Deep multi-view graph clustering network with weighting mechanism and collaborative training
EXPERT SYSTEMS WITH APPLICATIONS(2024)
摘要
With the development of graph convolutional network (GCN), which is powerful in graph embedding learning meanwhile can capture node feature information, deep multi-view graph clustering methods based on graph autoencoder have emerged as a new stream. Although they achieve satisfactory performance, they still have the following weaknesses: (1) some of them model the weights for different views in the encoder part by using attention in the multi-view embedding fusion layer, but fail to consider the weighting in the decoder part to measure the contributions of different views to the reconstruction loss. (2) Most of them directly conduct clustering on the multi-view common embedding layer, but fail to guarantee the alignment of clustering results of different views. To this end, we propose a novel GCN-based deep multi-view graph clustering network with weighting mechanism and collaborative training (DMVGC). The model is composed of multiple view-specific graph encoders and a unified graph decoder. Besides the specially-designed attention module in the encoder part, we construct a reconstruction loss with adaptive weighting mechanism in the decoder part. Additionally, a collaborative self-training clustering objective is jointly conducted on each view-specific embedding layer and the common embedding layer, to make the embedding of each view clustering-friendly toward a common partition. Experiments on several datasets demonstrate the effectiveness of our model.
更多查看译文
关键词
Multi-view clustering,Graph clustering,Graph convolutional network,Graph autoencoder
AI 理解论文
溯源树
样例
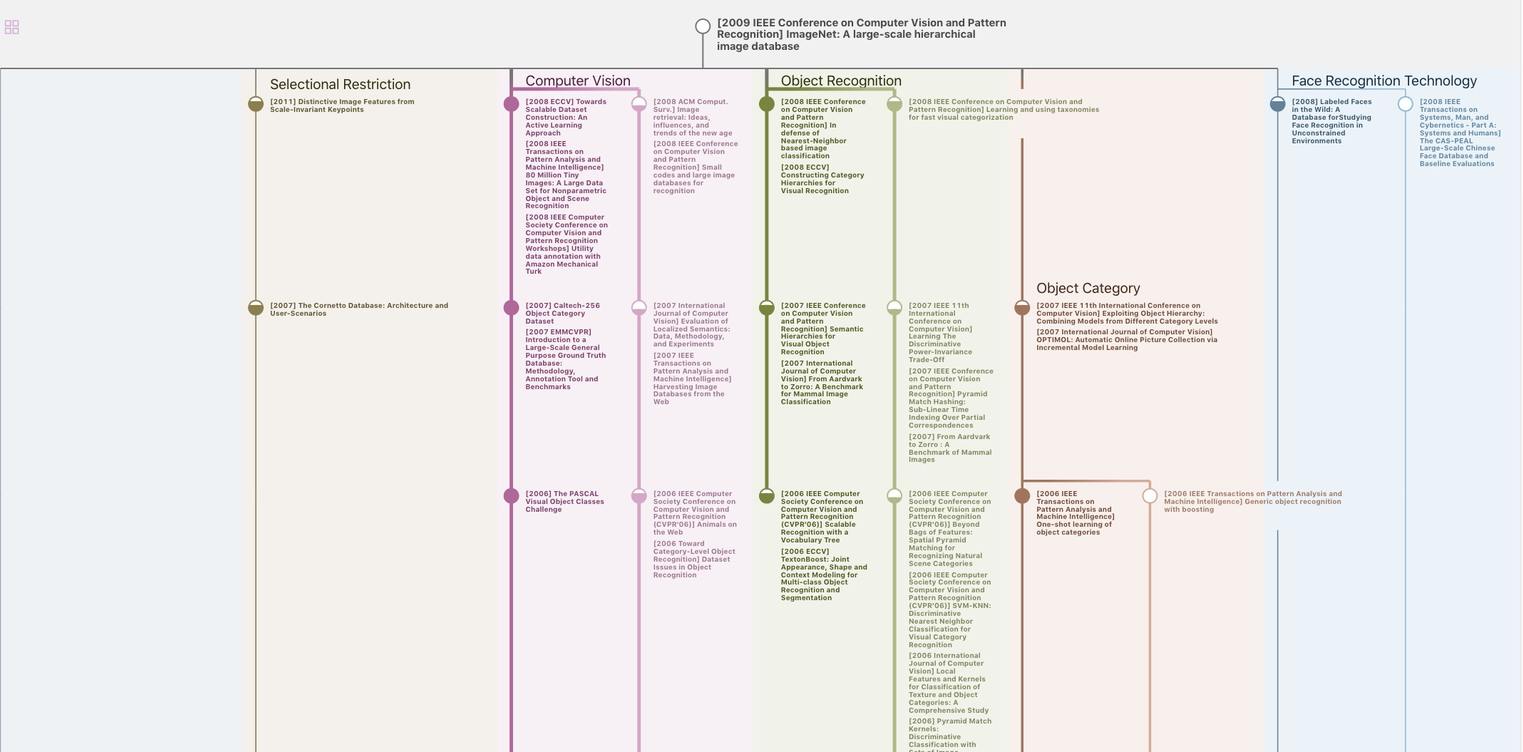
生成溯源树,研究论文发展脉络
Chat Paper
正在生成论文摘要