GTP-Force: Game-Theoretic Trajectory Prediction through Distributed Reinforcement Learning
2023 IEEE 20th International Conference on Mobile Ad Hoc and Smart Systems (MASS)(2023)
摘要
This paper introduces Game-theoretic Trajectory Prediction through distributed reinForcement learning (GTP-Force), a system that tackles the challenge of predicting joint pedestrian trajectories in multi-agent scenarios. GTP-Force utilizes decentralized reinforcement learning agents to personalize neural networks for each competing player based on their non-cooperative preferences and social interactions with others. By identifying the Nash Equilibria, GTP-Force accurately predicts joint trajectories while minimizing overall system loss in non-cooperative environments. The system outperforms existing state-of-the-art trajectory predictors, achieving an average displacement error of 0.19m on the ETH+UCY dataset and 80% accuracy on the Orange dataset, which is -0.01m and 5% better than the best-performing baseline, respectively. Additionally, GTP-Force considerably reduces the model size of social mobility predictors compared to approaches with classical game theory.
更多查看译文
关键词
Trajectory Prediction,Multi-Agent Social Interactions,Transformers,Reinforcement Learning,Neural Architecture Search,Non-cooperative Game Theory,Clustering
AI 理解论文
溯源树
样例
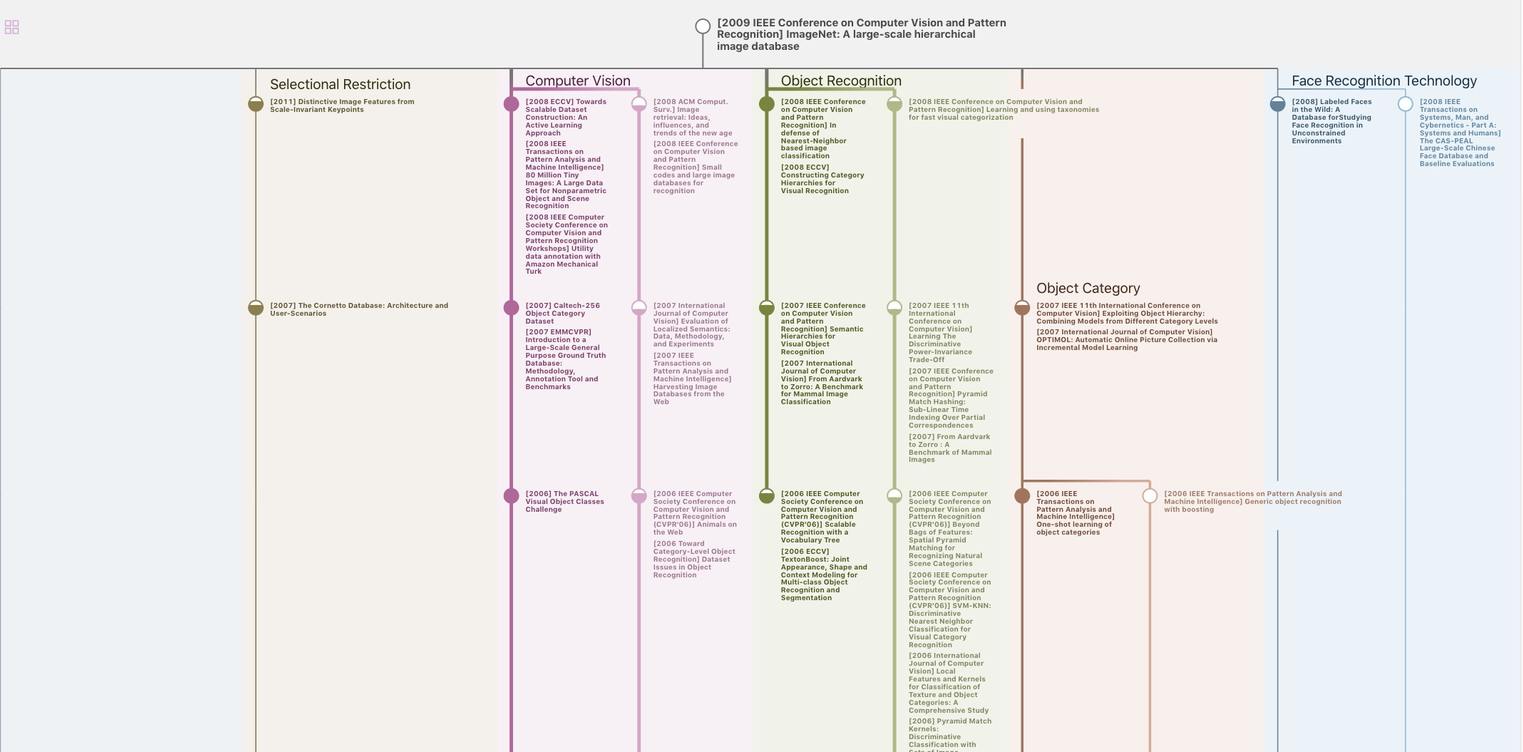
生成溯源树,研究论文发展脉络
Chat Paper
正在生成论文摘要