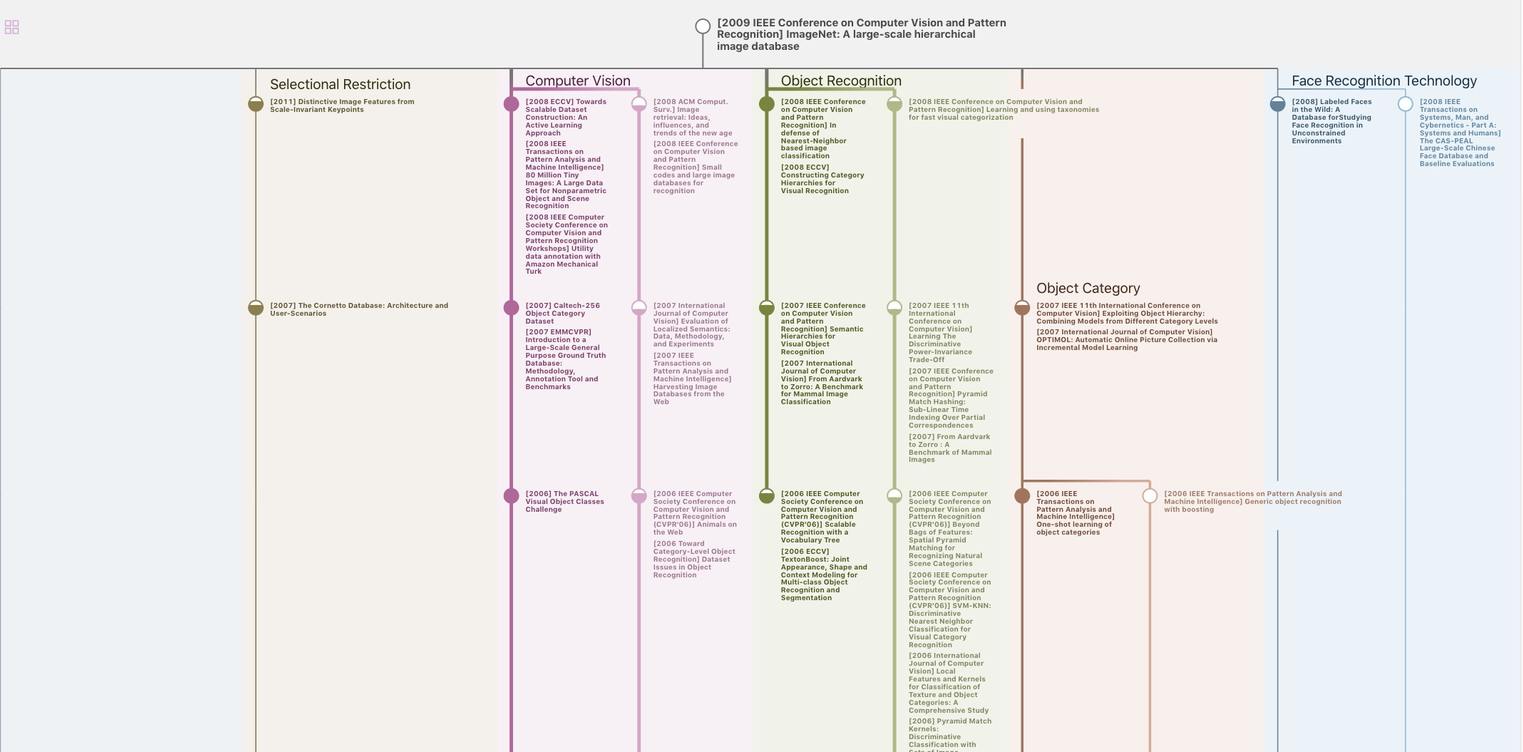
生成溯源树,研究论文发展脉络
Chat Paper
正在生成论文摘要