Data-driven rational design of single-atom materials for hydrogen evolution and sensing
Nano Research(2024)
摘要
Herein we proposed a data-driven high-throughput principle to screen high-performance single-atom materials for hydrogen evolution reaction (HER) and hydrogen sensing by combing the theoretical computations and a topology-based multi-scale convolution kernel machine learning algorithm. After the rational training by 25 groups of data and prediction of all 168 groups of single-atom materials for HER and sensing, respectively, a high prediction accuracy (> 0.931 R 2 score) was achieved by our model. Results show that the promising HER catalysts include Pt atoms in C 4 and Sc atoms in C 1 N 3 coordination environment. Moreover, Y atoms in C 4 coordination environment and Cd atoms in C 2 N 2 -ortho coordination environment were predicted with great potential as hydrogen sensing materials. This method provides a way to accelerate the discovery of innovative materials by avoiding the time-consuming empirical principles in experiments.
更多查看译文
关键词
machine learning,single-atom materials,sensing,hydrogen evolution
AI 理解论文
溯源树
样例
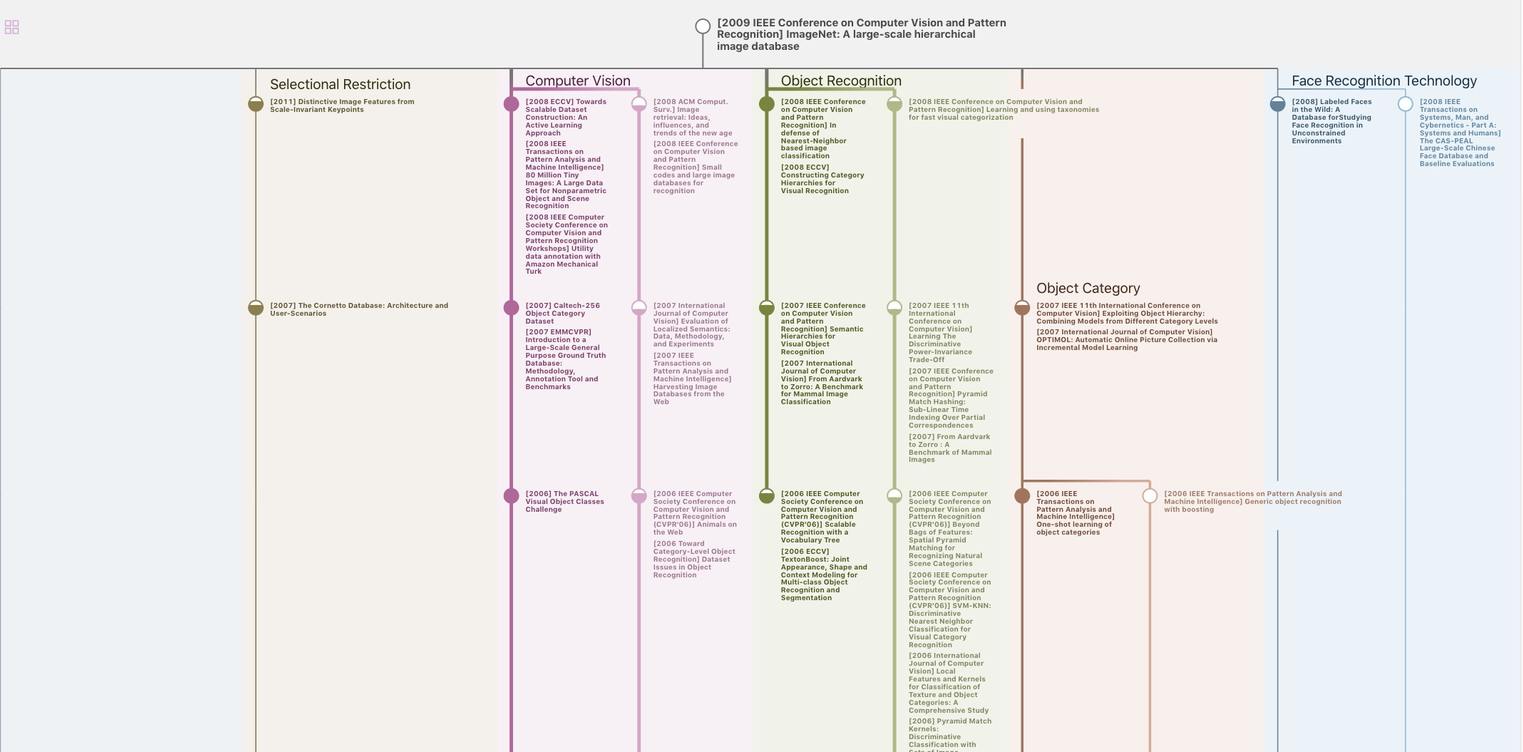
生成溯源树,研究论文发展脉络
Chat Paper
正在生成论文摘要