Deep reinforcement learning in service of air traffic controllers to resolve tactical conflicts
EXPERT SYSTEMS WITH APPLICATIONS(2024)
摘要
Dense and complex air traffic requires higher levels of automation than those exhibited by tactical conflict detection and resolution (CD & R) tools that air traffic controllers (ATCOs) use today: AI tools can act on their own initiative, increasing the capacity of ATCOs to control higher volumes of traffic. However, given that the air traffic control (ATC) domain is safety critical, requires AI systems to which ATCOs are comfortable to relinquishing control, guaranteeing operational integrity and automation adoption. Two major factors towards this goal are quality of solutions and operational transparency. ResoLver, the system that this article presents, addresses these challenges using an enhanced graph convolutional reinforcement learning method operating in a multiagent setting where each agent - representing a flight - performs a CD & R task, jointly with other agents. We show that ResoLver can provide high-quality solutions with respect to stakeholders interests (air traffic controllers and airspace users), addressing also operational transparency issues, which have been validated by ATCOs in simulated real-world settings.
更多查看译文
关键词
Air traffic control,Conflict detection and resolution,Graph convolutional reinforcement learning,Transparency
AI 理解论文
溯源树
样例
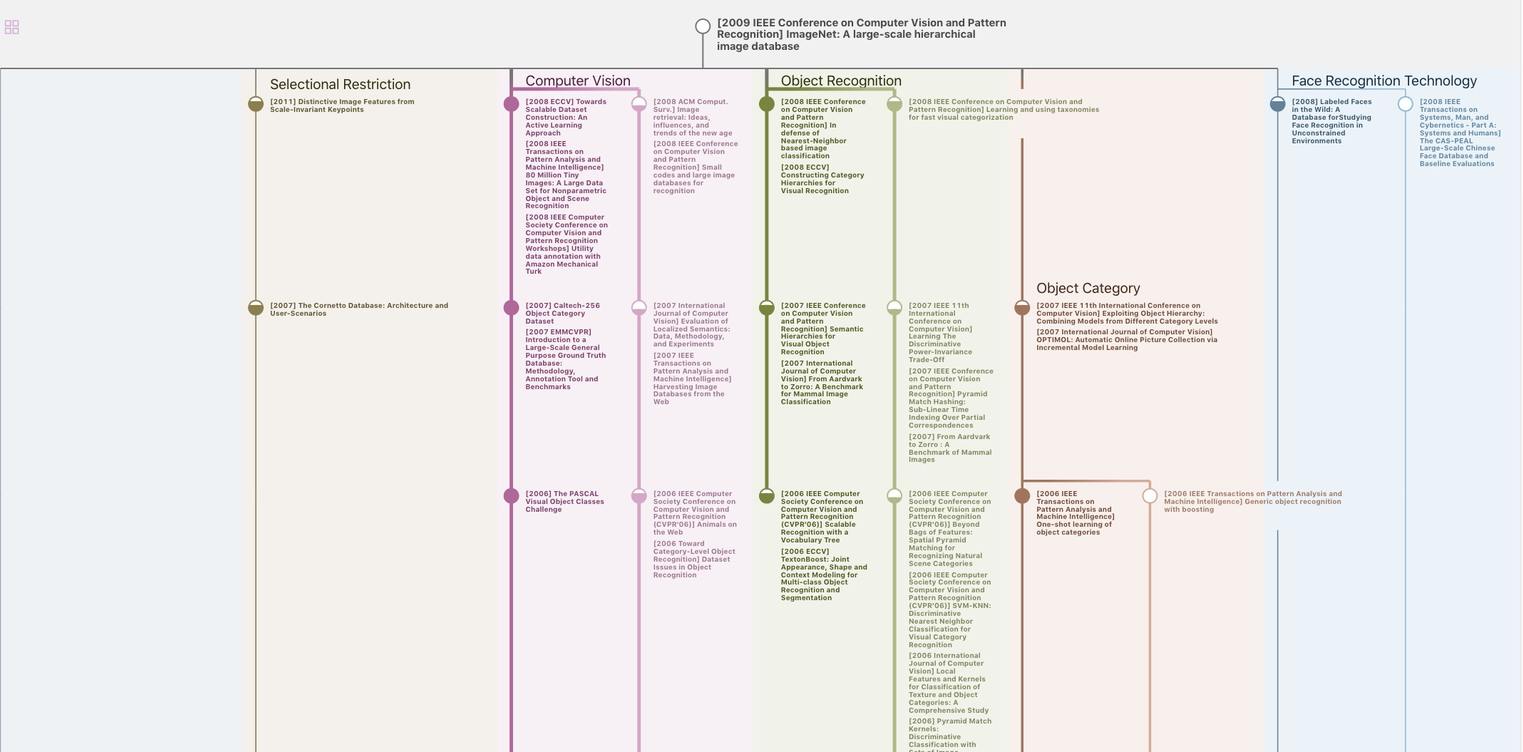
生成溯源树,研究论文发展脉络
Chat Paper
正在生成论文摘要