A composite surrogate-assisted evolutionary algorithm for expensive many-objective optimization
EXPERT SYSTEMS WITH APPLICATIONS(2024)
摘要
In many practical many-objective optimization problems, the computational cost of real evaluation is often high. To solve such problems, surrogate-assisted evolutionary algorithms (SAEAs) are effective methods that use low-cost surrogate models to approximate expensive real evaluation and select better-performing individuals for further evaluation. However, existing surrogate models accumulate prediction errors with increasing numbers of objectives, and the computational cost of constructing surrogate models also increases sharply. This paper proposes a composite surrogate-assisted evolutionary algorithm for expensive many-objective optimization. The crux of this method involves categorizing the surrogate models into two distinct types: a global model, constructed based on all costly real evaluations, and local models tailored to subsets derived from clustering all evaluations. Additionally, a new filling sampling criteria is proposed to guide the selection of solutions, considering the requirements of convergence, diversity, and uncertainty. Experimental results on multiple benchmark problems demonstrate that the proposed method is competitive compared to other popular surrogate-assisted evolutionary algorithms in solving expensive many-objective optimization problems within limited computational budgets.
更多查看译文
关键词
Expensive many-objective optimization,Filling sampling criteria,Evolutionary algorithms,Surrogate assisted
AI 理解论文
溯源树
样例
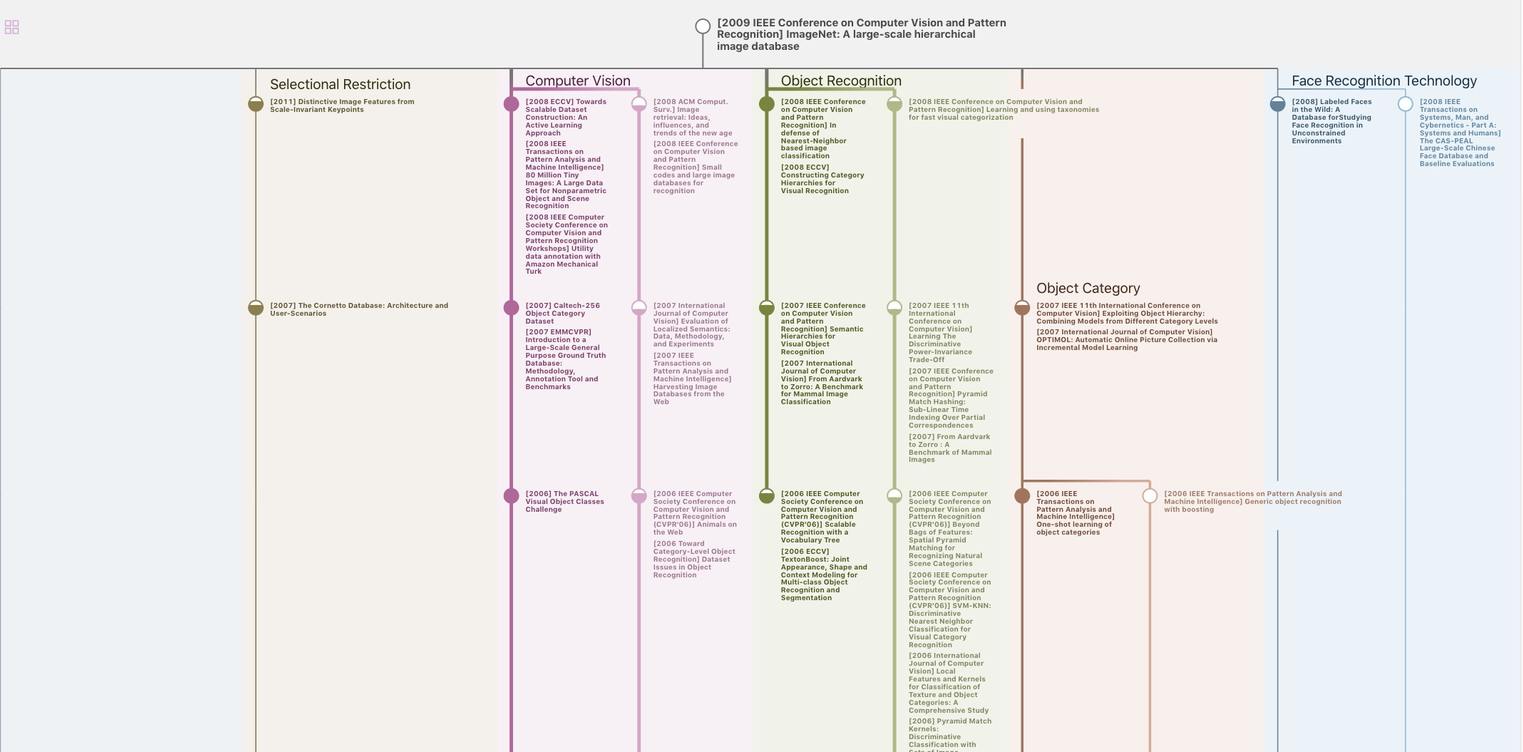
生成溯源树,研究论文发展脉络
Chat Paper
正在生成论文摘要