An Evaluation of Conditional Random Fields in Predicting Out-of-Home Activities
2023 IEEE International Smart Cities Conference (ISC2)(2023)
摘要
In urban planning, information of travel-activity demands can be used to optimize mobility services and land use patterns. To support this practice, this study evaluated the performance of Conditional Random Fields (CRFs) in predicting out-of-home activities. The performance of CRFs was also compared with those of Naive Bayes classifier, Logistic classifier, and Hidden Markov Model classifier. Data of 13,000 respondents in a survey in Japan were used for evaluations. Using the weekday dataset, the CRFs Model 3 yielded the highest F1 score of 52.91% and Accuracy of 59.91% in the second test time, and on average of five test times its performance by two criteria were 23.1% and 13.05% higher than the second-best classifiers. Using the weekend dataset with higher class-imbalance level, all classifiers performed quite similarly. Further, the performance of CRFs decreased as the sequence length increased. Overall, CRFs can be a good predictor for out-of-home activities but this should be taken with noting that its performance may vary greatly depending on various factors, including the number of response-specific variables, the activity sequence lengths, and the imbalance level of activity types.
更多查看译文
关键词
Conditional Random Fields,travel,activities
AI 理解论文
溯源树
样例
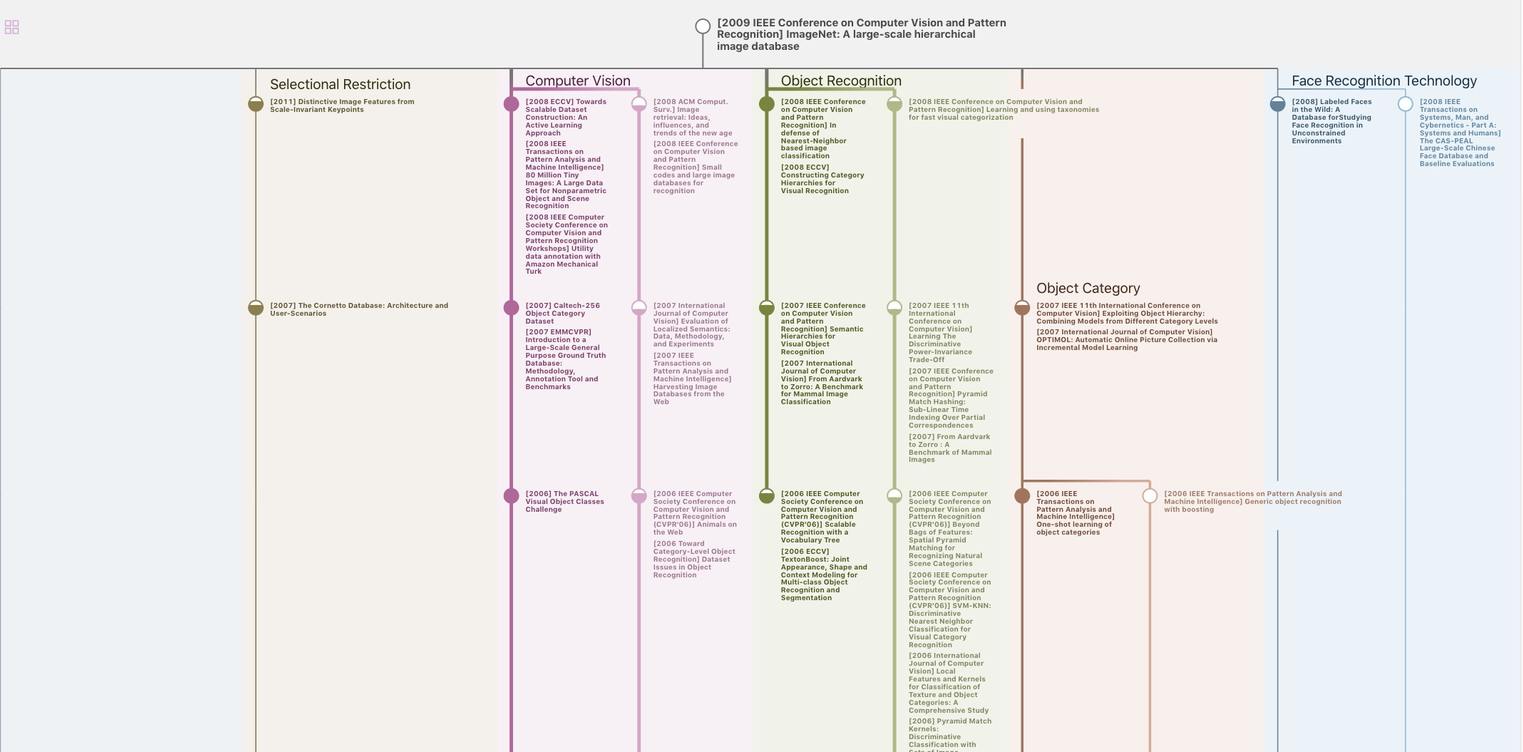
生成溯源树,研究论文发展脉络
Chat Paper
正在生成论文摘要