Predicting In-Vitro Fertilization Outcome from Ultrasound Measurements Using Machine Learning Techniques
2023 Seventh International Conference on Advances in Biomedical Engineering (ICABME)(2023)
摘要
Predicting the implantation outcomes of in vitro fertilization (IVF) is important to the treatment's success. Therefore, machine-learning techniques including Support Vector Machines (SVM), Gradient Boosting (GB), Extreme Gradient Boosting (XGB), and Random Forest (RF) were tested for their ability to predict IVF pregnancy success using a combination of Doppler and clinical parameters. Forecasting ability was evaluated utilizing widely known performance indicators such as accuracy rate, sensitivity, specificity, and AUC. The GB combined with Random Forest Importance Feature selection technique showed the highest performance with a sensitivity of 100
%
, a specificity of 66%, and an accuracy of 82.3% compared to other techniques that tried to predict the outcome of IVF and Intracytoplasmic sperm injection (ICSI) using machine learning techniques. Ultrasound measurement parameters and especially Doppler parameters are an important factor that affects the outcome of IVF.
更多查看译文
关键词
In Vitro Fertilization (IVF),Intra Cytoplasmic Sperm Injection (ICSI),Machine Learning,Gradient Boosting (GB),Support Vector Machine (SVM),Random Forest (RF),Extreme Gradient Boosting (XGB),Doppler parameters
AI 理解论文
溯源树
样例
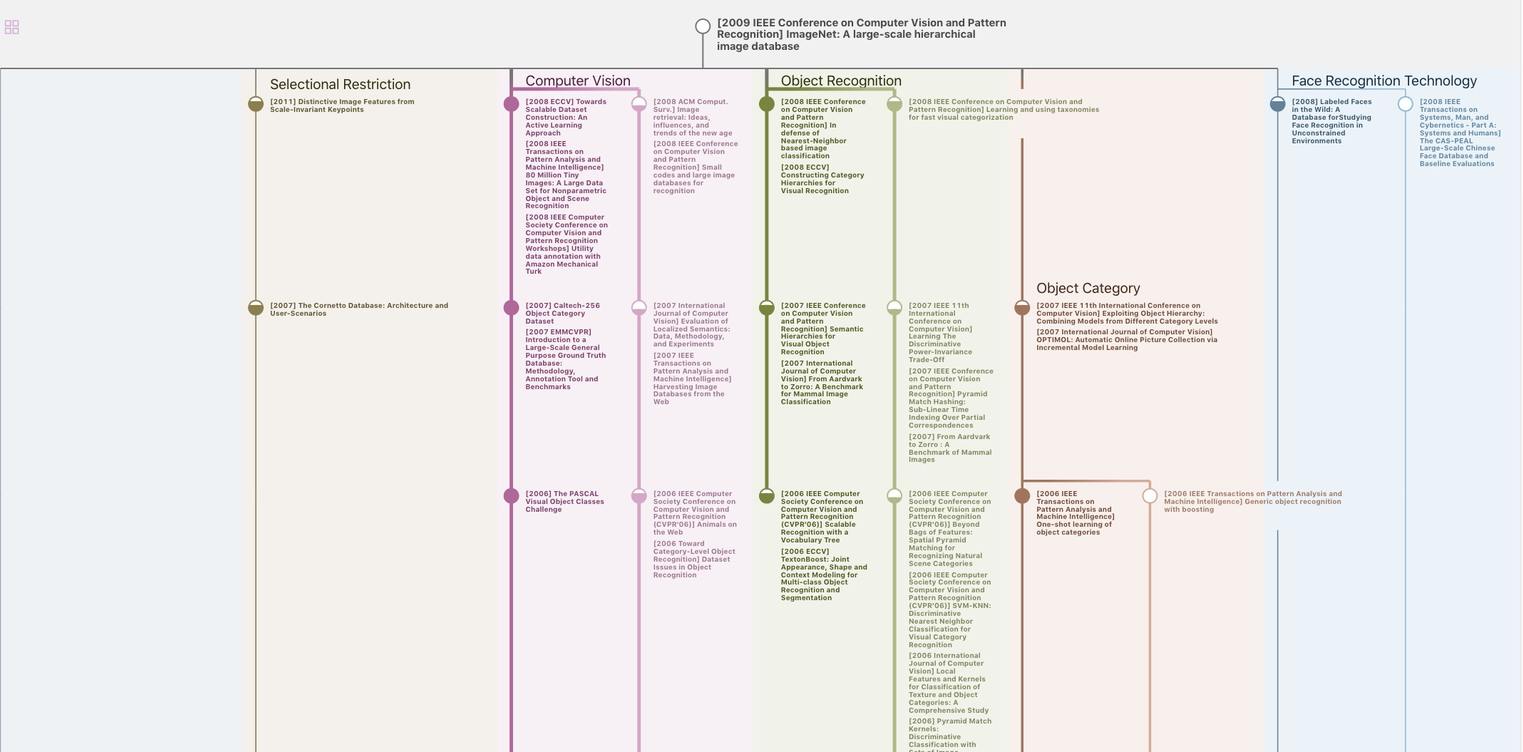
生成溯源树,研究论文发展脉络
Chat Paper
正在生成论文摘要