IBADR: an Iterative Bias-Aware Dataset Refinement Framework for Debiasing NLU models.
CoRR(2023)
摘要
As commonly-used methods for debiasing natural language understanding (NLU) models, dataset refinement approaches heavily rely on manual data analysis, and thus maybe unable to cover all the potential biased features. In this paper, we propose IBADR, an Iterative Bias-Aware Dataset Refinement framework, which debiases NLU models without predefining biased features. We maintain an iteratively expanded sample pool. Specifically, at each iteration, we first train a shallow model to quantify the bias degree of samples in the pool. Then, we pair each sample with a bias indicator representing its bias degree, and use these extended samples to train a sample generator. In this way, this generator can effectively learn the correspondence relationship between bias indicators and samples. Furthermore, we employ the generator to produce pseudo samples with fewer biased features by feeding specific bias indicators. Finally, we incorporate the generated pseudo samples into the pool. Experimental results and in-depth analyses on two NLU tasks show that IBADR not only significantly outperforms existing dataset refinement approaches, achieving SOTA, but also is compatible with model-centric methods.
更多查看译文
关键词
models,refinement,bias-aware
AI 理解论文
溯源树
样例
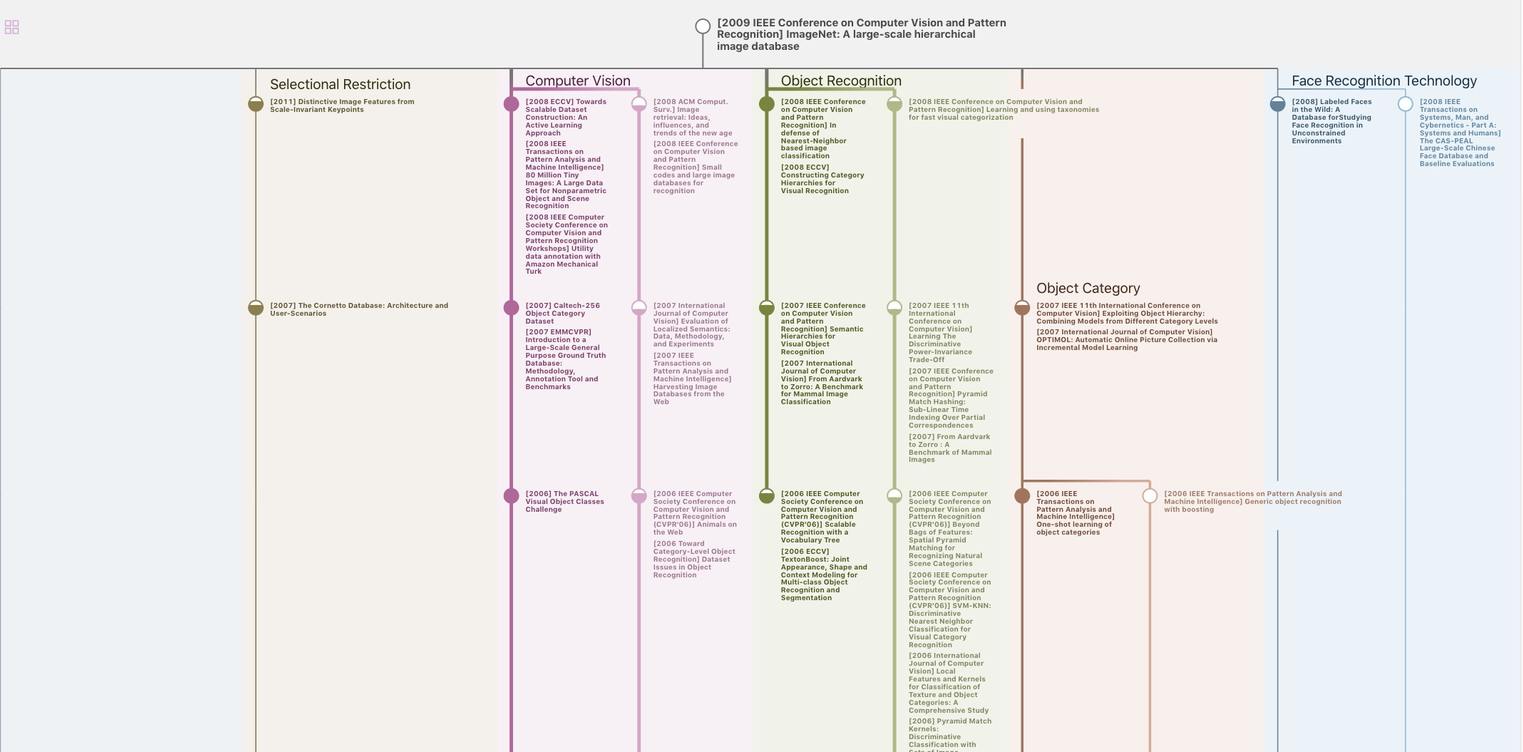
生成溯源树,研究论文发展脉络
Chat Paper
正在生成论文摘要