ECC plus plus : An algorithm family based on ensemble of classifier chains for classifying imbalanced multi-label data
EXPERT SYSTEMS WITH APPLICATIONS(2024)
摘要
Multi-label learning has attracted a great deal of research interests as it has a wide range of real-world applications. Although many multilabel learning methods have been proposed, very few of them have addressed the problem of class imbalance distribution in multilabel data. Moreover, most of the existing class imbalance multilabel learning algorithms only focus on solving the class imbalance problem, without taking into account the correlations among labels. To address these issues simultaneously, we propose to combine the well-known ensemble of classifier chain (ECC) algorithm with various binary-class imbalance learning techniques such as sampling, cost-sensitive learning, and threshold moving. This approach creates a new algorithm family called ECC++, designed specifically for class imbalance multi-label learning. ECC is already an excellent ensemble high-order binary relevance multi-label learning algorithm that is well-suited to exploiting correlations among labels. Combining it with binary-class imbalance learning techniques enables each link in a classifier chain (CC) to overcome the negative effect of skewed data distribution. ECC++ is a dynamic algorithm family that can be extended arbitrarily by applying any new binary-class imbalance learning techniques. To demonstrate the effectiveness and superiority of the proposed ECC++ algorithm family, we developed several ECC++ family members using some popular binary-class imbalance learning techniques. We then compared them with several state-of-the-art class imbalance multi-label learning algorithms on twelve benchmark and four real-world multilabel datasets. Our experimental results showed the effectiveness and superiority of the proposed ECC++ algorithm family over existing class imbalance multi-label learning algorithms. In conclusion, the proposed ECC++ algorithm family combines the strengths of the wellestablished ECC algorithm and binary-class imbalance learning techniques, resulting in a superior methodology for class imbalance multi-label learning.
更多查看译文
关键词
Multi-label learning,Class imbalance,Ensemble of classifier chains,Sampling,Cost-sensitive learning,Threshold-moving
AI 理解论文
溯源树
样例
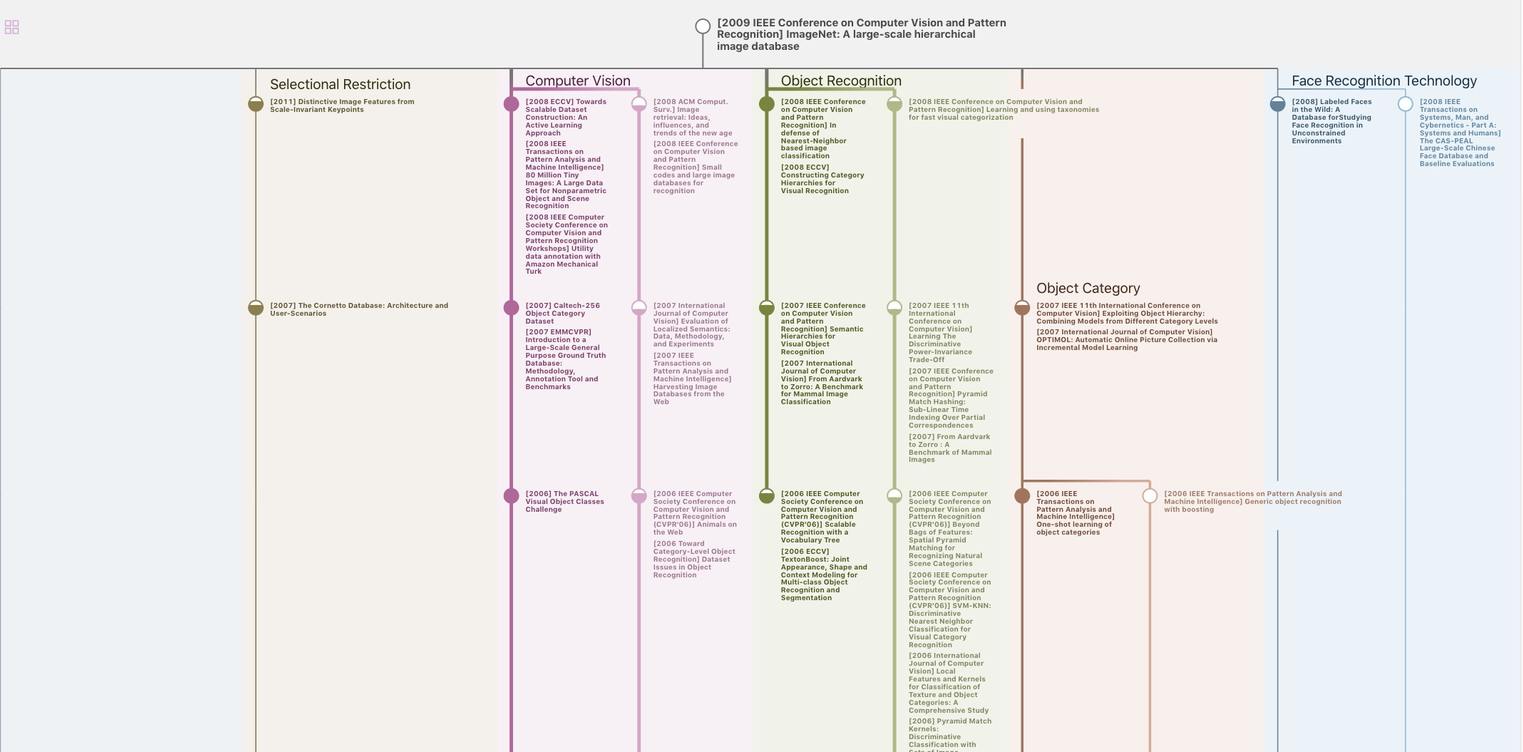
生成溯源树,研究论文发展脉络
Chat Paper
正在生成论文摘要