Forecasting tourism demand with a novel robust decomposition and ensemble framework
EXPERT SYSTEMS WITH APPLICATIONS(2024)
摘要
Current research highlights the efficacy of decomposition and ensemble algorithms in enhancing forecasting accuracy; however, the investigation of robustness associated with decomposed components within these algorithms remains notably scarce in existing literature. To address this gap, we introduce a novel tourism demand forecasting framework, underpinned by a sophisticated decomposition algorithm. Our approach initially decomposes the original data into multiple sub-series and subsequently selects forecasting models based on their respective data attributes. We evaluated the proposed framework by forecasting monthly tourist arrivals in Hong Kong from six countries. The superiority of the novel decomposition method was further substantiated through comparisons with alternative decomposition techniques in both single-step and multi-step ahead forecasting contexts. The results indicate that the proposed forecasting framework consistently outperforms baseline models in terms of forecasting accuracy across all tourism demand forecasting scenarios, with the innovative decomposition method exhibiting exceptional performance.
更多查看译文
关键词
Tourism demand forecasting,Decomposition,RobustSTL,Ensemble framework
AI 理解论文
溯源树
样例
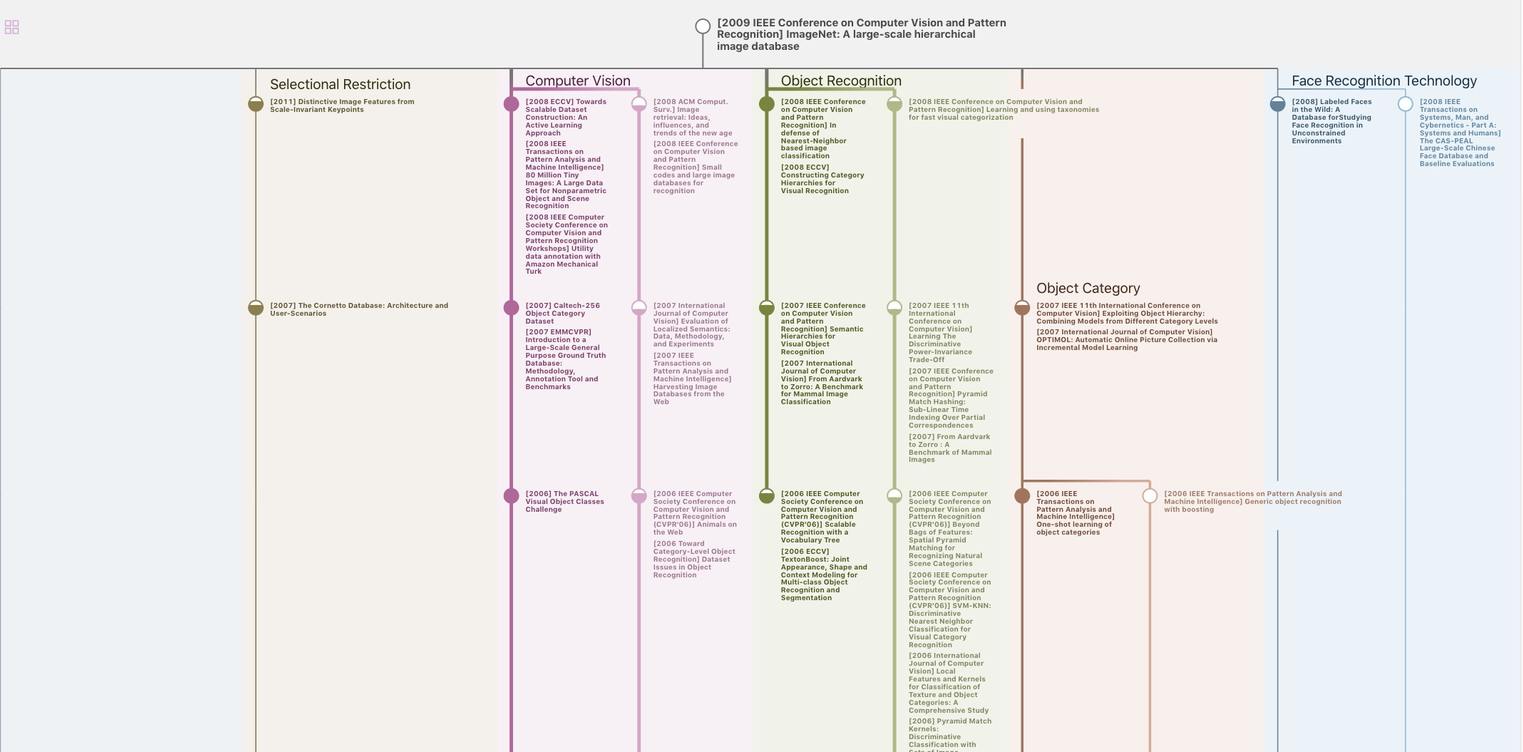
生成溯源树,研究论文发展脉络
Chat Paper
正在生成论文摘要