Sequence to sequence hybrid Bi-LSTM model for traffic speed prediction
EXPERT SYSTEMS WITH APPLICATIONS(2024)
摘要
Congestion is a bane of urban life that affects a large share of the population on a daily basis. Thus, congestion gets tremendous attention from city stakeholders, residents, and researchers. The key challenge to preventing congestion is to accurately predict the traffic status (e.g., speed) of a particular road segment which is greatly affected by many factors, such as spatial, temporal, and road conditions. Although several research studies have focused on preventing congestion, most prediction-based literature came short of accurate predictions regarding precision and time efficiency regarding large-scale datasets. This paper proposes a new hybrid approach called GRIZZLY. This approach utilizes an improved Sequence to Sequence Bi-directional Long Short Term Memory Neural Network model that integrates data pre-processing techniques such as normalization and embeddings to improve traffic prediction accuracy. Carried out experiments on two large-scale real-world datasets, namely PEMS-BAY and METR-LA, pinpointing that the proposed approach outperformed the pioneering competitors from time-series-based and hybrid neural network-based baselines in terms of the agreed-on evaluation criteria (precision and computation time).
更多查看译文
关键词
Embedding,Hybrid Bi-directional LSTM neural network,Intelligent transportation system (ITS),Normalization,Sequence to Sequence,Time-series,Traffic speed prediction
AI 理解论文
溯源树
样例
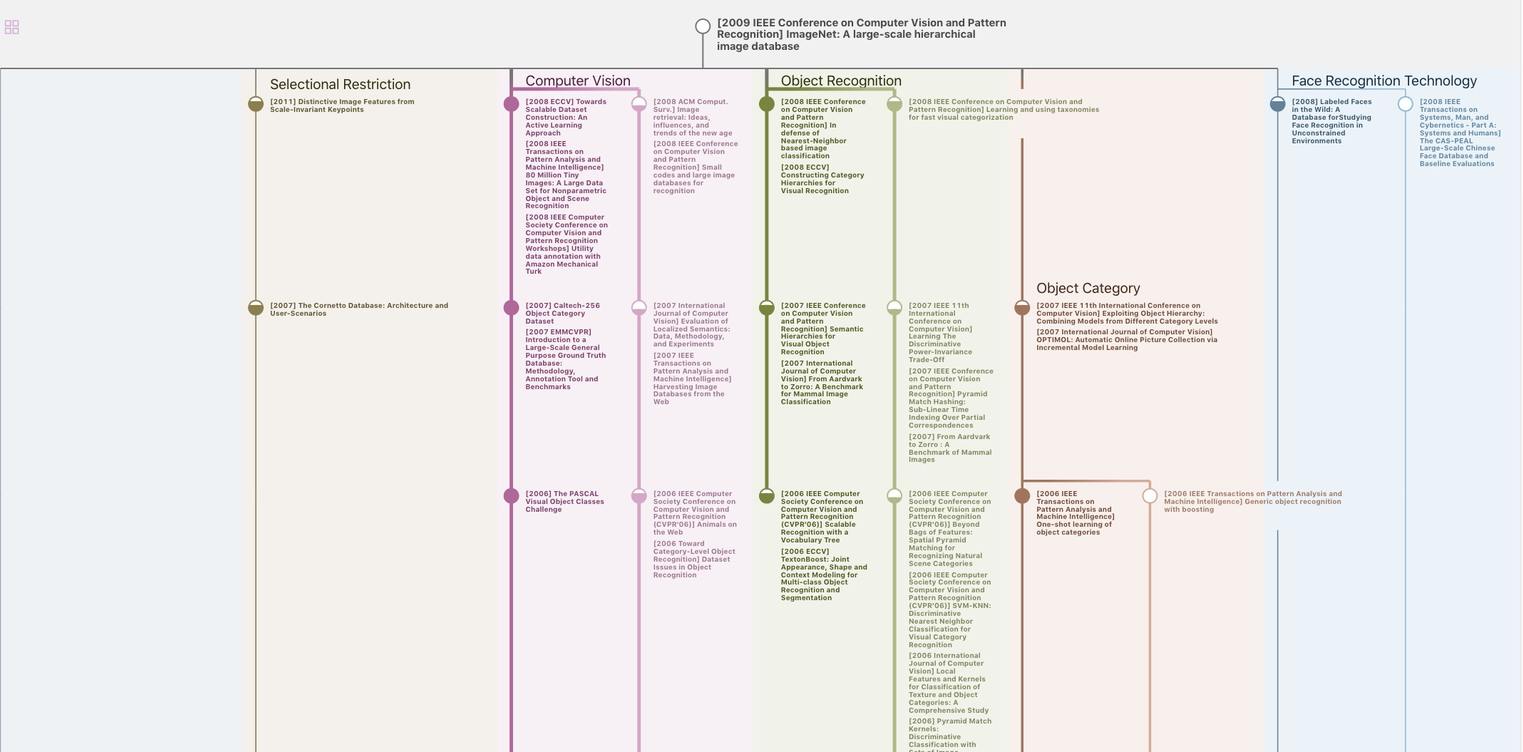
生成溯源树,研究论文发展脉络
Chat Paper
正在生成论文摘要