ISFB-GAN: Interpretable semantic face beautification with generative adversarial network
EXPERT SYSTEMS WITH APPLICATIONS(2024)
摘要
Face beautification is an emerging application widely used in the entertainment industry. Previous studies have proved that Generative Adversarial Networks (GANs) can successfully build the mapping from a general face to a target face. However, their facial style code in the latent space is taken as a black box with a lack of semantics interpretation. They usually fail to consider the geometric semantic information, and cannot focus on the facial beauty-related regions. Moreover, most of them change the background information. To overcome these problems, we propose a novel Interpretable Semantic Generative Adversarial Network (ISFB-GAN) for face beautification. First, we design a new dual-branch facial style encoder to factorize the abundant semantic information of the reference face into the facial geometry style codes and the appearance style codes in the latent space. Then, a novelty facial attention generator is proposed to preserve more background information in the generated images. In the attention generator, the facial style attention is designed to focus on the most discriminative facial region; the background attention is designed to retain the background information. Finally, we propose a hybrid loss function including geometric loss, orthogonality loss, segmentation loss, style reconstruction loss, perceptual loss, etc., to enhance the learning capacity and improve the visual quality. The combination of the geometric loss and the orthogonality loss is deployed to guarantee the disentanglement between the semantic information. Extensive experiments conducted on the CelebAMask-HQ dataset and the SCUT-FBP5500 dataset, demonstrate that ISFB-GAN performs better than the state-of-the-art methods in facial attractiveness enhancement.
更多查看译文
关键词
Face beautification,Generative adversarial network,Style code,Interpretable semantics
AI 理解论文
溯源树
样例
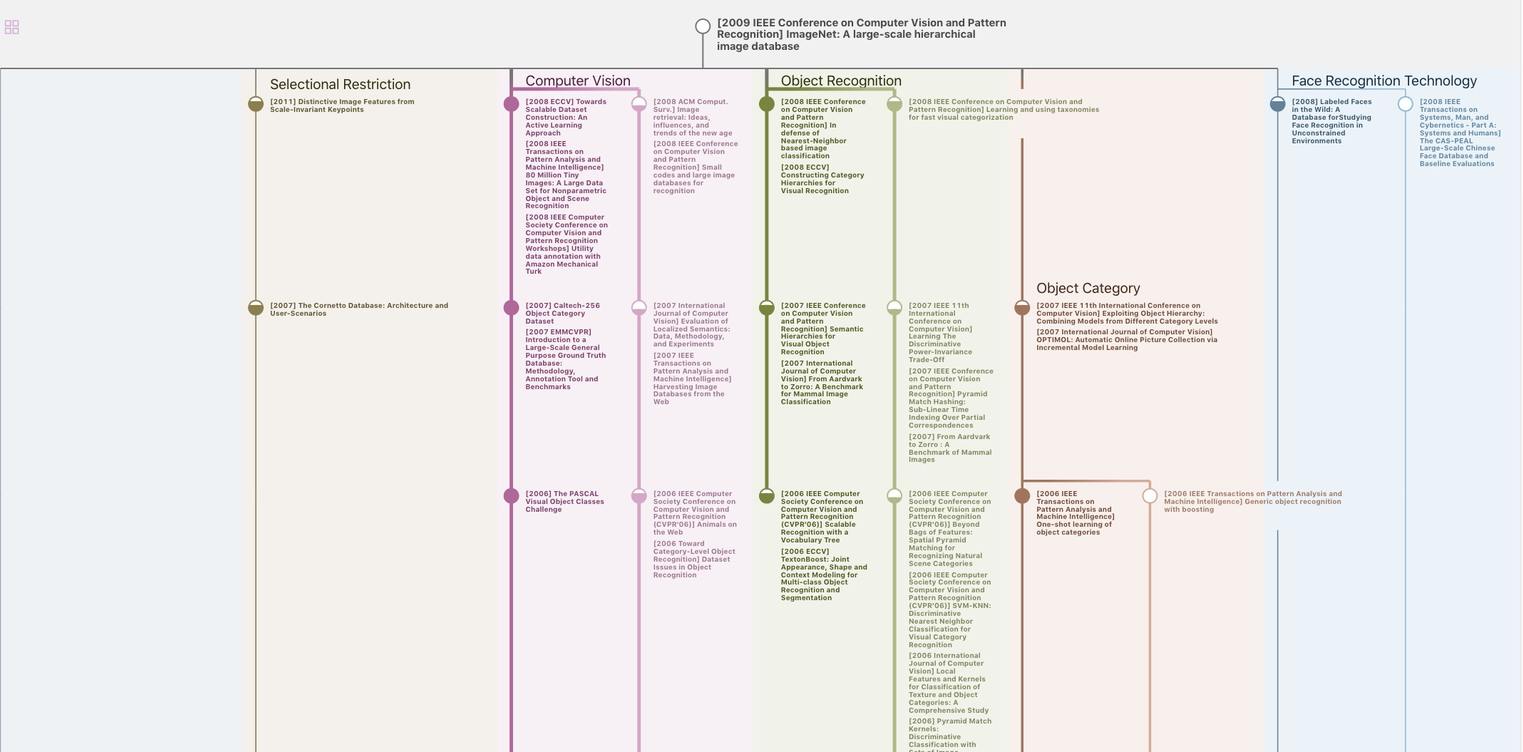
生成溯源树,研究论文发展脉络
Chat Paper
正在生成论文摘要