A smartphone accelerometer data-driven approach to recognize activities of daily life: A comparative study
Smart Health(2023)
摘要
Smartphones have become an indispensable part of our everyday life, influencing various aspects of our routines, from wake-up alarms to managing daily life activities. Nowadays, almost every smartphone has a built-in accelerometer sensor. Motivated by the notable increase in smartphone usage in our everyday life, in this research, we focus on harnessing the potential of smartphone accelerometers to recognize human daily life activities, aiming to leverage the usability and convenience of smartphones. We used smartphone accelerometer data from data collection to daily life activity recognition. To accomplish this, we first collected the smartphone's accelerometer data while performing five activities of daily living (ADLs) namely: moving downstairs, upstairs, running, standing, and walking, from 25 volunteers through a mobile application. After this, we extracted 15 statistical features from the smartphone's accelerometer data to efficiently classify the five referred ADLs. We then applied data pre-processing techniques, i.e., data cleaning and feature extraction. Afterward, we trained nine commonly used machine learning models to recognize five ADLs. Finally, we evaluated and compared the performance of all nine ML models to recognize each activity and analyzed the performance of these trained ML models to identify all five ADLs. The evaluated results revealed that the Adaboost (AB) classifier outperformed all other ML models with 100% area under the curve (AUC), precision, recall, accuracy, and F1-score for recognizing the five ADLs.
更多查看译文
关键词
daily life,smartphone,activities,data-driven
AI 理解论文
溯源树
样例
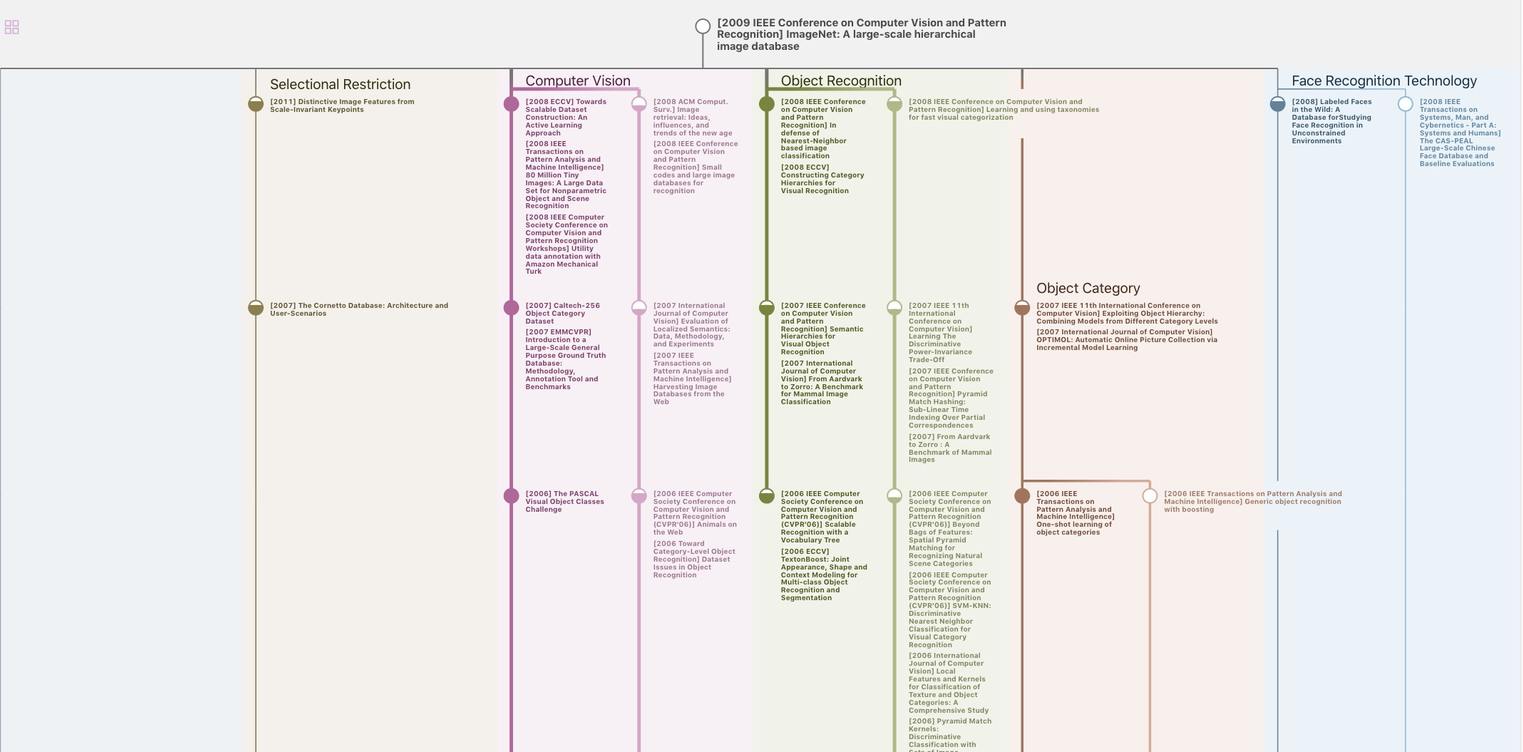
生成溯源树,研究论文发展脉络
Chat Paper
正在生成论文摘要