Extending Synthetic Data Evaluation Metrics
2023 IEEE 27th International Conference on Intelligent Engineering Systems (INES)(2023)
摘要
This paper explores the impact of the evolving evaluation metrics used to evaluate synthetic data and the related generative mechanism in the context of Differential Privacy (DP). Specifically, the authors provide an overview of current synthetic data evaluation methodologies and most commonly used metrics, identifying improvement possibilities. As the field continues to evolve, the authors also propose an improved version of a previously introduced comprehensive evaluation metric useful to redefine such evaluation frameworks in order to make them more complete and exhaustive. This proposed composite metric offers new ideas and directions for future comprehensive quantitative analysis of differentially private synthetic data and, thus, of their related generators.
更多查看译文
关键词
Synthetic Data,Evaluation Metrics,Data Evaluation Framework,Differential Privacy
AI 理解论文
溯源树
样例
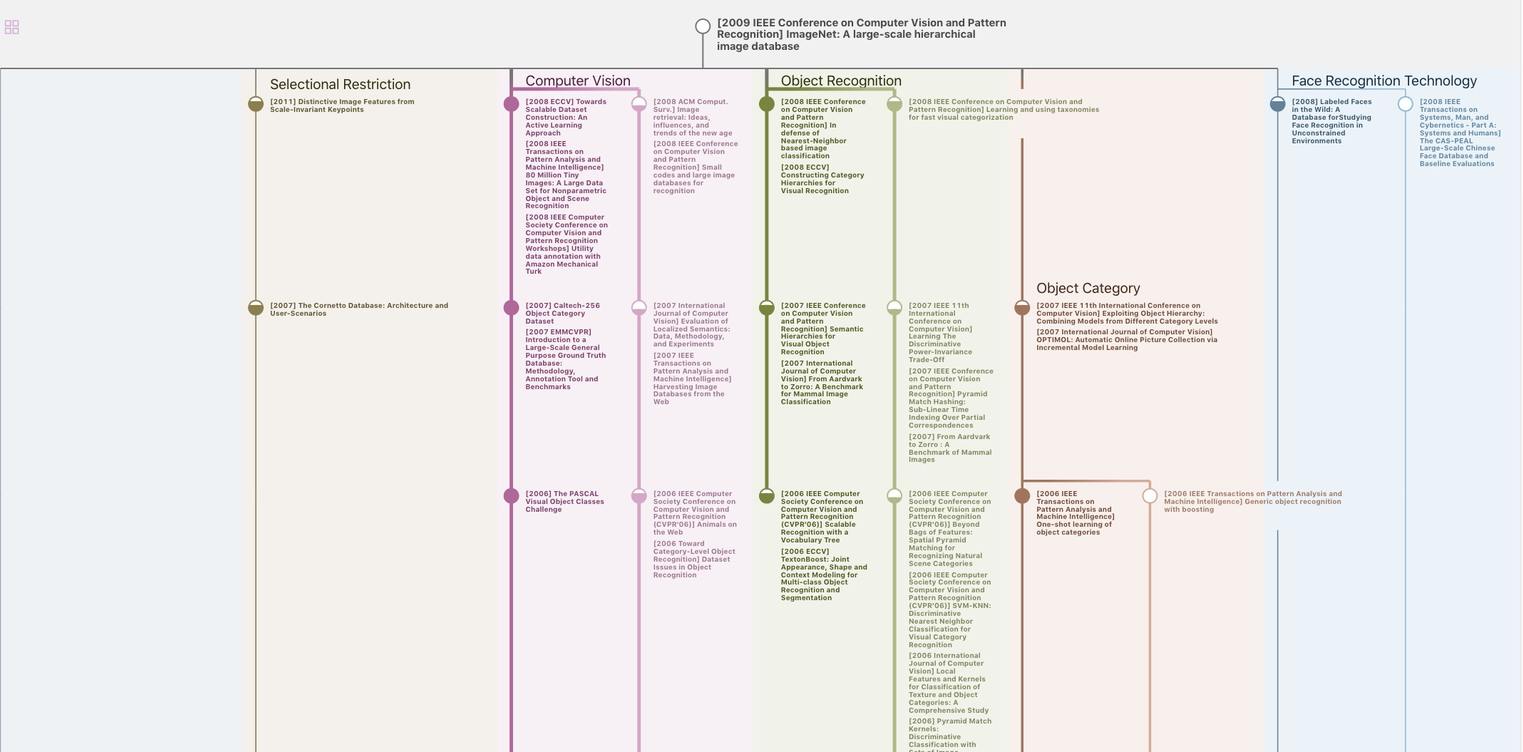
生成溯源树,研究论文发展脉络
Chat Paper
正在生成论文摘要