Machine learning-based estimation of gaseous and particulate emissions using internally observable vehicle operating parameters
URBAN CLIMATE(2023)
摘要
Measuring vehicular emissions is crucial for emission management and air quality control. However, conventional measurement equipment is costly and requires continuous maintenance. This study aims to address these challenges by introducing an Artificial Neural Network (ANN) based methodology that using internally observable vehicle operating parameters to estimate emissions of particulate number (PN), nitrogen oxide (NOx), carbon monoxide (CO), and carbon dioxide (CO2). The research was conducted in three steps: (1) analyzing the correlations between vehicle operating parameters and exhaust emissions through emission map analysis, (2) developing and validating artificial neural networks (ANNs), and (3) examining the impact of vehicle driving conditions on emission predictions. The ANNs were rigorously validated across various operating and ambient conditions, including different temperatures, cold and warmed-up starts, and various driving scenarios. The prediction accuracy for CO2 is higher than other exhaust gases, with an error of <1% in all validation cases. The mean prediction error for NOx was 12.5%, with observed variations in accuracy depending on dynamic and thermal conditions. The ANN model accurately predicted the influence of thermal conditions on CO emissions, which exhibit a significant increase at lower temperatures.
更多查看译文
关键词
Vehicle emission model,Particulate emissions,Gaseous emissions,Artificial neural network,Emission map
AI 理解论文
溯源树
样例
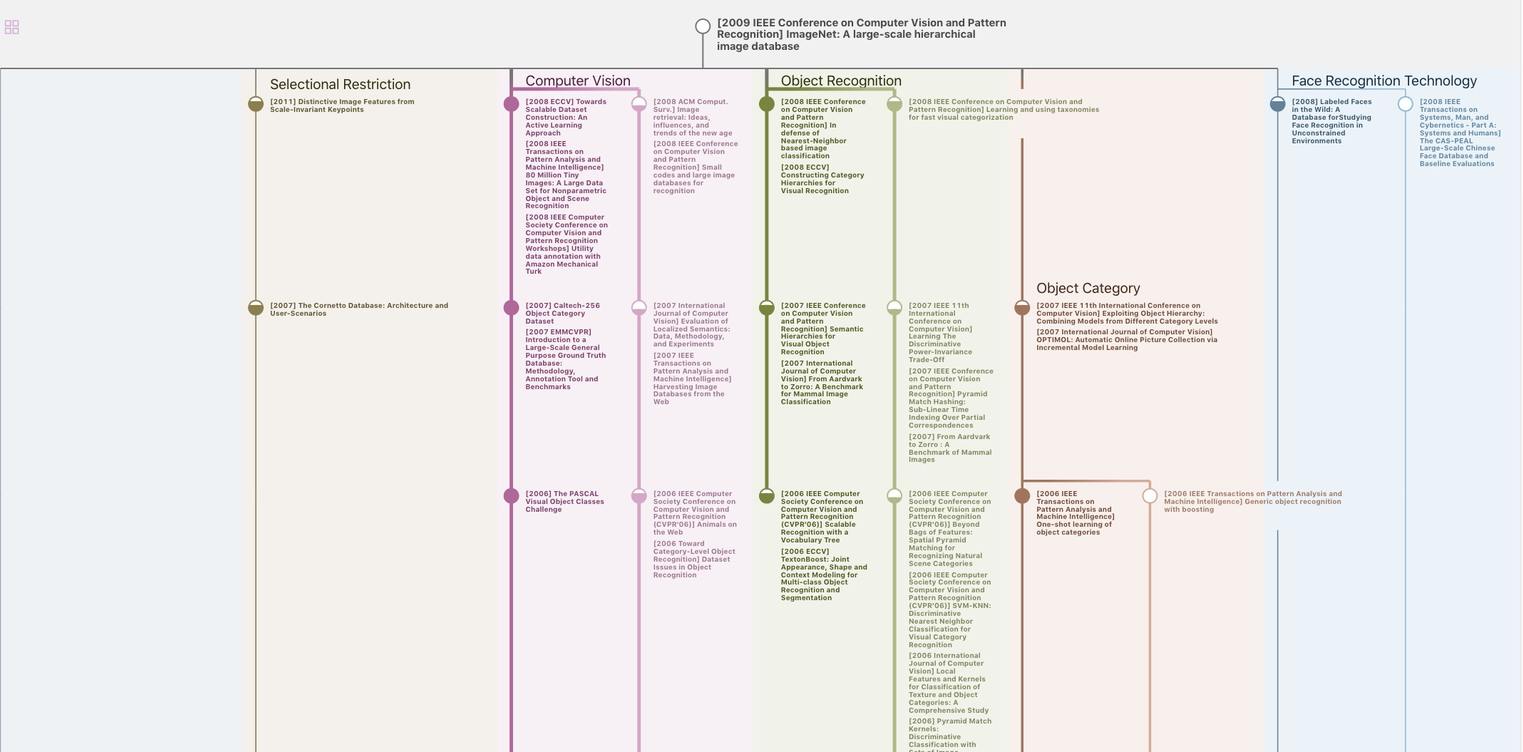
生成溯源树,研究论文发展脉络
Chat Paper
正在生成论文摘要