Controllable News Comment Generation based on Attribute Level Contrastive Learning
2023 IEEE International Conference on Intelligence and Security Informatics (ISI)(2023)
摘要
News comments provide a convenient way for people to express opinions and exchange ideas. Positive comments en-courage a harmonious discussion atmosphere within news media communities. In contrast, offensive or insulting comments may result in cyberbullying and personal psychological trauma, which have particular practical impacts in security-related domains. The automatic generation of news comments with controllable attributes (e.g. sentiment) to assist users and news platform administrators is greatly needed. However, existing research for news comment generation has not addressed the controllable issue yet. On the other hand, existing methods for controllable text generation focus on token-level constraints, which are not applicable to controlling the sentence level attributes for news comment generation. To address this challenging issue, in this paper, we propose an attribute-level contrastive learning method for controllable news comment generation. To apply attribute level constraints on the generated text, our method considers the attributes of the generated comments and the pre-defined attributes as different views of the same attribute, and maximizes their similarity during the training process. We conduct experiments on two publicly available news comment datasets, and the experimental results show that our model achieves competitive performance in news comment generation and attribute controllability.
更多查看译文
关键词
controllable text generation,news comment generation,attribute level constraints,contrastive learning
AI 理解论文
溯源树
样例
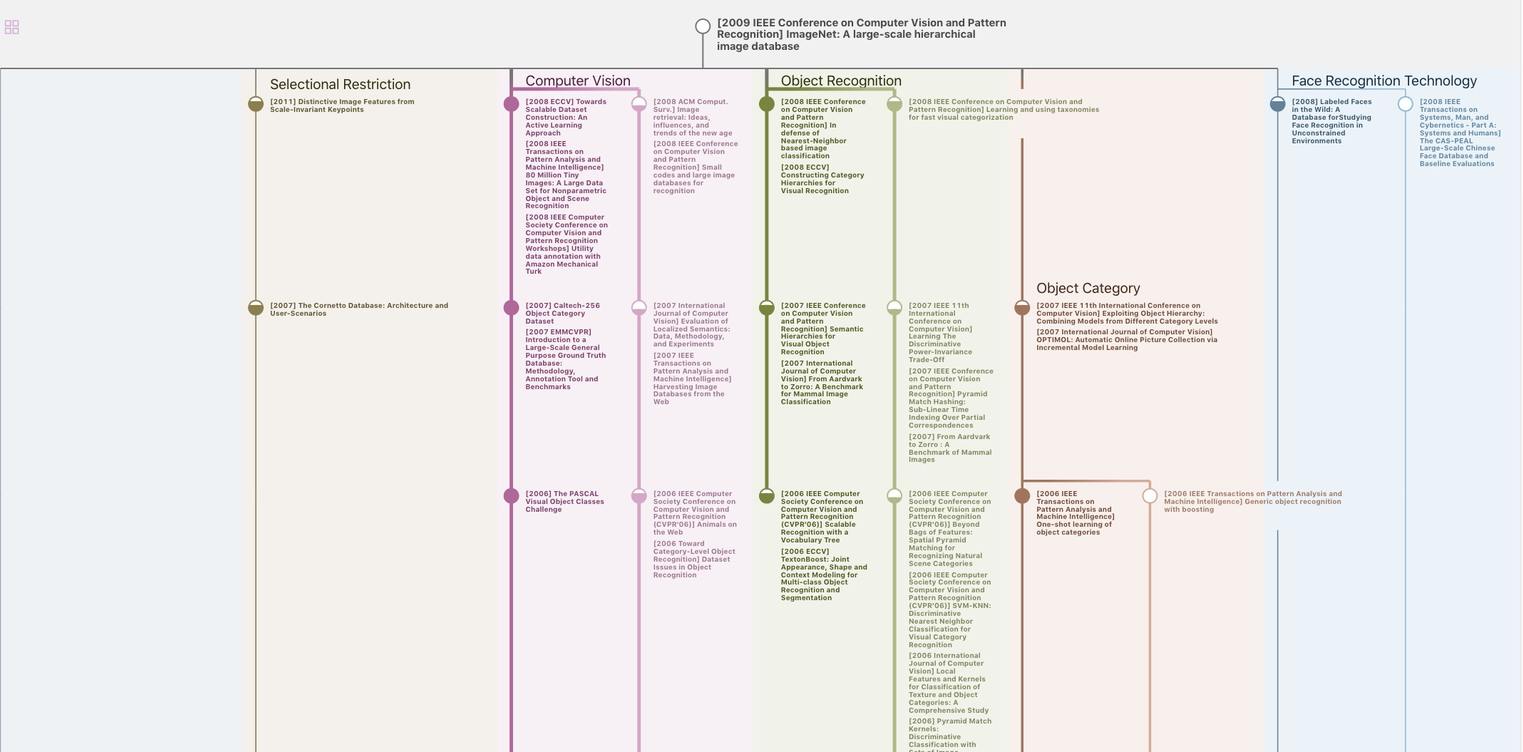
生成溯源树,研究论文发展脉络
Chat Paper
正在生成论文摘要