Robustness Tests for Automatic Machine Translation Metrics with Adversarial Attacks.
CoRR(2023)
摘要
We investigate MT evaluation metric performance on adversarially-synthesized texts, to shed light on metric robustness. We experiment with word- and character-level attacks on three popular machine translation metrics: BERTScore, BLEURT, and COMET. Our human experiments validate that automatic metrics tend to overpenalize adversarially-degraded translations. We also identify inconsistencies in BERTScore ratings, where it judges the original sentence and the adversarially-degraded one as similar, while judging the degraded translation as notably worse than the original with respect to the reference. We identify patterns of brittleness that motivate more robust metric development.
更多查看译文
关键词
automatic machine translation metrics
AI 理解论文
溯源树
样例
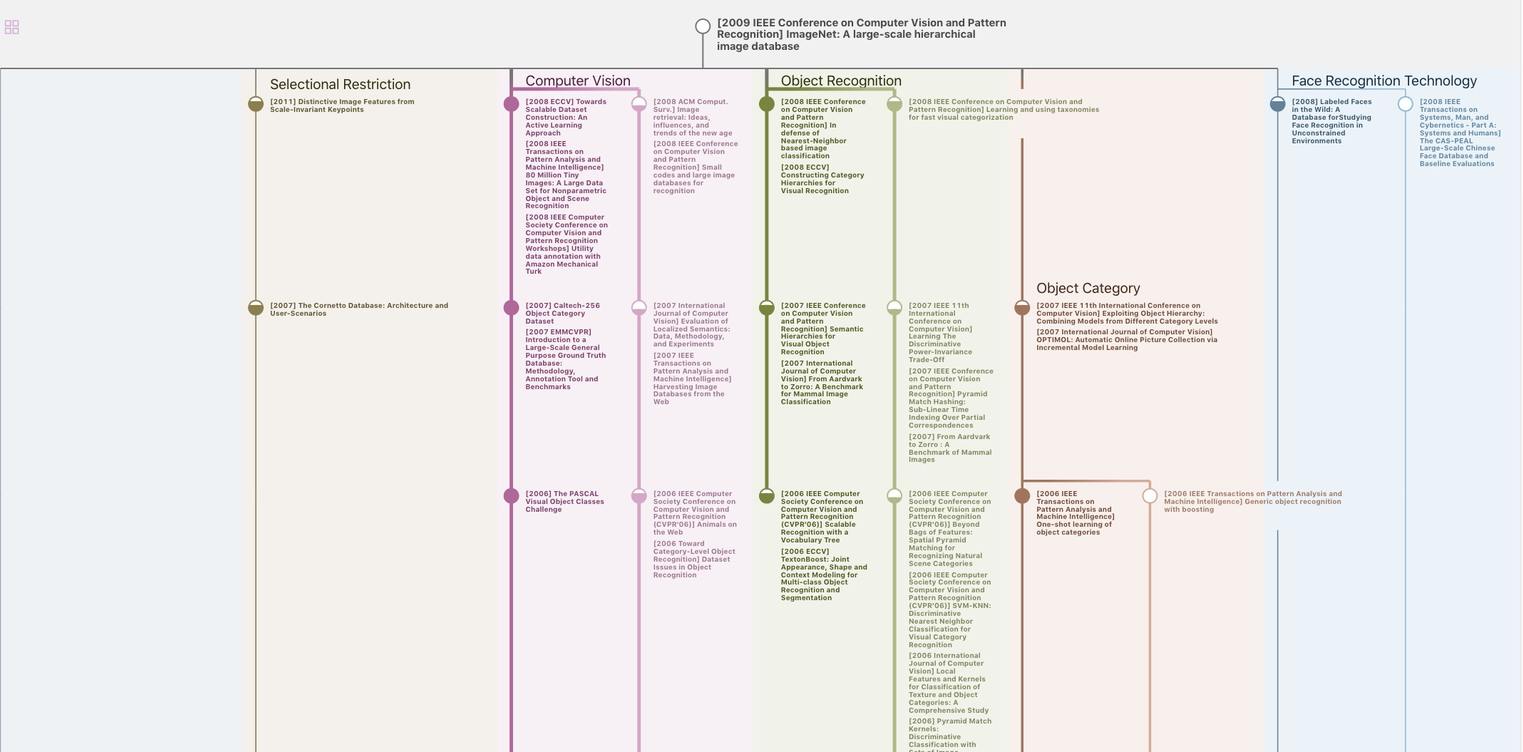
生成溯源树,研究论文发展脉络
Chat Paper
正在生成论文摘要