A comparative study for nuclei segmentation using latest deep learning optimizers
2023 5th Novel Intelligent and Leading Emerging Sciences Conference (NILES)(2023)
摘要
Nuclei segmentation is a critical task in biological image analysis, with numerous applications in cancer diagnosis, grading, staging, and treatment planning. However, this task is challenging, particularly when dealing with low-resolution and low signal-to-noise ratio microscopy images. Segmentation problems arise, such as touching and missing cells, which make the process even more challenging. Deep learning models, including Attention U-Net and TransUNet, have demonstrated exceptional performance in medical image segmentation. Nonetheless, the choice of optimizer can significantly impact the model’s performance, especially in nuclei segmentation. In this work, we assessed the efficiency of the latest deep learning optimizers, such as Ranger and GC, on Attention U-Net and TransUNet models and compared their performance with traditional optimizers, i.e., Adam. We conducted experiments on the publicly available Fluo-N2DH-HeLa dataset that was adapted from the cell tracking challenge. The Ranger optimizer, along with the TransUNet model, achieved the highest mean IOU of 95.05% and reasonable recall and precision scores of 94.4% and 96.8%, respectively. The experimental results proved that integrating the TransUNet model with the Ranger optimizer significantly enhanced touching nuclei segmentation performance in fluorescence microscopy images.
更多查看译文
关键词
Ranger optimizer,Attention U-Net,Trans Unet,2D cell nuclei segmentation
AI 理解论文
溯源树
样例
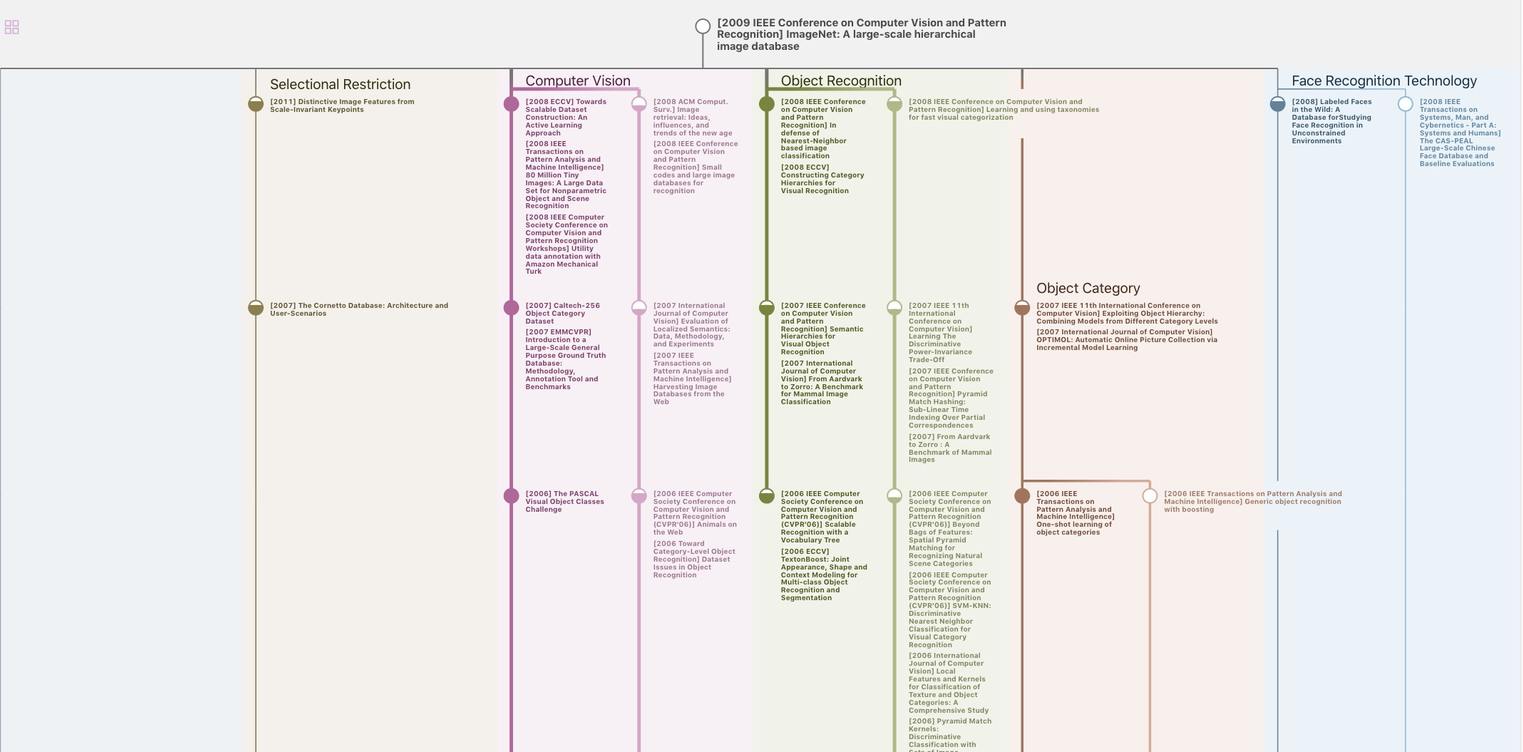
生成溯源树,研究论文发展脉络
Chat Paper
正在生成论文摘要