Estimation of extreme quantiles from heavy-tailed distributions with neural networks
Statistics and Computing(2023)
摘要
We propose new parametrizations for neural networks in order to estimate extreme quantiles in both non-conditional and conditional heavy-tailed settings. All proposed neural network estimators feature a bias correction based on an extension of the usual second-order condition to an arbitrary order. The convergence rate of the uniform error between extreme log-quantiles and their neural network approximation is established. The finite sample performances of the non-conditional neural network estimator are compared to other bias-reduced extreme-value competitors on simulated data. It is shown that our method outperforms them in difficult heavy-tailed situations where other estimators almost all fail. Finally, the conditional neural network estimators are implemented to investigate the behavior of extreme rainfalls as functions of their geographical location in the southern part of France. The source code is available at https://github.com/michael-allouche/nn-quantile-extrapolation.git .
更多查看译文
关键词
Extreme-value theory,Heavy-tailed distribution,Quantile estimation,Conditional quantile estimation,Neural networks
AI 理解论文
溯源树
样例
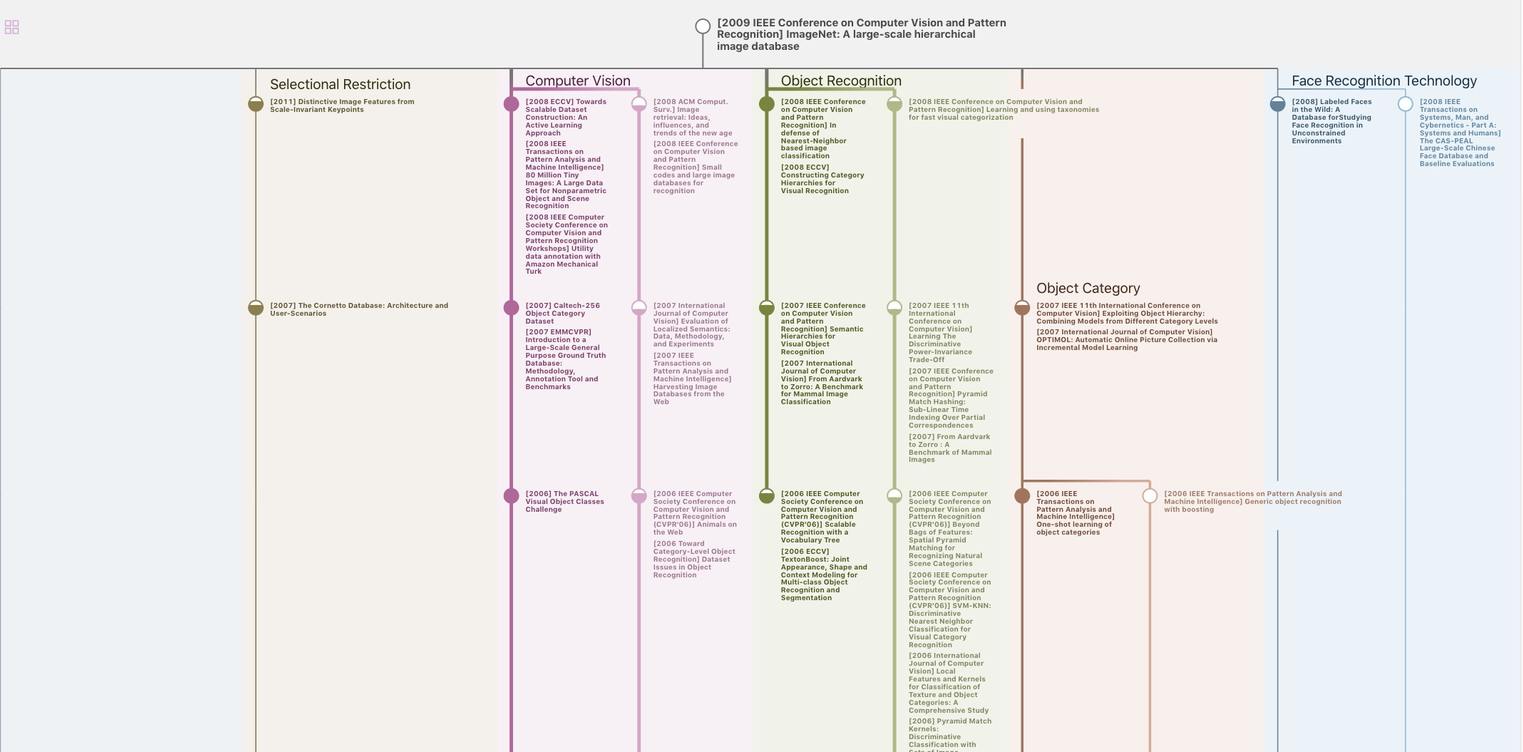
生成溯源树,研究论文发展脉络
Chat Paper
正在生成论文摘要