Dimensionality Reduction on IoT Monitoring Data of Smart Building for Energy Consumption Forecasting
2023 IEEE International Smart Cities Conference (ISC2)(2023)
摘要
The Internet of Things (IoT) plays a major role today in smart building infrastructures, from simple smart-home applications, to more sophisticated industrial type installations. The vast amounts of data generated from relevant systems can be processed in different ways revealing important information. This is especially true in the era of edge computing, when advanced data analysis and decision-making is gradually moving to the edge of the network where devices are generally characterised by low computing resources. In this context, one of the emerging main challenges is related to maintaining data analysis accuracy even with less data that can be efficiently handled by low resource devices. The present work focuses on correlation analysis of data retrieved from a pilot IoT network installation monitoring a small smart office by means of environmental and energy consumption sensors. The research motivation was to find statistical correlation between the monitoring variables that will allow the use of machine learning (ML) prediction algorithms for energy consumption reducing input parameters. For this to happen, a series of hypothesis tests for the correlation of three different environmental variables with the energy consumption were carried out. A total of ninety tests were performed, thirty for each pair of variables. In these tests, p-values showed the existence of strong or semi-strong correlation with two environmental variables, and of a weak correlation with a third one. Using the proposed methodology, we manage without examining the entire data set to exclude weak correlated variables while keeping the same score of accuracy.
更多查看译文
关键词
Internet of Things,Data Analysis,Correlation,Hypothesis Testing,Energy Consumption Prediction
AI 理解论文
溯源树
样例
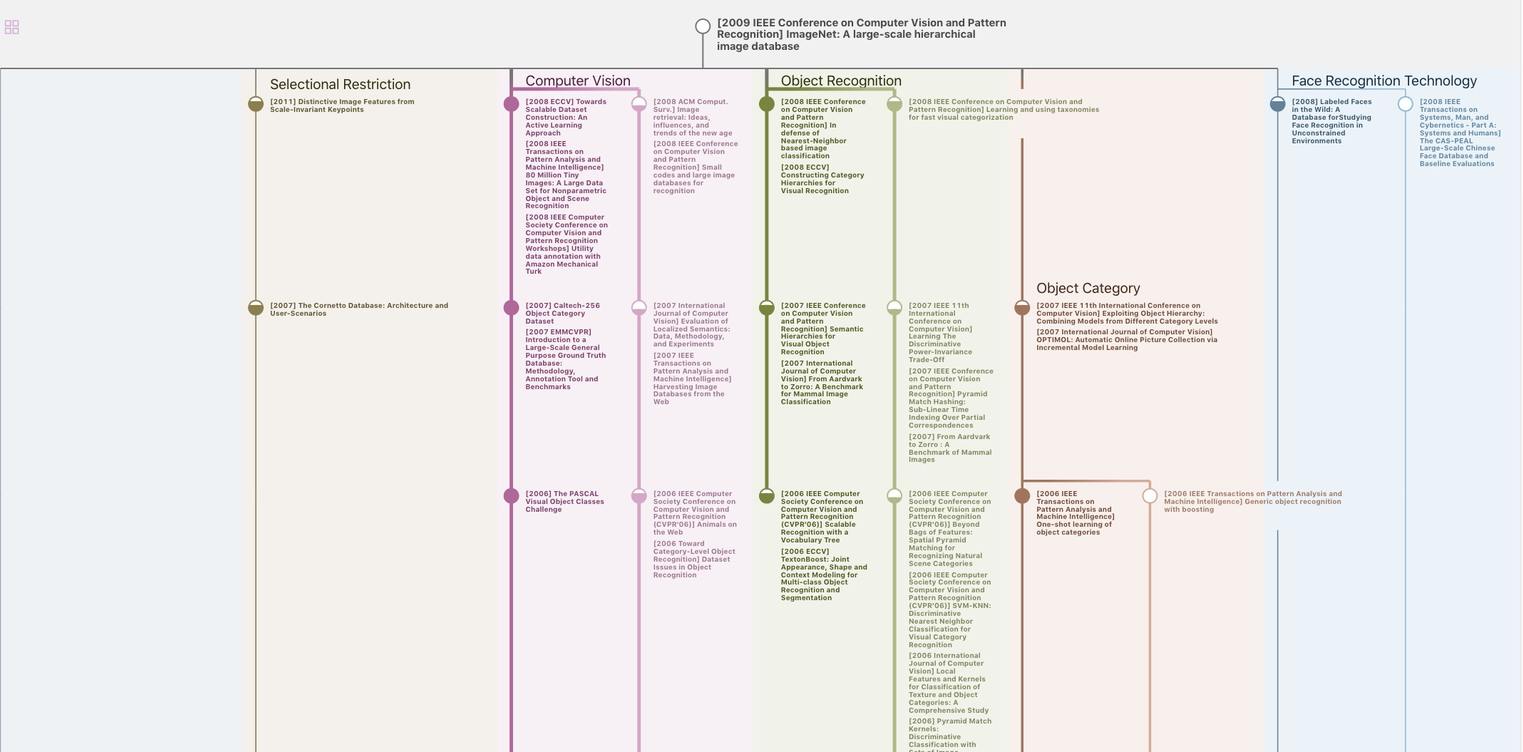
生成溯源树,研究论文发展脉络
Chat Paper
正在生成论文摘要