A Data-Driven LSTM Soft Sensor Model Based on Bayesian Optimization for Hydraulic Pressure Measurement of Excavator
IEEE SENSORS JOURNAL(2023)
摘要
With the development of hardware and software, the pressure control of construction machinery embraced new characteristics of sensors' failure. In recent years, soft sensors have been widely used in construction machinery for fault-tolerant controlling. To cope with the failure of some pressure sensors which cause great harm to the electro-hydraulic proportional control of excavators and accurately measure hydraulic pressure without actual pressure sensors, a pressure soft sensor based on long short-term memory (LSTM) neural network is proposed in this work. And Bayesian optimization (BO) is used to tune the hyperparameters of LSTM. The hydraulic system of an SY375IDS excavator is analyzed, and the mutual information (MI) strategy is adopted to determine features that are given to LSTM. In the case of multiple sensors' failure at the same time, a multiple-input multiple-output (MIMO) prediction mechanism based on BO-LSTM and an MIMO cyclic prediction mechanism based on two multiple-input single-output (MISO) models are proposed. Finally, the performance of the proposed pressure soft sensor is evaluated in the compared experiments with back propagation neural network (BPNN), convolutional neural network (CNN), and recurrent neural network (RNN). The results show that the proposed method is superior to other methods in terms of pressure prediction.
更多查看译文
关键词
Bayesian optimization (BO),hydraulic pressure,long short-term memory (LSTM),soft sensor
AI 理解论文
溯源树
样例
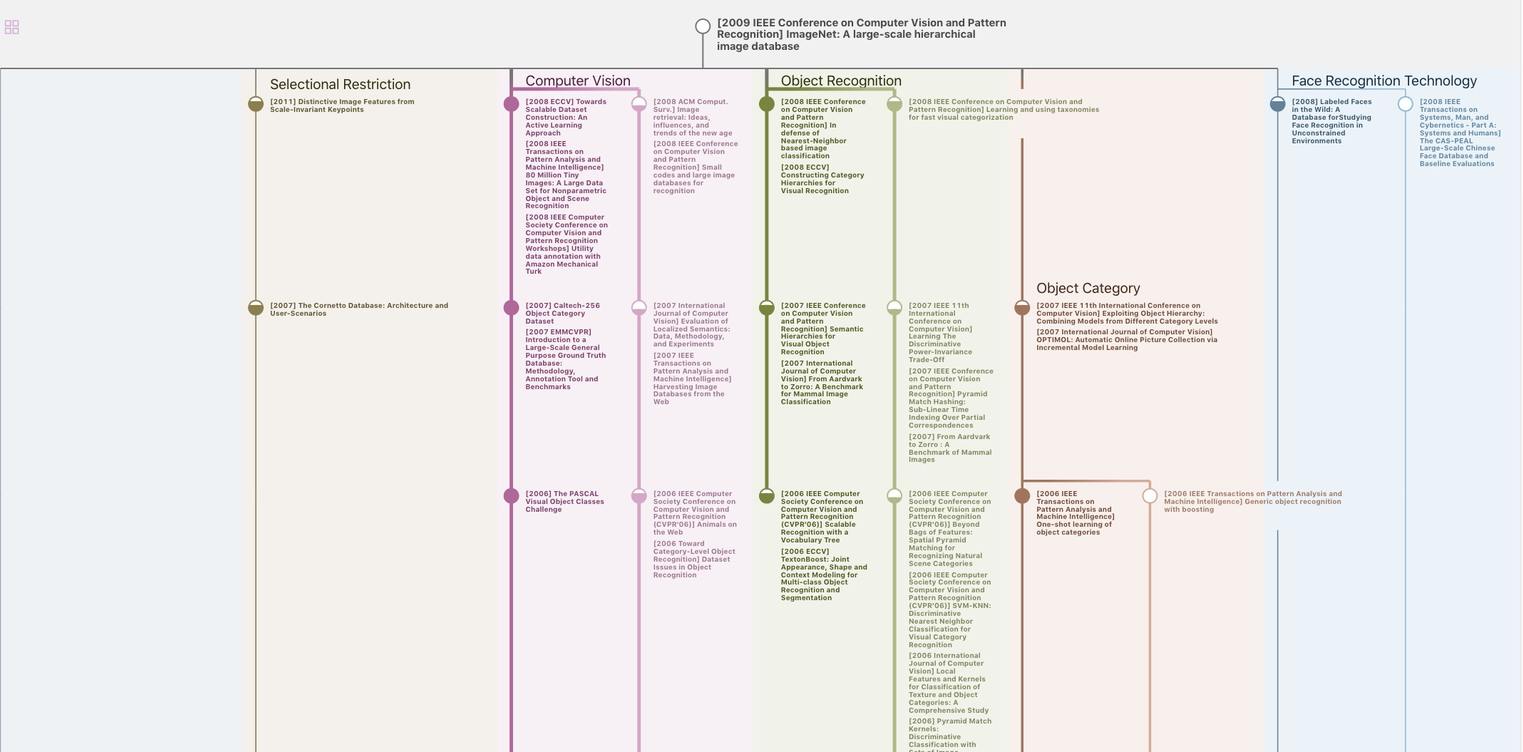
生成溯源树,研究论文发展脉络
Chat Paper
正在生成论文摘要