Are Large Language Models Reliable Judges? A Study on the Factuality Evaluation Capabilities of LLMs.
CoRR(2023)
摘要
In recent years, Large Language Models (LLMs) have gained immense attention due to their notable emergent capabilities, surpassing those seen in earlier language models. A particularly intriguing application of LLMs is their role as evaluators for texts produced by various generative models. In this study, we delve into the potential of LLMs as reliable assessors of factual consistency in summaries generated by text-generation models. Initially, we introduce an innovative approach for factuality assessment using LLMs. This entails employing a singular LLM for the entirety of the question-answering-based factuality scoring process. Following this, we examine the efficacy of various LLMs in direct factuality scoring, benchmarking them against traditional measures and human annotations. Contrary to initial expectations, our results indicate a lack of significant correlations between factuality metrics and human evaluations, specifically for GPT-4 and PaLM-2. Notable correlations were only observed with GPT-3.5 across two factuality subcategories. These consistent findings across various factual error categories suggest a fundamental limitation in the current LLMs' capability to accurately gauge factuality. This version presents the information more concisely while maintaining the main points and findings of the original text.
更多查看译文
关键词
large language models,language models,factuality
AI 理解论文
溯源树
样例
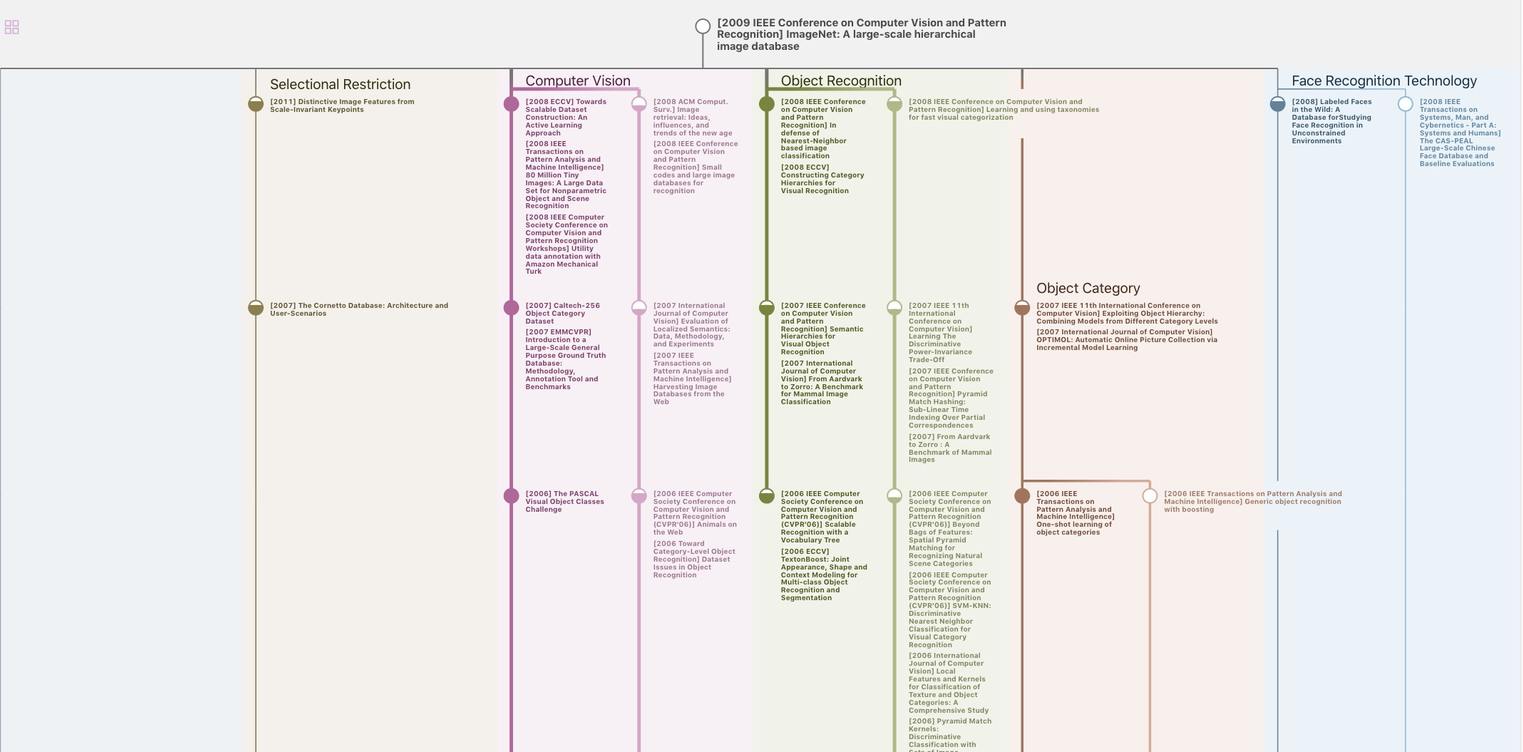
生成溯源树,研究论文发展脉络
Chat Paper
正在生成论文摘要