Data-driven Non-uniform Filterbanks Based on F-ratio for Machine Anomalous Sound Detection
2023 31st European Signal Processing Conference (EUSIPCO)(2023)
摘要
Anomalous sound detection (ASD) aims to detect unknown anomalous sounds emitted from a target machine. Most advanced ASD systems use a complicated neural-network- based detector with the log Mel spectrum as input. However, different types of machines have different vibration frequency regions depending on their physical property. The Mel filterbank (FB), which has high resolution in low-frequency regions and low resolution in high frequency, may filter out discriminative information from some important frequency regions, particularly the high-frequency regions. We propose to quantify the frequency importance in ASD of seven types of machines using the Fisher's ratio (F-ratio). The quantified frequency importance is then used to design an ensemble of machine-wise non-uniform FBs and extract the log non-uniform spectrum (LNS). This LNS feature is input to an autoencoder NN-based detector for anomalous sound detection. Experimental results in the DCASE2022 Challenge Task 2 verify the correctness of the quantification results and the effectiveness of the proposed LNS. With a simple autoencoder-based detector, the performance in the averaged harmonic mean of the area under the ROC curve achieved a relative improvement of 9.22 and 5.60% in development and evaluation datasets, respectively.
更多查看译文
关键词
Anomalous sound detection,frequencies importance,data-driven non-uniform filterbank,log non-uniform spectrum,F-ratio
AI 理解论文
溯源树
样例
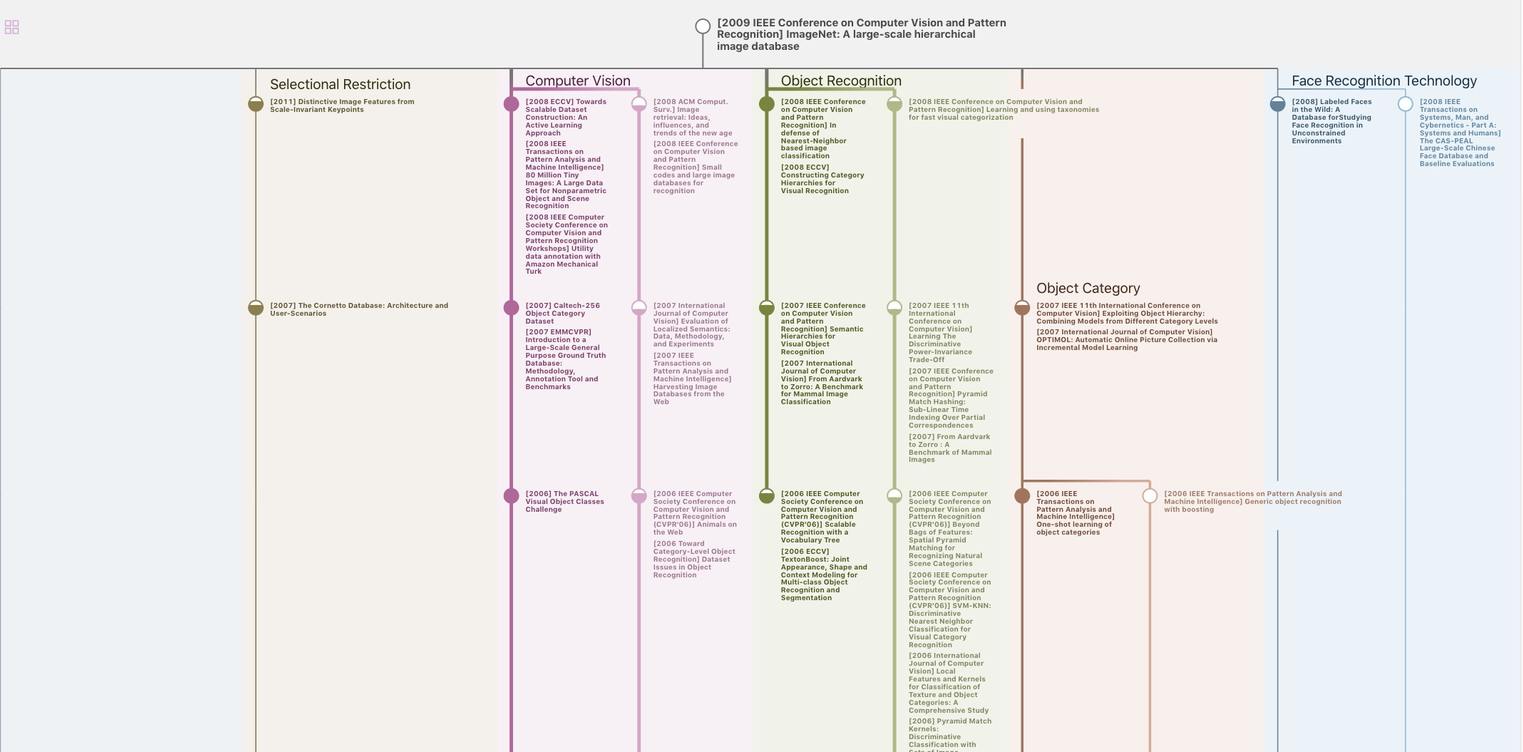
生成溯源树,研究论文发展脉络
Chat Paper
正在生成论文摘要