Automatic Noise Generation and Reduction for Text Classification
IEEE-ACM TRANSACTIONS ON AUDIO SPEECH AND LANGUAGE PROCESSING(2024)
摘要
Label noise is an important issue in machine learning, which might lead to negative influences on various tasks. Given that real benchmarks for evaluation of noise reduction methods are limited, plenty of studies construct pseudo noisy data to verify their proposed methods. However, very few works have realized the rationality of the noise generation strategies. If the generated pseudo datasets are biased, their final conclusions might also be problematic. In this work, we focus on text classification of natural language processing (NLP) to investigate various pseudo noise generation methods, which is the first work of this line for NLP. In particular, we compare the noise generated with crowdsourcing noise, a kind of real noise as gold-standard, to evaluate these noise generation methods. After then, we measure and compare the performance of representative noise reduction methods respectively based on the data of crowdsourcing and our top-ranked pseudo noisy generation strategies. We conduct experiments on five text classification datasets, offering detailed comparison results as well as discussions.
更多查看译文
关键词
Noise generation,noise reduction,pseudo noise,crowdsourcing
AI 理解论文
溯源树
样例
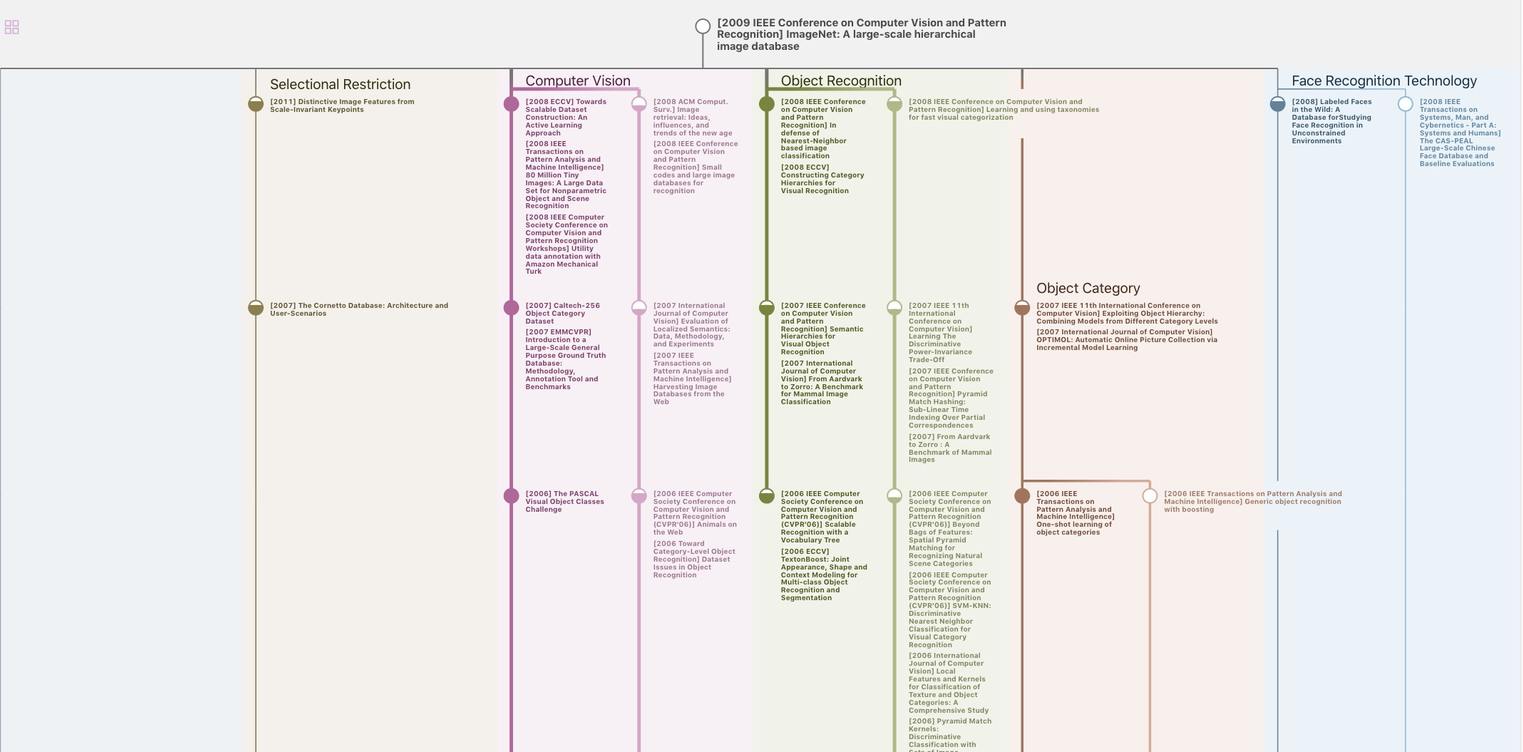
生成溯源树,研究论文发展脉络
Chat Paper
正在生成论文摘要