One-Shot Learning for Task-Oriented Grasping
IEEE ROBOTICS AND AUTOMATION LETTERS(2023)
摘要
Task-oriented grasping models aim to predict a suitable grasp pose on an object to fulfill a task. These systems have limited generalization capabilities to new tasks, but have shown the ability to generalize to novel objects by recognizing affordances. This object generalization comes at the cost of being unable to recognize the object category being grasped, which could lead to unpredictable or risky behaviors. To overcome these generalization limitations, we contribute a novel system for task-oriented grasping called the One-Shot Task-Oriented Grasping (OS-TOG) framework. OS-TOG comprises four interchangeable neural networks that interact through dependable reasoning components, resulting in a single system that predicts multiple grasp candidates for a specific object and task from multi-object scenes. Embedded one-shot learning models leverage references within a database for OS-TOG to generalize to novel objects and tasks more efficiently than existing alternatives. Additionally, the paper presents suitable candidates for the framework's neural components, covering essential adjustments for their integration and evaluative comparisons to state-of-the-art. In physical experiments with novel objects, OS-TOG recognizes 69.4% of detected objects correctly and predicts suitable task-oriented grasps with 82.3% accuracy, having a physical grasp success rate of 82.3%.
更多查看译文
关键词
Deep learning in grasping and manipulation,grasping,computer vision for automation,recognition
AI 理解论文
溯源树
样例
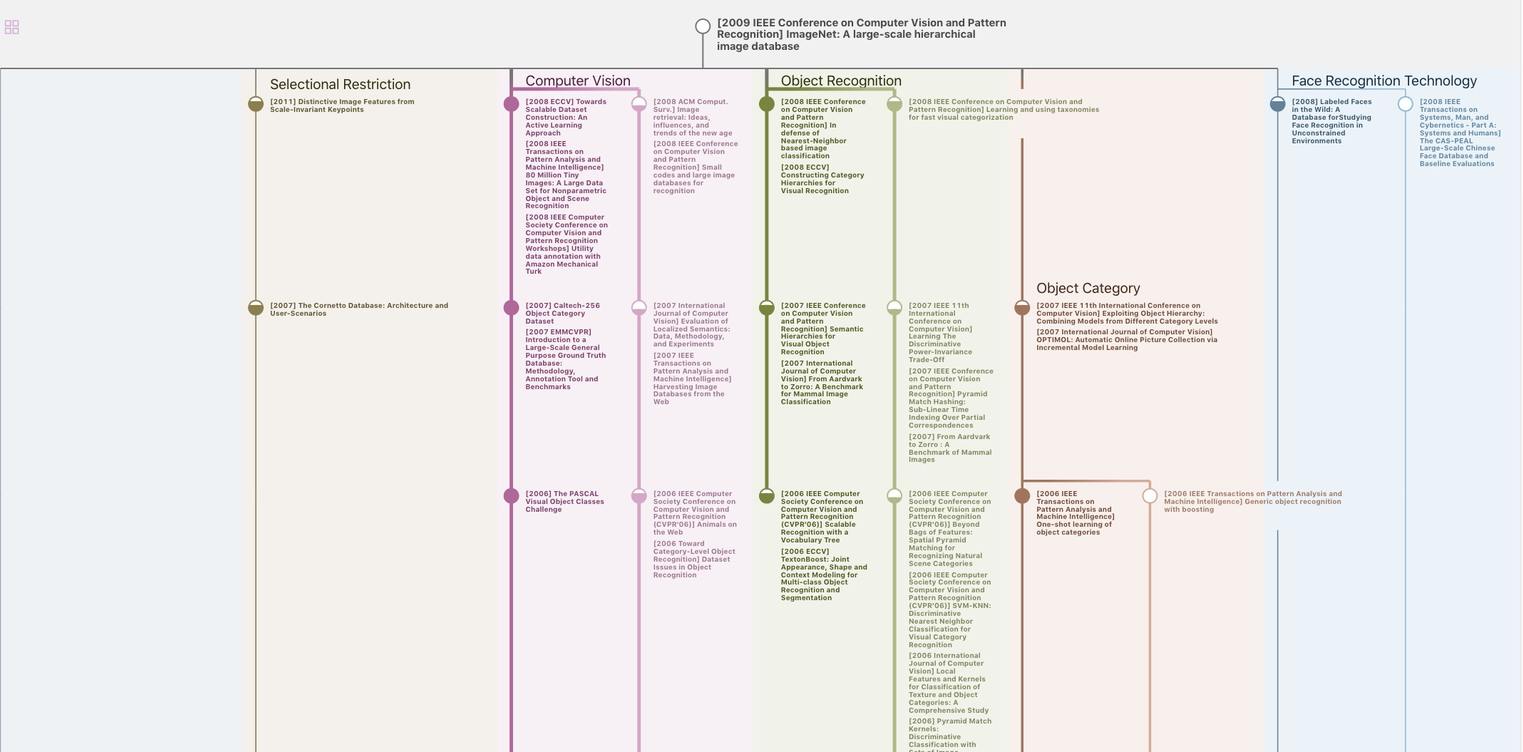
生成溯源树,研究论文发展脉络
Chat Paper
正在生成论文摘要